Multirate-Former: An Efficient Transformer-Based Hierarchical Network for Multistep Prediction of Multirate Industrial Processes
IEEE TRANSACTIONS ON INSTRUMENTATION AND MEASUREMENT(2024)
摘要
Due to the limitations of measurement technology and cost in industrial processes, it is difficult to obtain measured values of variables with different properties, such as flow rate and temperature, under uniform sampling rates. This leads to the ubiquitous multirate sampling characteristics of the collected industrial process data, which brings great challenges to the quality prediction of industrial processes. To address this issue, this article proposes a novel quality prediction modeling method based on the transformer modal called Multirate-Former for multirate industrial processes. First, the raw data are chunked by arranging data variables to the same sampling rate. Then, the hierarchical coarse-grained and fine-grained complementation of the data are completed by the multilayer convolution network and transformer. Notably, a novel sampling-type coding method is proposed to explore the missing pattern of multirate process data. After the above pretraining, the patched completed dataset and better initial weights are provided for the subsequent fine-tuning process. Finally, the multistep prediction error of quality variables is exploited to fine-tune the entire network parameters. The proposed method is applied to an industrial debutanizer column and an actual industrial hydrocracking process for multirate multistep prediction. Experimental results demonstrate that the proposed method outperforms other state-of-the-art methods in dealing with multisampling rate types of industrial process data.
更多查看译文
关键词
Multirate industrial processes,Multirate-Former,multistep prediction,sampling-type coding
AI 理解论文
溯源树
样例
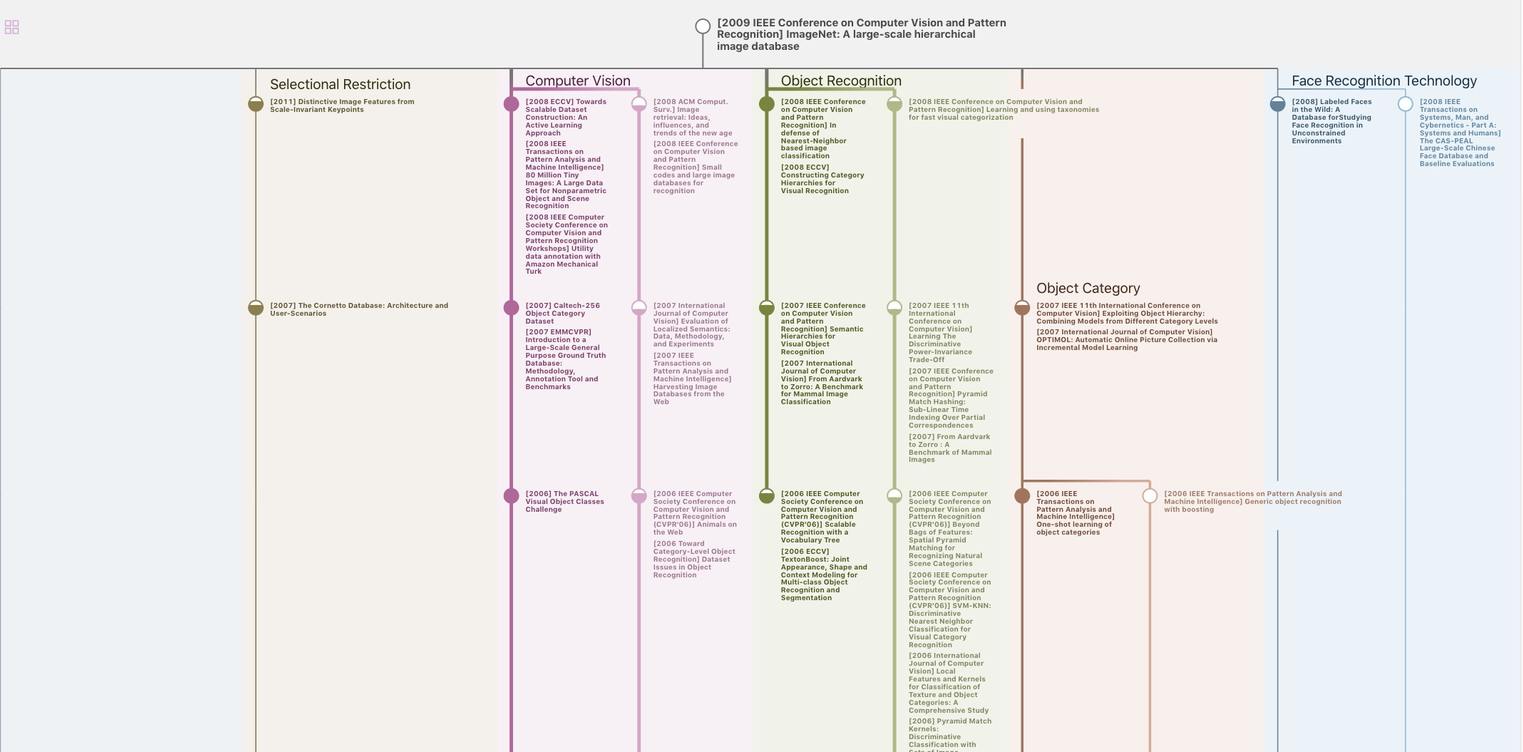
生成溯源树,研究论文发展脉络
Chat Paper
正在生成论文摘要