Towards Fully Automated Machine Learning for Routability Estimator Development
IEEE Transactions on Computer-Aided Design of Integrated Circuits and Systems(2023)
摘要
The rise of machine learning technology inspires a boom of its applications in electronic design automation (EDA) and helps improve the degree of automation in chip designs. However, manually crafting machine learning models remains a complex and time-consuming process because it requires extensive human expertise and tremendous engineering efforts to carefully extract features and design model architectures. In this work, we leverage automated machine learning techniques to automate the machine learning (ML) model development for routability prediction, a well-established technique that can help to guide cell placement toward routable solutions. We present an automated feature selection method to identify suitable features for model inputs.We develop a neural architecture search method to search for high-quality neural architectures without human interference. Our search method supports various operations and highly flexible connections, leading to architectures significantly different from all previous human-crafted models. Our experimental results demonstrate that our automatically generated models clearly outperform multiple representative manually crafted solutions with a superior 9.9% improvement. Moreover, compared with human-crafted models, which easily take weeks or months to develop, our efficient AutoML framework completes the whole model development process with only 1 day.
更多查看译文
关键词
routability estimator development,machine learning
AI 理解论文
溯源树
样例
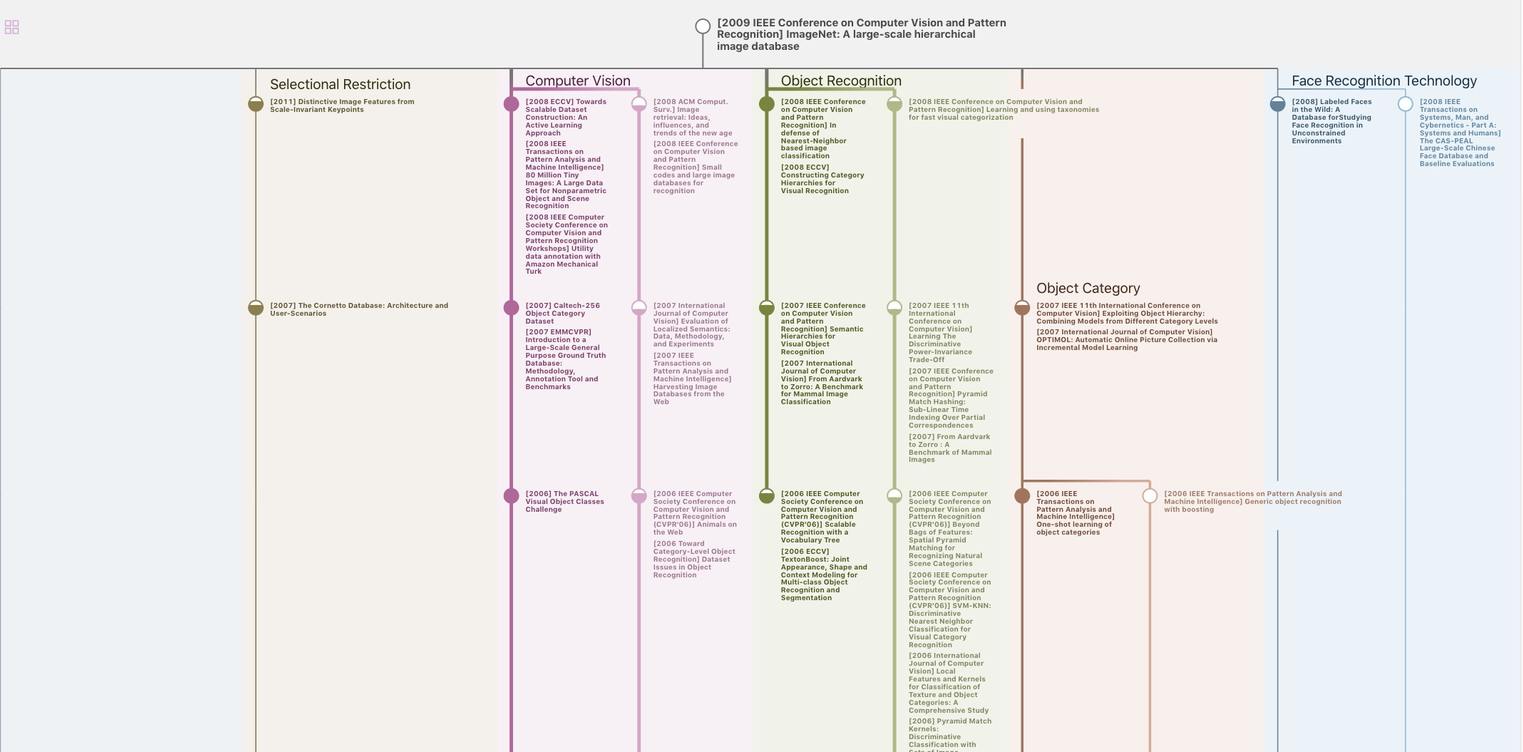
生成溯源树,研究论文发展脉络
Chat Paper
正在生成论文摘要