An Efficient Adaptive Multi-Kernel Learning With Safe Screening Rule for Outlier Detection
IEEE Transactions on Knowledge and Data Engineering(2023)
摘要
Recent advances in multi-kernel-based methods for outlier detection have positioned them as an attractive way to detect instances that are markedly different from the remaining data in a dataset. Currently, most outlier detection approaches based on multi-kernel learning are simply a convex combination of various kernels with handcrafted weights, meaning that these weights may not be suitable. Meanwhile, this combination of weights does not sufficiently consider the intrinsic correlations of instances when fusing different kernels. Thus, a key challenge is how to adaptively learn an appropriate combination of weights for capturing a new feature space in which outliers can be better detected than the original space. Simultaneously, it is still a burning issue to get the optimal combination of weights due to considerable computational cost and memory usage when the feature or instance size is large. In this paper, we propose a novel method for
e
fficient
a
daptive
m
ulti-kernel for
o
utlier
d
etection (EAMOD), which automatically learns the optimal weight for each training instance under different kernels using a non-negative function. In addition, we design a safe screening rule (SSR) for EAMOD to improve its training efficiency without any loss of accuracy. To the best of our knowledge, it is the first attempt to develop SSR for multi-kernel-based outlier detection methods. Extensive experiments show that EAMOD is effective and efficient.
更多查看译文
关键词
Multi-kernel learning,outlier detection,safe screening rule (SSR),support vector data description (SVDD)
AI 理解论文
溯源树
样例
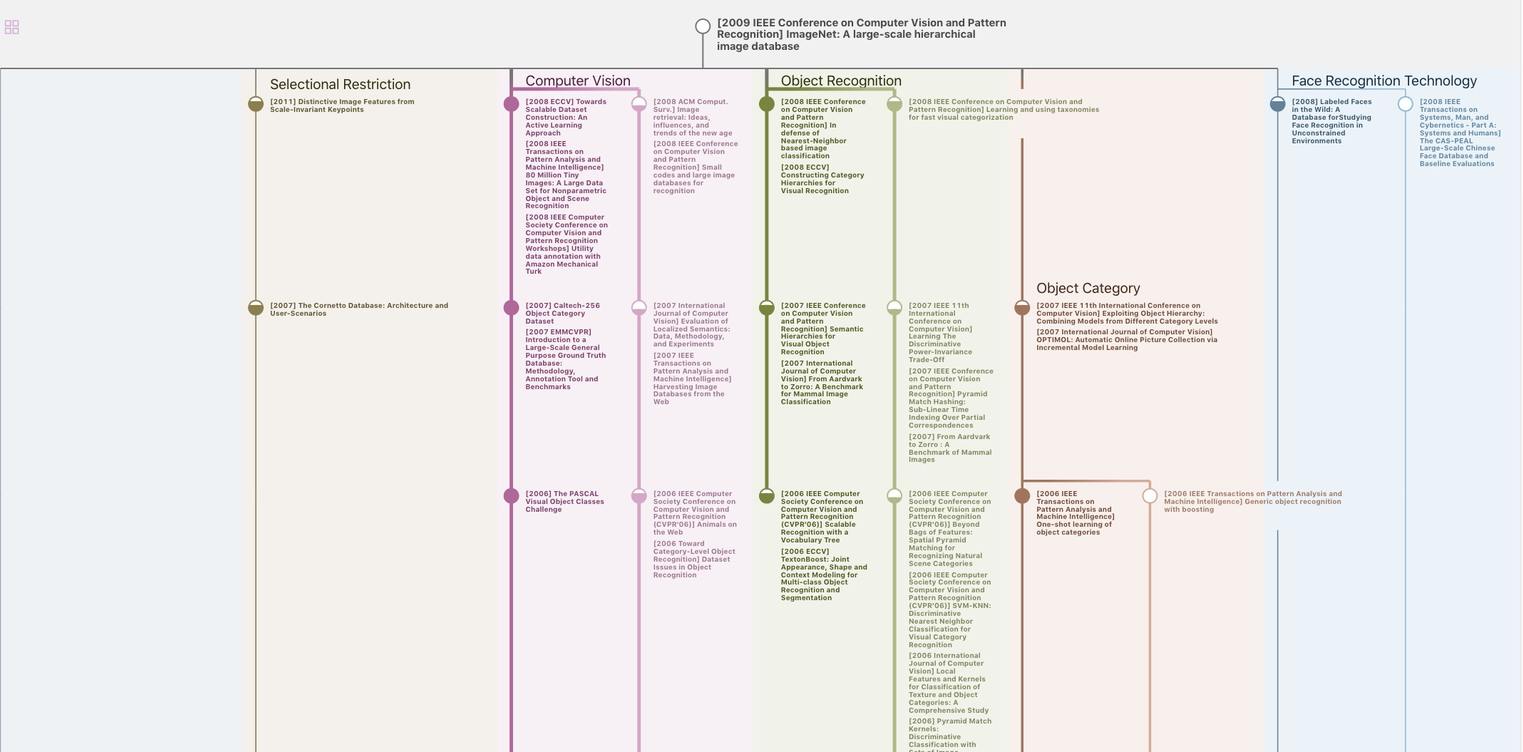
生成溯源树,研究论文发展脉络
Chat Paper
正在生成论文摘要