Universal Multi-modal Multi-domain Pre-trained Recommendation
arXiv (Cornell University)(2023)
摘要
There is a rapidly-growing research interest in modeling user preferences via pre-training multi-domain interactions for recommender systems. However, Existing pre-trained multi-domain recommendations mostly select the item texts to be bridges across domains, and simply explore the user behaviors in target domains. Hence, they ignore other informative multi-modal item contents (e.g., visual information), and also lack of thorough consideration of user behaviors from all interactive domains. To address these issues, in this paper, we propose to pre-train universal multi-modal item content presentation for multi-domain recommendation, called UniM^2Rec, which could smoothly learn the multi-modal item content presentations and the multi-modal user preferences from all domains. With the pre-trained multi-domain recommendation model, UniM^2Rec could be efficiently and effectively transferred to new target domains in practice. Extensive experiments conducted on five real-world datasets in target domains demonstrate the superiority of the proposed method over existing competitive methods, especially for the real-world recommendation scenarios that usually struggle with seriously missing or noisy item contents.
更多查看译文
关键词
recommendation,multi-modal,multi-domain,pre-trained
AI 理解论文
溯源树
样例
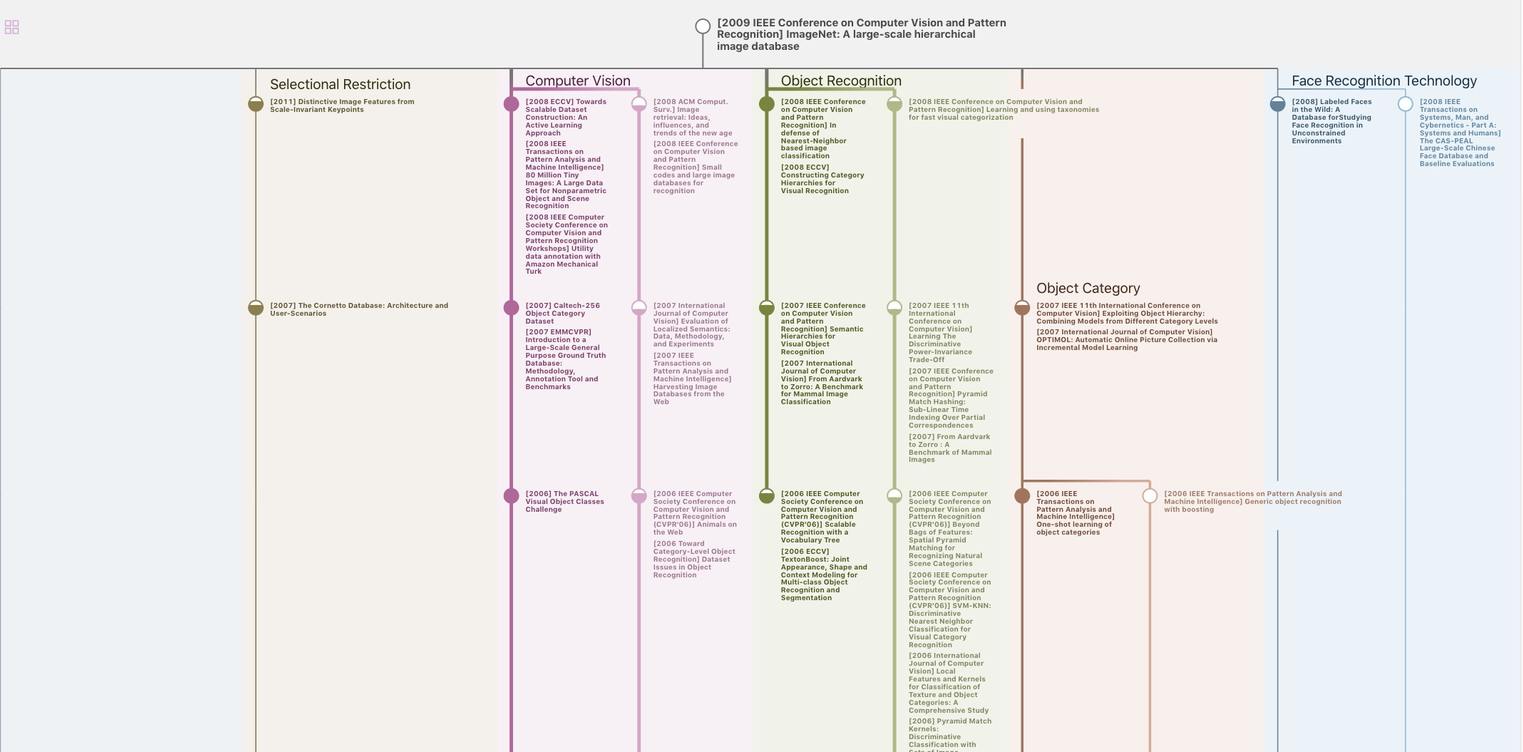
生成溯源树,研究论文发展脉络
Chat Paper
正在生成论文摘要