Mountain Gazelle Optimizer with Deep Learning Driven Satirical News Classification on Low-resource Language Corpus
ACM Transactions on Asian and Low-Resource Language Information Processing(2023)
摘要
The development of satirical and fake news on digital platforms has source of major concern about the spread of misinformation and its control on society. As part of the Arabic language, fake news detection (FND) shows particular problems because of language difficulties and the scarcity of labeled data. FND on Arabic corpus utilizing deep learning (DL) contains leveraging advanced neural network (NN) techniques and methods to automatically recognize and classify deceptive data in the Arabic language text. This procedure is vital in combating the spread of disinformation and misinformation, promoting media literacy, and make sure the credibility of data sources for the Arabic-speaking community. Recurrent Neural Networks (RNNs) and Convolutional Neural Networks (CNNs) are common selections for FND because of their capability for learning hierarchical features and model sequential data from the text. In this view, this study develops a Mountain Gazelle Optimizer with Deep Learning-Driven Fake News Classification on Arabic Corpus (MGODL-FNCAC) technique. The presented MGODL-FNCAC approach aims to increase the performance of the fake news classification on the Arabic corpus. Primarily, the MGODL-FNCAC technique involves different stages of pre-processing to make the input data compatible for classification. For fake news detection, the MGODL-FNCAC technique applies the deep belief network (DBN) model. At last, the MGO approach can be used for the better hyperparameter tuning of the DBN approach, which supports in enhancing the overall training process and detection rate. The simulation outcomes of the MGODL-FNCAC technique can be examined on Arabic corpus data. The extensive outcomes exhibit the importance of the MGODL-FNCAC system over other methodologies with maximum accuracy of 97.68% and 95.14% on Covid19Fakes and Satirical dataset, respectively.
更多查看译文
关键词
deep learning,low-resource
AI 理解论文
溯源树
样例
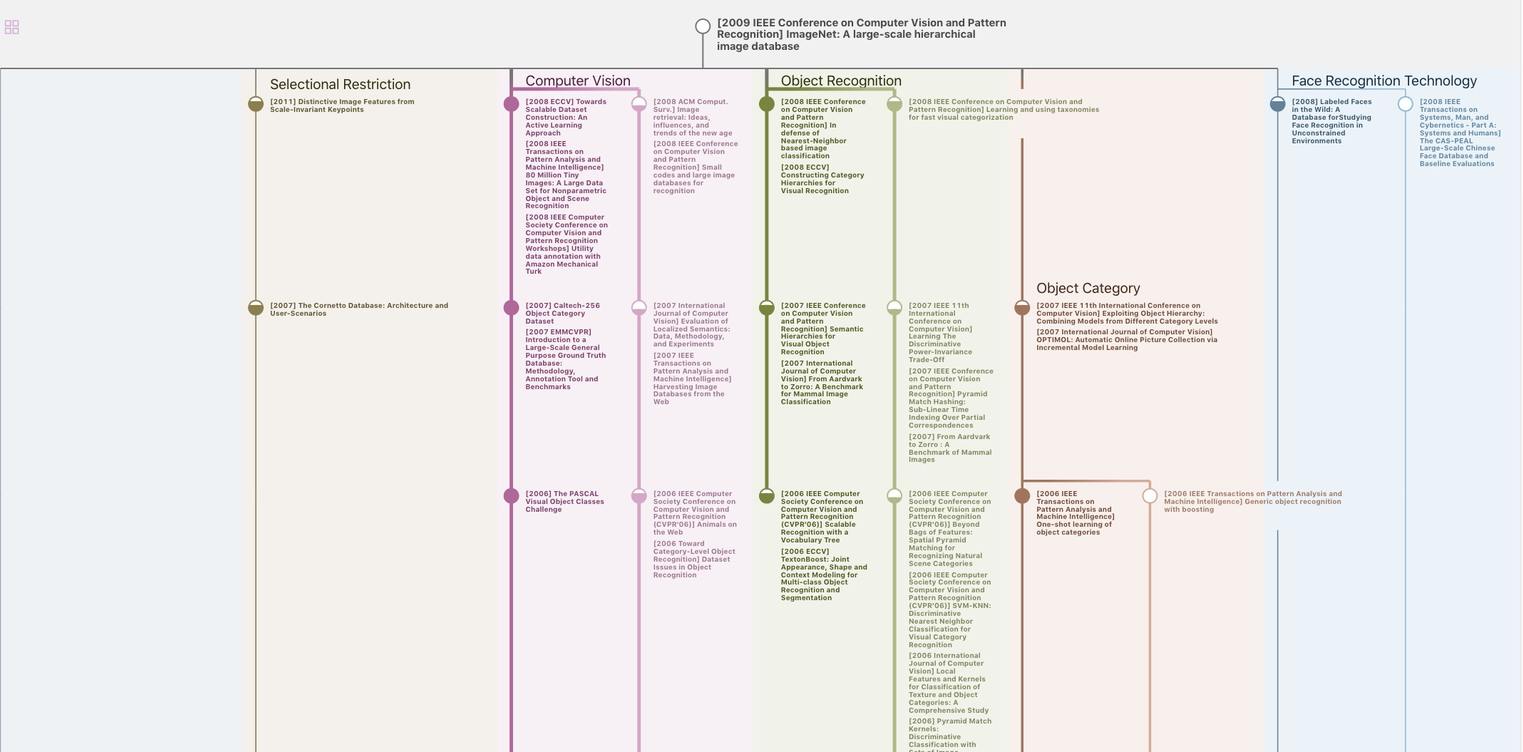
生成溯源树,研究论文发展脉络
Chat Paper
正在生成论文摘要