Low-Rank Correlation Learning for Unsupervised Domain Adaptation
IEEE TRANSACTIONS ON MULTIMEDIA(2024)
摘要
In unsupervised domain adaptation (UDA), negative transfer is one of the most challenging problems. Due to complex environments, the used domain data are always corrupted by noise or outliers in many applications. If the noisy data are directly used for domain adaptation, the disturbances and negative influence of the noise are also shifted for the target tasks. Thus, preventing disturbances and negative effects caused by noise are key problems in UDA that need to be addressed. In this article, a low-rank correlation learning (LRCL) method is proposed for UDA. In LRCL, the noisy domain data are recovered by low-rank learning; then both domain data are cleaned. Hence, the disturbances and negative effects of the noise are prevented. The maximized correlated features of the clean data from the source and target domains are learned by a novel correlation regularization term in a latent common space. LRCL also reduces the distribution difference of the learned clean source and target data by constructing a reconstruction term, in which the clean target data are linearly represented by the clean source data. To explore the temporal and structural information of the data, we further extend LRCL into a graph case and propose graph LRCL (GLRCL). Extensive experiments have been conducted on several public data benchmarks, and the experimental results demonstrate that our methods can effectively prevent negative transfer and obtain better classification outcomes than other compared approaches.
更多查看译文
关键词
Feature extraction,Task analysis,Correlation,Training data,Electronic mail,Noise measurement,Image color analysis,domain adaptation,image classification,low-rank,transfer learning
AI 理解论文
溯源树
样例
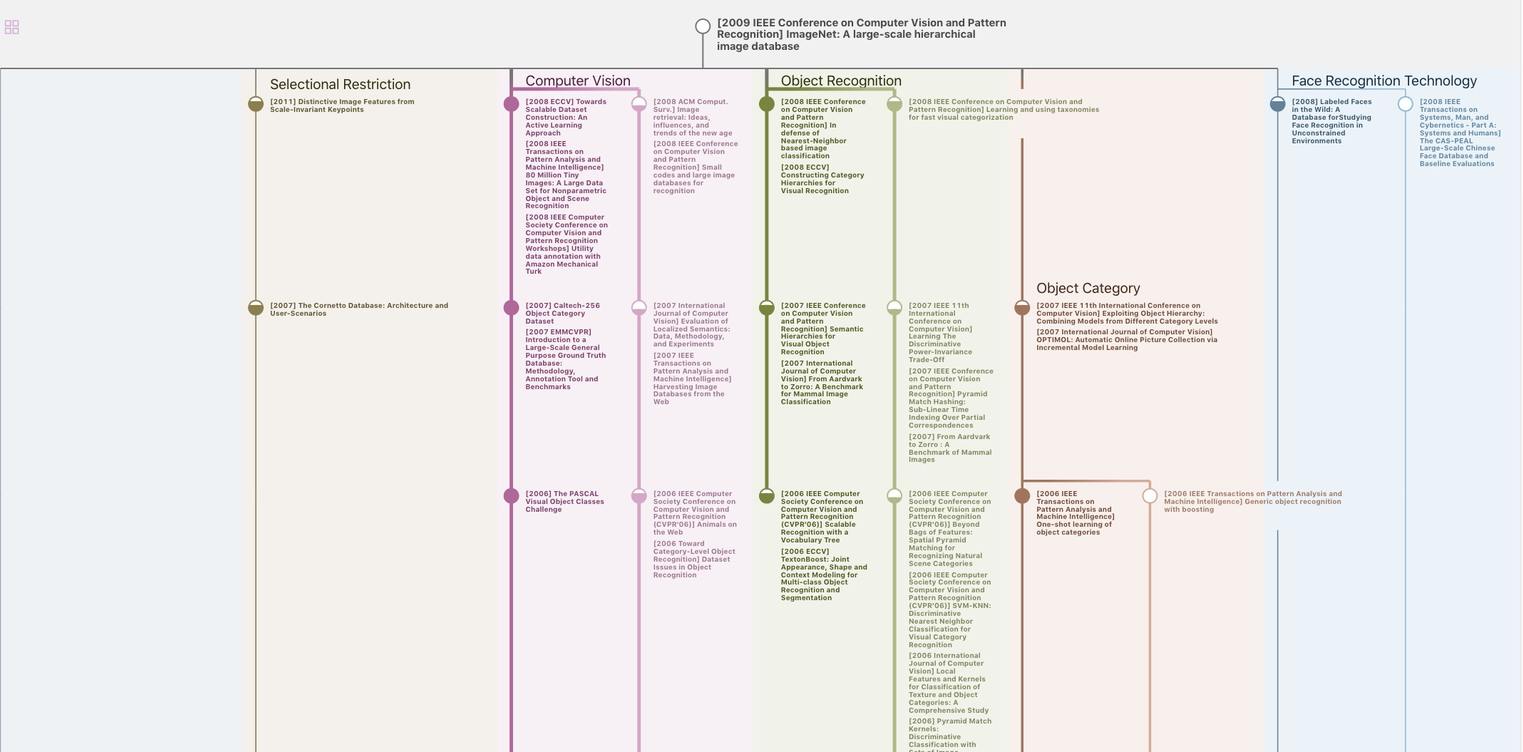
生成溯源树,研究论文发展脉络
Chat Paper
正在生成论文摘要