GLDAN: Global and Local Domain Adaptation Network for Cross-Wind Turbine Fault Diagnosis
JOURNAL OF ENGINEERING FOR GAS TURBINES AND POWER-TRANSACTIONS OF THE ASME(2024)
Abstract
Operating under harsh conditions and exposed to fluctuating loads for extended periods, wind turbines experience a heightened vulnerability in their key components. Early fault detection is crucial to enhance the reliability of wind turbines, minimize downtime, and optimize power generation efficiency. Although deep learning techniques have been widely applied to fault diagnosis tasks, yielding remarkable performance, practical implementations frequently confront the obstacle of acquiring a substantial quantity of labeled data to train an effective deep learning model. Consequently, this paper introduces an unsupervised global and local domain adaptation network (GLDAN) for fault diagnosis across wind turbines, enabling the model to efficiently transfer acquired knowledge to the target domain in the absence of labeled data. This feature renders it an appropriate solution for situations with limited labeled data availability. Employing adversarial training, GLDAN aligns global domain distributions, diminishing the overall discrepancy between source and target domains, and local domain distributions within a single fault category for both domains, capturing more intricate and specific fault features. The proposed approach is corroborated using actual wind farm data, and comprehensive experimental results demonstrate that GLDAN surpasses deep global domain adaptation methods in cross-wind turbine fault diagnosis, underlining its practical value in the field.
MoreTranslated text
Key words
wind turbines,fault diagnosis,transfer learning,domain adaptation,global and local domain adaptation network
AI Read Science
Must-Reading Tree
Example
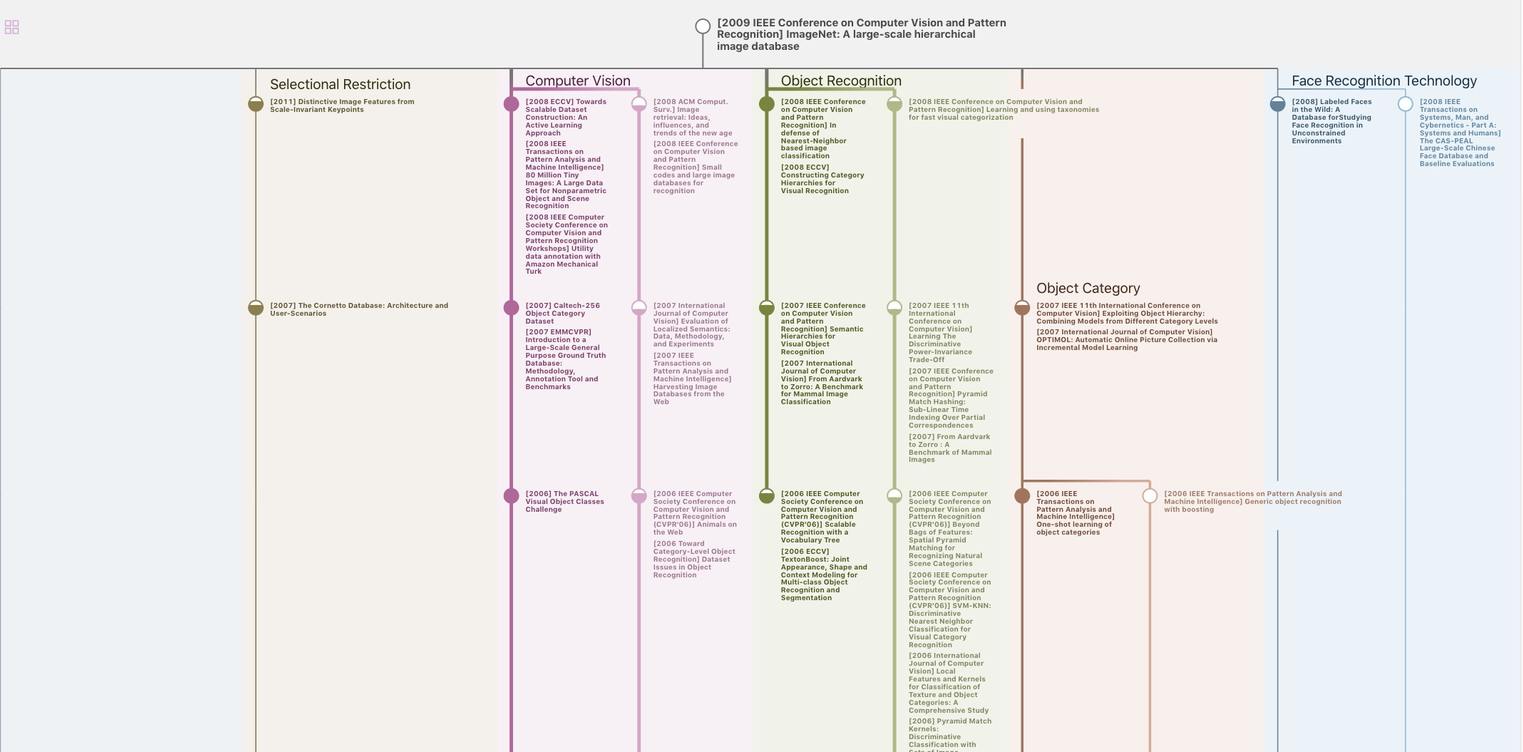
Generate MRT to find the research sequence of this paper
Chat Paper
Summary is being generated by the instructions you defined