Deep learning multimodal methods for geophysical inversion : application to glacier ice thickness estimation
20TH INTERNATIONAL CONFERENCE ON CONTENT-BASED MULTIMEDIA INDEXING, CBMI 2023(2023)
摘要
Glaciers play a critical role in the Earth's climate system, and accurate estimates of their behaviours are essential for understanding the impacts of climate change and informing policy decisions. One of the most important parameters for such a task is ice distribution, which is difficult to measure and predict using traditional physics-based models. In this study, we propose a deep learning approach to predict glacier thickness by learning directly from ice velocity and topography. Our approach overcomes the limitations of traditional physics-based models, such as computational cost and the need for expert knowledge to calibrate the models. In addition, deep learning models are flexible enough to explore the relevance of multimodality and multitasking to address the physical problem. Our results demonstrate the feasibility of quickly training a neural network model with sufficient training data and producing stable, high-quality ice thickness estimates. We highlight the importance of some specific input features suggested by geophysicists that have a positive impact on model stability.
更多查看译文
关键词
deep learning,multi-modal,multi-task,glaciology,geophysical inversion
AI 理解论文
溯源树
样例
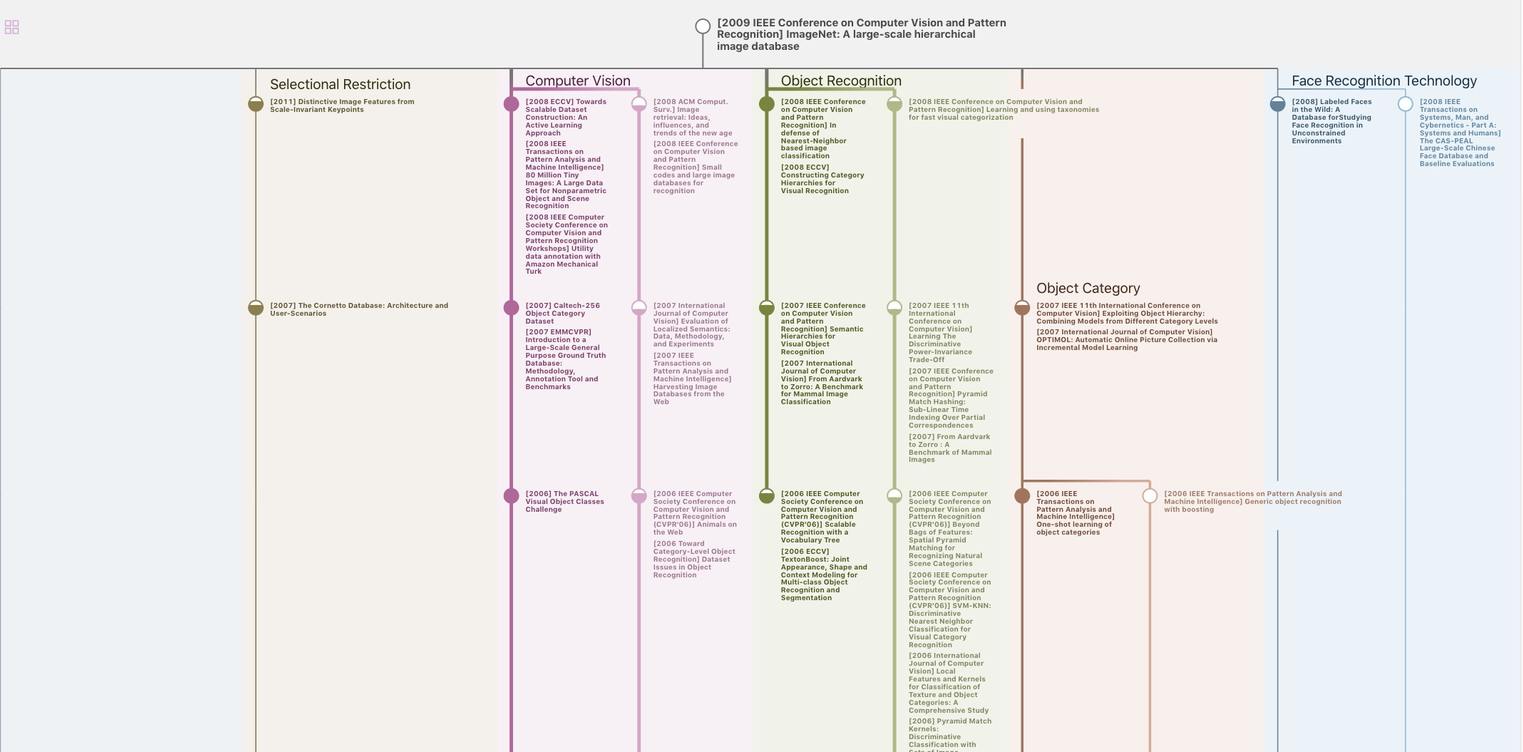
生成溯源树,研究论文发展脉络
Chat Paper
正在生成论文摘要