Ground Motion Shaking Predictions Based on Machine Learning and Physics-based Simulations
Research Square (Research Square)(2023)
摘要
After large-magnitude earthquakes occur, a crucial task for impact assessment is to rapidly and accurately estimate the ground shaking intensities in the affected region. The intensity response can be obtained through computationally demanding simulations of wave propagation phenomena, which are accurate but still slow to produce timely results. To satisfy real-time constraints, ground shaking is traditionally evaluated with approximated Ground Motion Models (GMMs) that can drastically limit the accuracy of the estimated intensity map. In this work, one of the largest existent datasets (with more than 100M simulated seismograms), from CyberShake Study 15.4 developed by the Southern California Earthquake Center covering the Los Angeles basin region, was used to train and validate several Machine Learning (ML) strategies as an alternative to GMMs. Our predictions using the ML-based EStimator for ground-shaking maps (MLESmap) strategy outperform GMM solutions requiring similar evaluation times. We show a reduction in the median of the root mean square error (RMSE) up to 45\% for a set of unseen samples of the synthetic database, and a reduction in RMSE between 11\% and 88\% for real historical earthquakes relative to GMM predictions.
更多查看译文
关键词
shaking,simulations,ground,motion,machine learning,physics-based
AI 理解论文
溯源树
样例
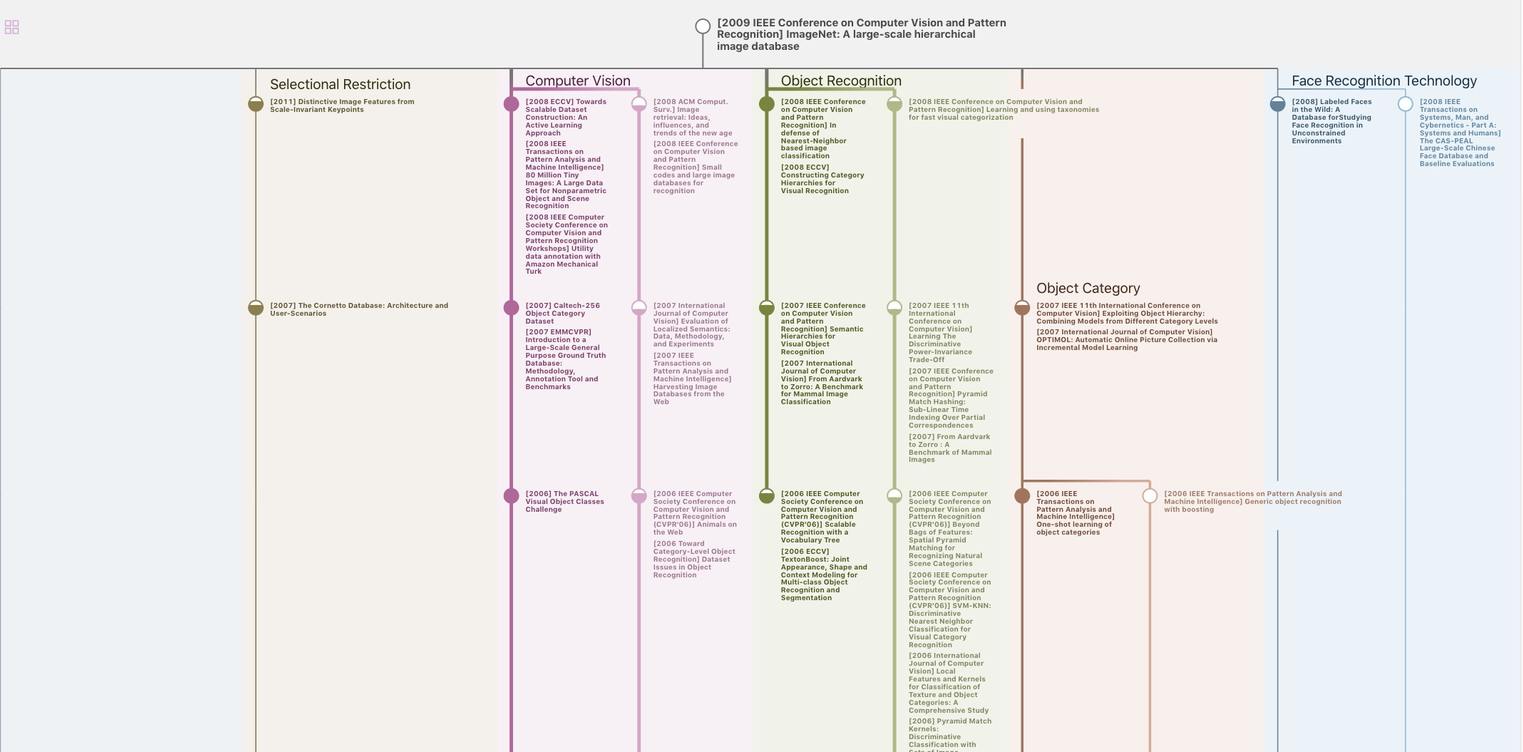
生成溯源树,研究论文发展脉络
Chat Paper
正在生成论文摘要