Machine-Learning Approach for Prognosis of Oscillating Water Column Wave Generators
WSEAS transactions on computers(2023)
Abstract
Wave excitations cause structural vibrations on the Oscillating Water Columns (OWC) lowering the power generated and reducing the life expectancy. The problem of generator deterioration has been considered for the Mutriku MOWC plant and a machine learning-based approach for prognosis and fault characterization has been proposed. In particular, the use of k-Nearest Neighbor (kNN) models for predicting the time to failure of OWC generators has been proposed. The analysis is based on data collected from sensors that measure various operational parameters of the turbines. The results demonstrate that the proposed kNN model is an excellent choice for reducing maintenance costs by enabling maintenance scheduling months in advance. The model's high accuracy in predicting generator failures allows for timely and cost-effective maintenance, preventing costly breakdowns and improving turbine efficiency. The results highlight the potential of machine learning-based approaches for addressing maintenance challenges in the energy sector and underscore the importance of proactive maintenance strategies in reducing operational costs and maximizing energy production.
MoreTranslated text
Key words
machine-learning machine-learning,wave,prognosis
AI Read Science
Must-Reading Tree
Example
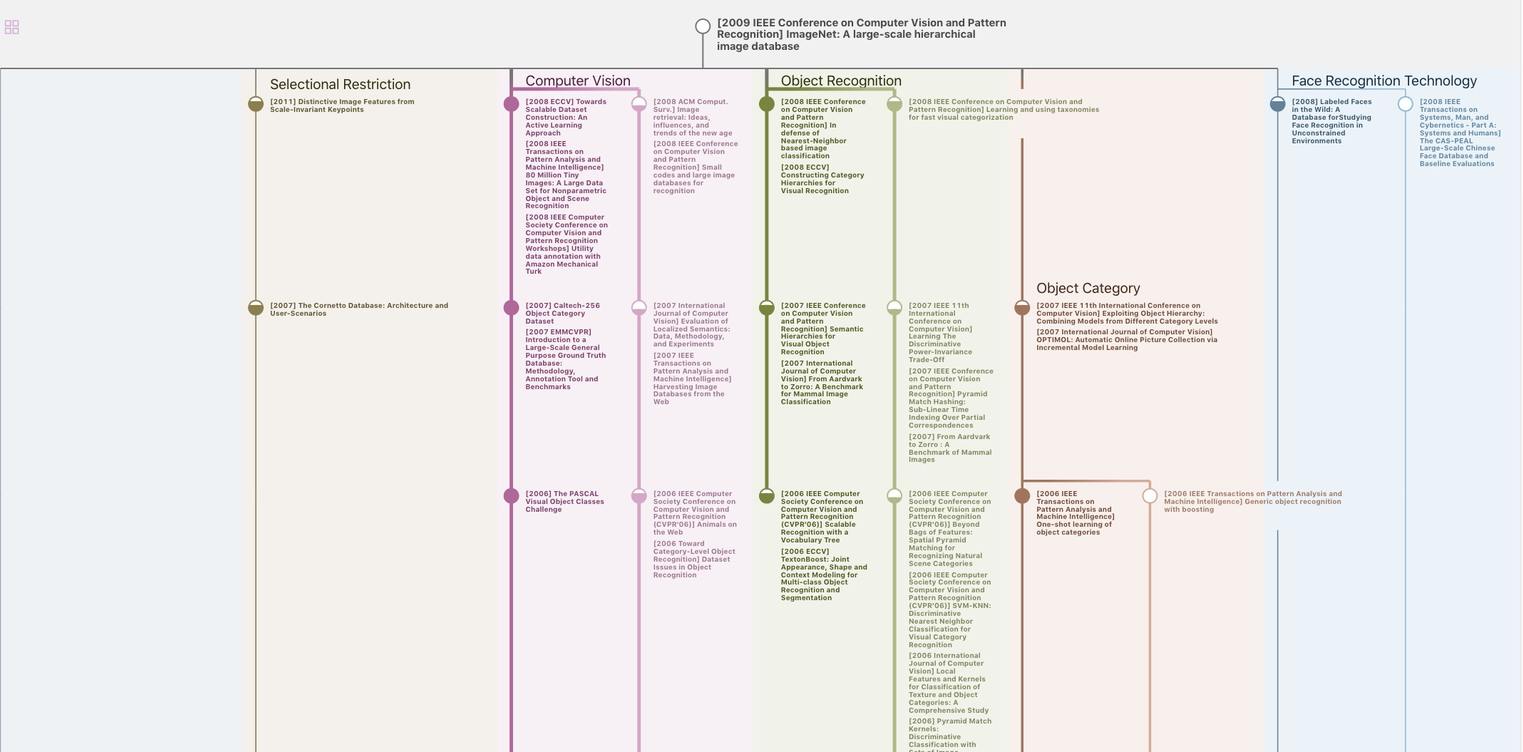
Generate MRT to find the research sequence of this paper
Chat Paper
Summary is being generated by the instructions you defined