Understanding cloud systems structure and organization using a machine’s self-learning approach
Artificial Intelligence for the Earth Systems(2023)
Abstract
Abstract In this study, we introduce a self-supervised deep neural network approach to classify satellite images into independent classes of cloud systems. The driving question of the work is to understand whether our algorithm can capture cloud variability and identify distinct cloud regimes. Ultimately, we want to achieve generalization such that the algorithm can be applied to unseen data and thus help automatically extract relevant information important to atmospheric science and renewable energy applications from the ever-increasing satellite data stream. We use cloud optical depth (COD) retrieved from postprocessed high-resolution Meteosat Second Generation (MSG) satellite data as input for the network. The network’s architecture is based on the DeepCluster, version 2, and consists of a convolutional neural network and a multilayer perceptron, followed by a k -means algorithm. We explore the network’s training capabilities by analyzing the centroids and feature vectors found from progressive minimization of the cross-entropy loss function. By making use of additional MSG retrieval products based on multichannel information, we derive the optimum number of classes to determine independent cloud regimes. We test the network capabilities on COD data from 2013 and find that the trained neural network gives insights into the cloud systems’ persistence and transition probability. The generalization on the 2015 data shows good skills of our algorithm with unseen data, but results depend on the spatial scale of cloud systems. Significance Statement This study uses a self-supervised deep neural network to identify distinct cloud systems from cloud optical depth satellite images over central Europe. Satellite-retrieved products support the physical interpretation of the identified cloud classes and help optimize the number of identified classes. The trained neural network gives insights into cloud systems’ persistence and transition probability. The generalization capacity of the deep neural network with unseen data is promising but depends on the spatial scale of cloud systems.
MoreTranslated text
Key words
cloud systems structure,organization,self-learning
AI Read Science
Must-Reading Tree
Example
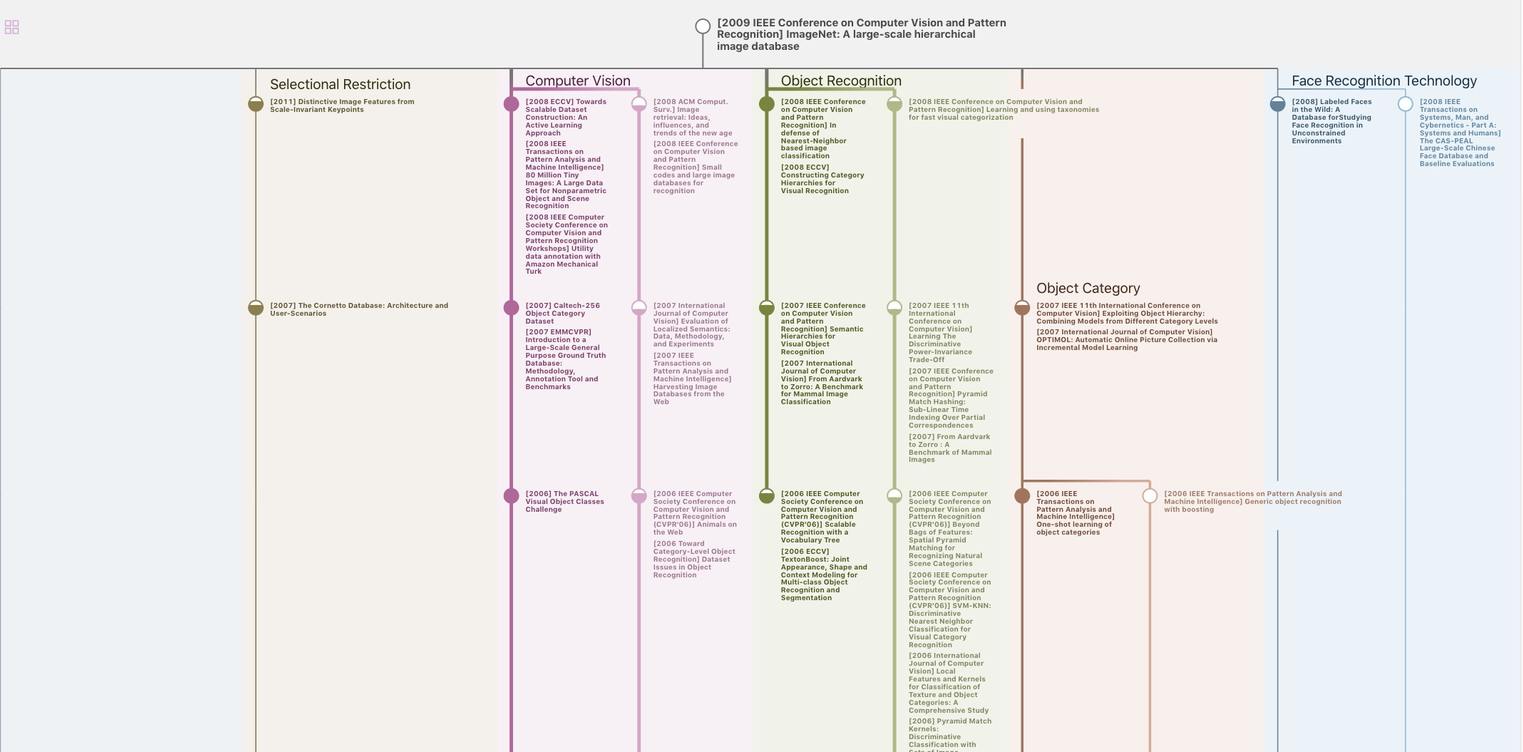
Generate MRT to find the research sequence of this paper
Chat Paper
Summary is being generated by the instructions you defined