Leveraging Multi-Task Learning in Code-Switched setting
Research Square (Research Square)(2023)
Abstract
Multilingualism is the ability to converse fluently in more than one language. In multilingual societies, code-switching, the practise of switching languages during a conversation, occurs often, creating a need for multilingual dialogue and voice recognition systems in natural language processing (NLP). However, interpreting code-switching utterances is extremely difficult for these NLP systems, as the model must adjust to code-switching patterns. In recent years, deep learning strategies have enabled natural language systems with massive volumes of training data to attain human-level performance in languages. However, they cannot accommodate a large number of low-resource languages, mostly mixed languages. Also, code-switching, despite being a common occurrence, is unique to spoken language and lacks the transcriptions necessary for training deep learning models. Our work in this paper, explores the usage of multi-task learning in code-switched setting to see if it can leverage from the training signals of related tasks and improve the generalizability as well as boost the performance and metrics of our model.
MoreTranslated text
Key words
learning,setting,multi-task,code-switched
AI Read Science
Must-Reading Tree
Example
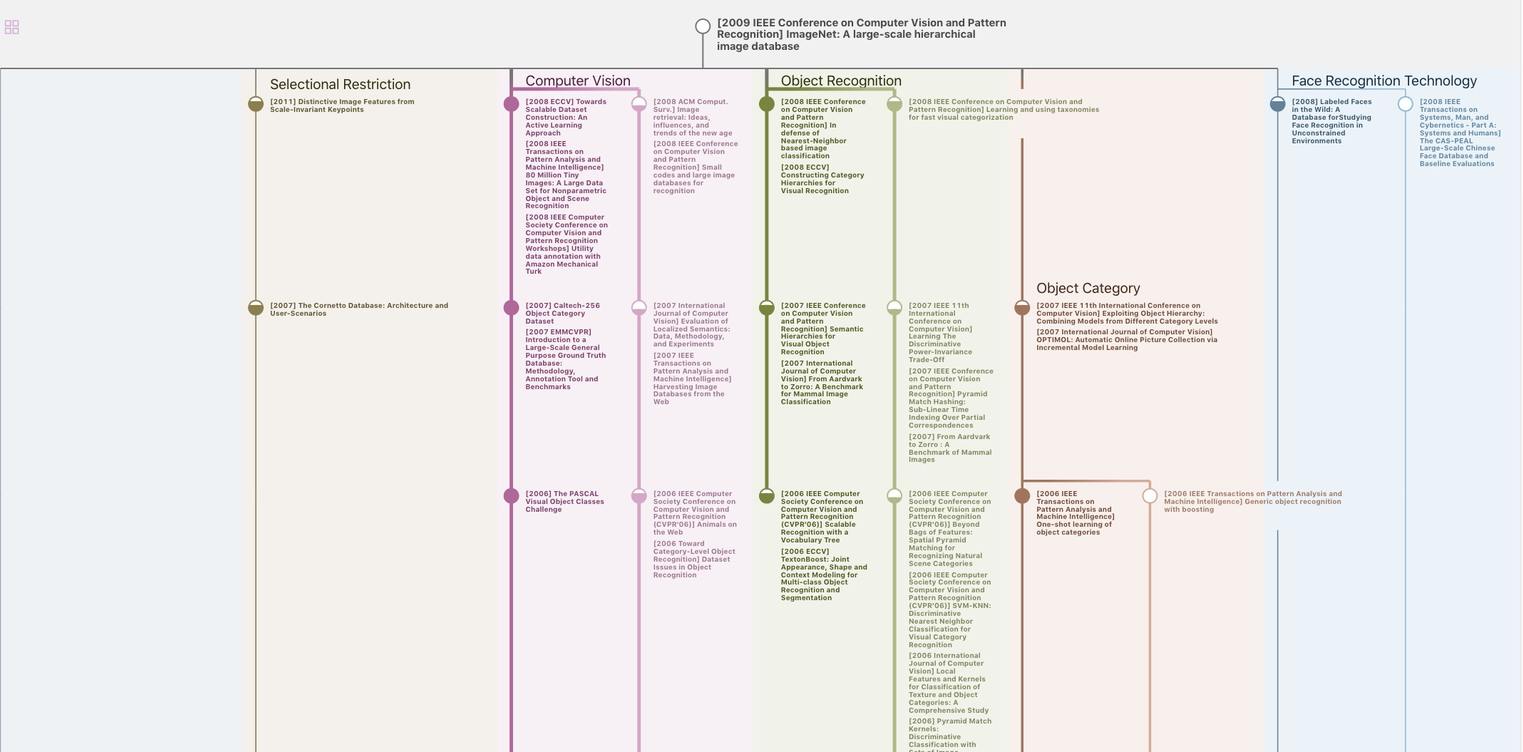
Generate MRT to find the research sequence of this paper
Chat Paper
Summary is being generated by the instructions you defined