Mathematical modelling of survival in low grade gliomas at malignant transformation
Neuro-Oncology(2023)
摘要
Abstract AIMS Low grade gliomas (LGG) represent 14.6% of gliomas and may remain stable years after initial diagnosis. MRI features suggesting malignant transformation (MT) include enlargement of non-enhancing areas and increasing enhancement but have low specificity. We report the first stages of a deep learning model to predict survival using radiomic feature extraction at MT, clinical and molecular genetics. METHOD Retrospective analysis of all LGGs treated at our institution (2005 - 2020) with histology, molecular genetics and MRIs. 766 patients: 457 IDH mutant astrocytomas, 251 IDH mutant 1p19q co-deleted oligodendrogliomas and 58 other gliomas; 398 male, 368 female; mean age 51 years; range 16-89. MRIs underwent in-house tumour segmentation pipeline with radiomic feature extraction of whole tumour (wt), enhancing (enh), non-enhancing (ne) component and oedema components. RESULTS At MT, mean wt volume was 36404mm3 (SD 37213mm3) with mean 2D-diameter of 75.90mm (SD 27.23mm). Mean tissue compartmental volumes: enh 9539mm3 (SD 17578mm3), ne 26865mm3 (SD 33796mm3), and oedema 61067mm3 (SD 61535 mm3). 92 lesions contained no post-contrast enhancing tissue. Lesions were more commonly frontal or temporally based, with relative sparing of parietal and occipital lobes. No predilection for left or right hemisphere. CONCLUSIONS Majority of LGGs demonstrate contrast enhancing lesions at MT in a frontal or temporal spatial distribution with no hemispheric predilection. We plan to extract the MRI features at MT to build a survival model with clinical, treatment and molecular genetic data. Defining the radiological features at MT would enable prognostication and support clinical decision-making in this patient group.
更多查看译文
关键词
low grade gliomas,survival,mathematical modelling
AI 理解论文
溯源树
样例
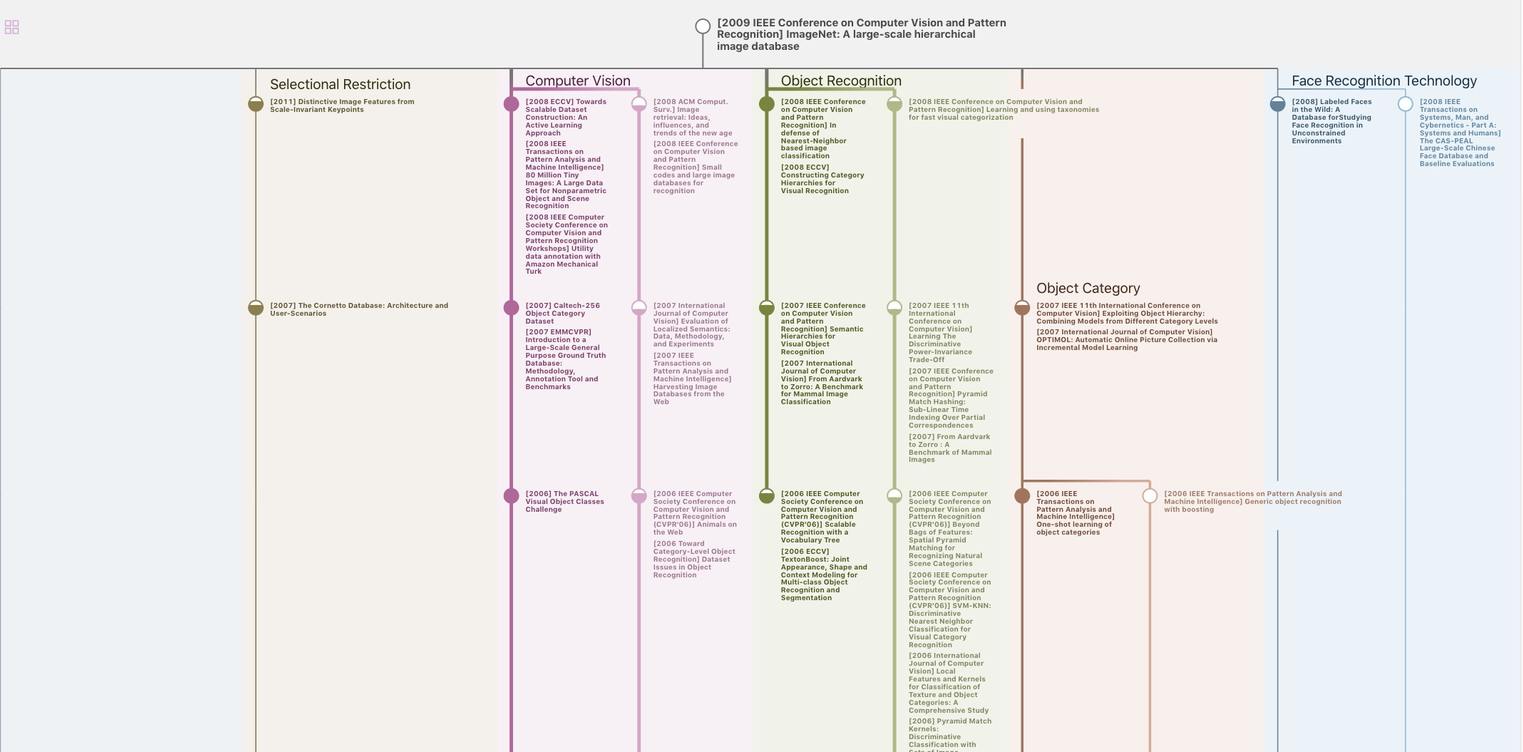
生成溯源树,研究论文发展脉络
Chat Paper
正在生成论文摘要