Bi-Level Adaptive Storage Expansion Strategy for Microgrids Using Deep Reinforcement Learning.
IEEE Trans. Smart Grid(2024)
摘要
Battery energy storage (BES) is a versatile resource for the secure and economic operation of microgrids (MGs). Prevailing stochastic optimization-based approaches for BES expansion planning for MGs are computationally complicated. This work proposes a data-driven bi-level multi-period BES expansion planning framework to determine the siting, sizing, and timing of BES installations. The proposed planning framework unifies deep reinforcement learning (DRL) and linear programming, thereby decoupling the determinations for the integer and continuous decision variables in two time scales, respectively. In the upper level, a rainbow DRL agent with quantile regression is trained to provide dynamic planning policies to accommodate stochastic renewable energy resources (RESs), load, and battery price changes efficiently. The lower level computes the optimal operation of MGs with frequency constraints to hedge the islanding contingency. The two levels communicate with one another by exchanging storage configuration and operating expenses in order to accomplish the shared goal of minimizing investment and operation costs. Comparative case studies on an MG are carried out to demonstrate the superiority of the proposed DRL-based solution to the mixed-integer linear programming counterpart on efficiency, scalability, and adaptability.
更多查看译文
关键词
battery storage systems,deep reinforcement learning,microgrid planning,two-timescale
AI 理解论文
溯源树
样例
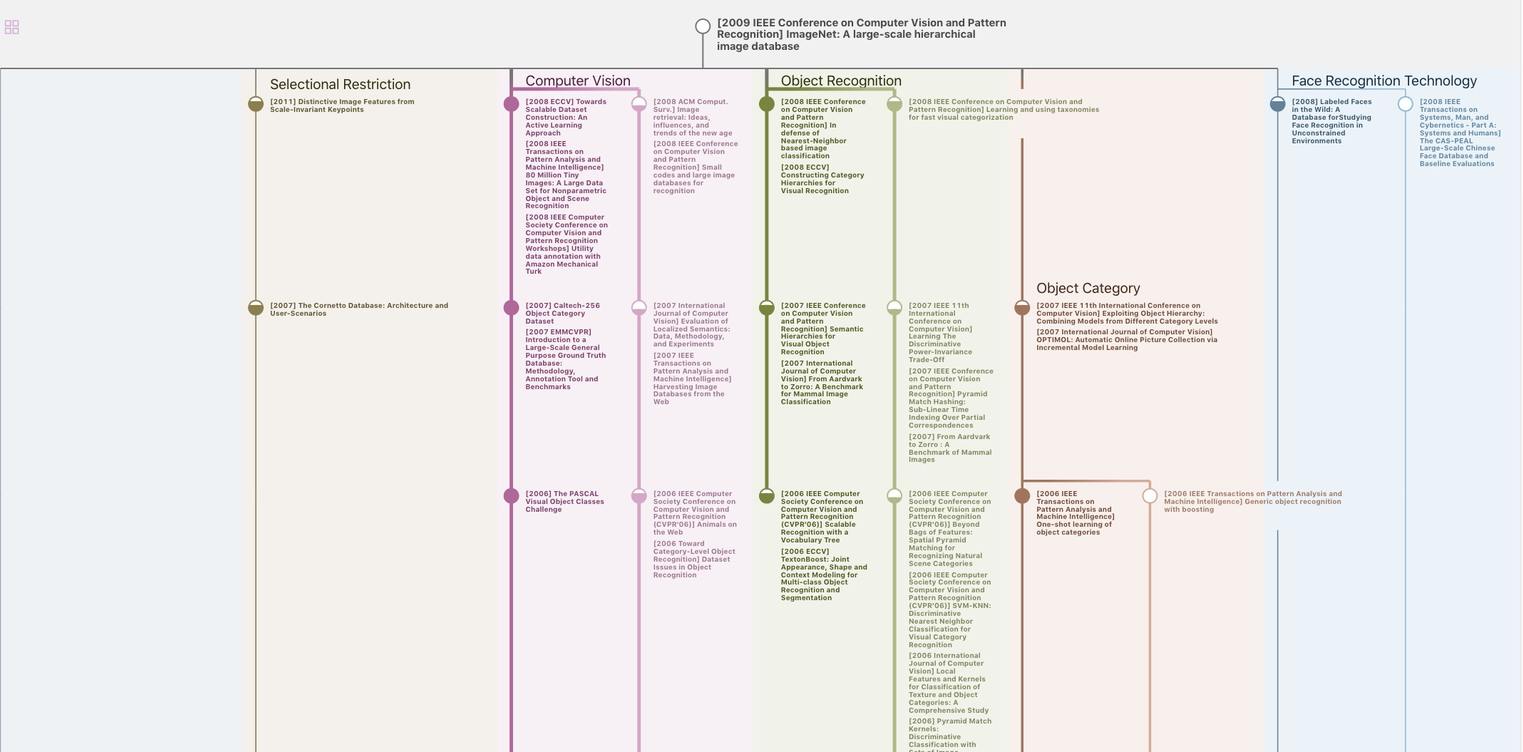
生成溯源树,研究论文发展脉络
Chat Paper
正在生成论文摘要