Machine Learning-based maternal health risk prediction model for IoMT framework
International journal of experimental research and review(2023)
摘要
The Internet of Things (IoT) is vital as it offers extensive applicability in various fields, including healthcare. In the context of the risk level during pregnancy, to monitor and predict abnormalities, IoT devices provide a means to collect real-time health data, enabling continuous monitoring and analysis in the Internet of Medical Things (IoMT) environments. By integrating IoT devices into the system, crucial signs such as Heart Rate (HR), Systolic and Diastolic Blood Pressure (BP), Fetal Movements (FM), and Temperature (T) can be tracked remotely and non-invasively. This allows for the timely detection of abnormalities or potential risk factors during pregnancy, empowering healthcare professionals to intervene proactively and provide personalized care. This research focuses on developing a system for observing and predicting the maternal risk level in the IoT environment, mainly in remote areas. The goal is to improve maternal health and reduce maternal and child mortality rates, a significant decline according to United Nations targets for 2030. The research utilizes analytical tools and Machine Learning (ML) algorithms to analyze health data and risk factors associated with pregnancy. The acquired dataset contains various risk factors categorized and classified based on intensity. After comparing different ML models’ experimental results, Exploratory Data Analysis (EDA) approaches to determine the most effective risk factors. The fine-tuned Random Forest Classifier (RF) achieves the highest accuracy of 93.14%. An Android-based application has also been developed to deploy the prediction model to determine risk levels based on the different parameters.
更多查看译文
关键词
prediction model,learning-based
AI 理解论文
溯源树
样例
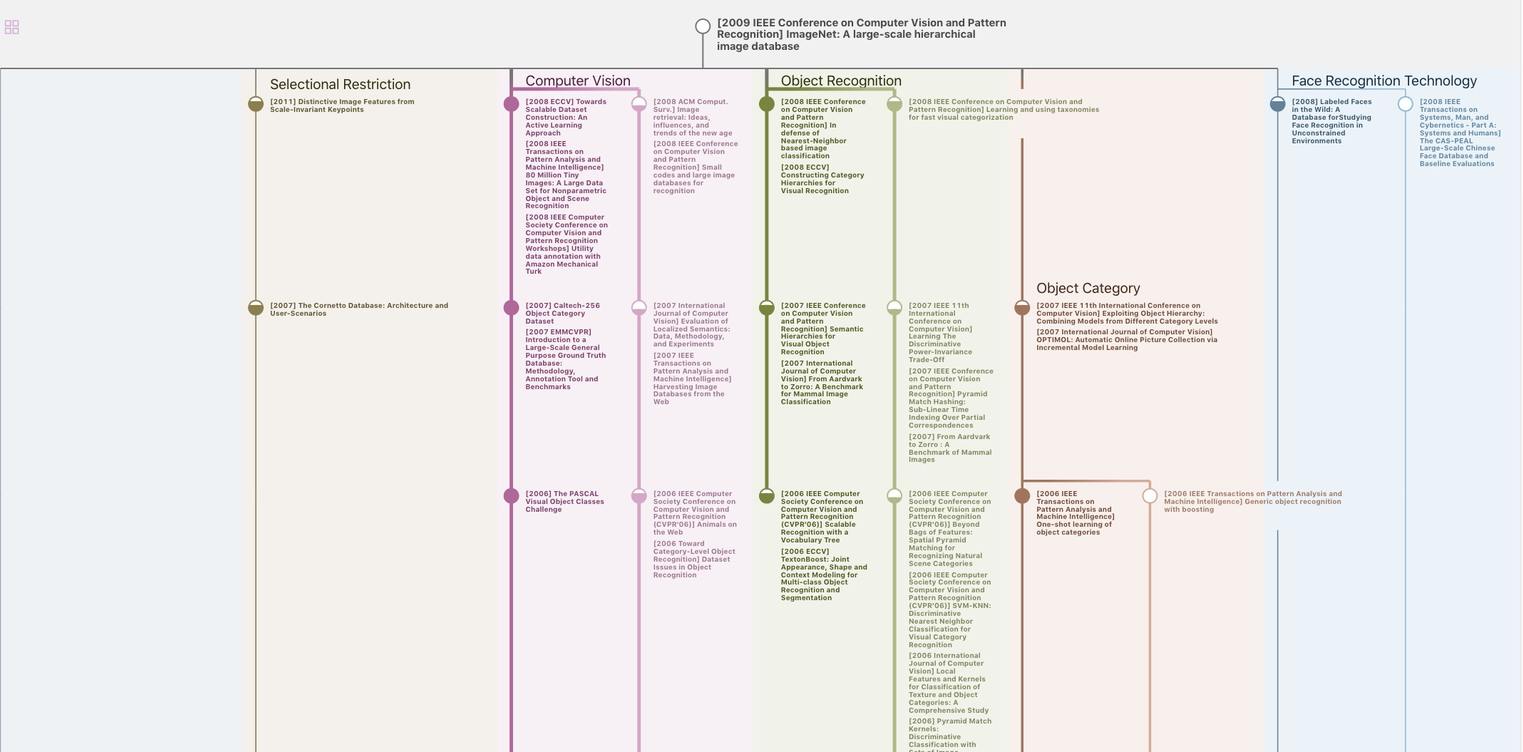
生成溯源树,研究论文发展脉络
Chat Paper
正在生成论文摘要