Machine Learning Photogrammetric Analysis of Images Provides a Scalable Approach to Study Riverbed Grain Size Distributions
EarthArXiv (California Digital Library)(2023)
摘要
The distribution of sediment grain size in streams and rivers is often quantified by the median grain size (d50), a key metric for understanding and predicting hydrologic and biogeochemical function of streams and rivers. Manual methods to measure d50 are time-consuming and ignore larger grains, while model-based methods to estimate d50 often over-generalize basin characteristics, and therefore cannot accurately represent site-scale heterogeneity. Here, we apply a machine learning photogrammetry methodology (You Only Look Once, or YOLO) for estimating d50 for grains > 2 mm based on images collected from streams and rivers throughout the Yakima River Basin (YRB). To understand how photogrammetric methods may help bridge the gaps in resolution and accuracy between manual and model-based d50 estimates, we compared YOLO d50 values to manual and model-based estimates across the YRB. We found distinct differences among methods for d50 averages and variability, and relationships between d50 estimates and basin characteristics. We discuss the advantages and limitations of the YOLO algorithm versus current methods, and explore potential future directions to combine d50 methods to better estimate spatiotemporal variation of d50, and improve incorporation into basin-scale models.
更多查看译文
关键词
images,grain,machine learning
AI 理解论文
溯源树
样例
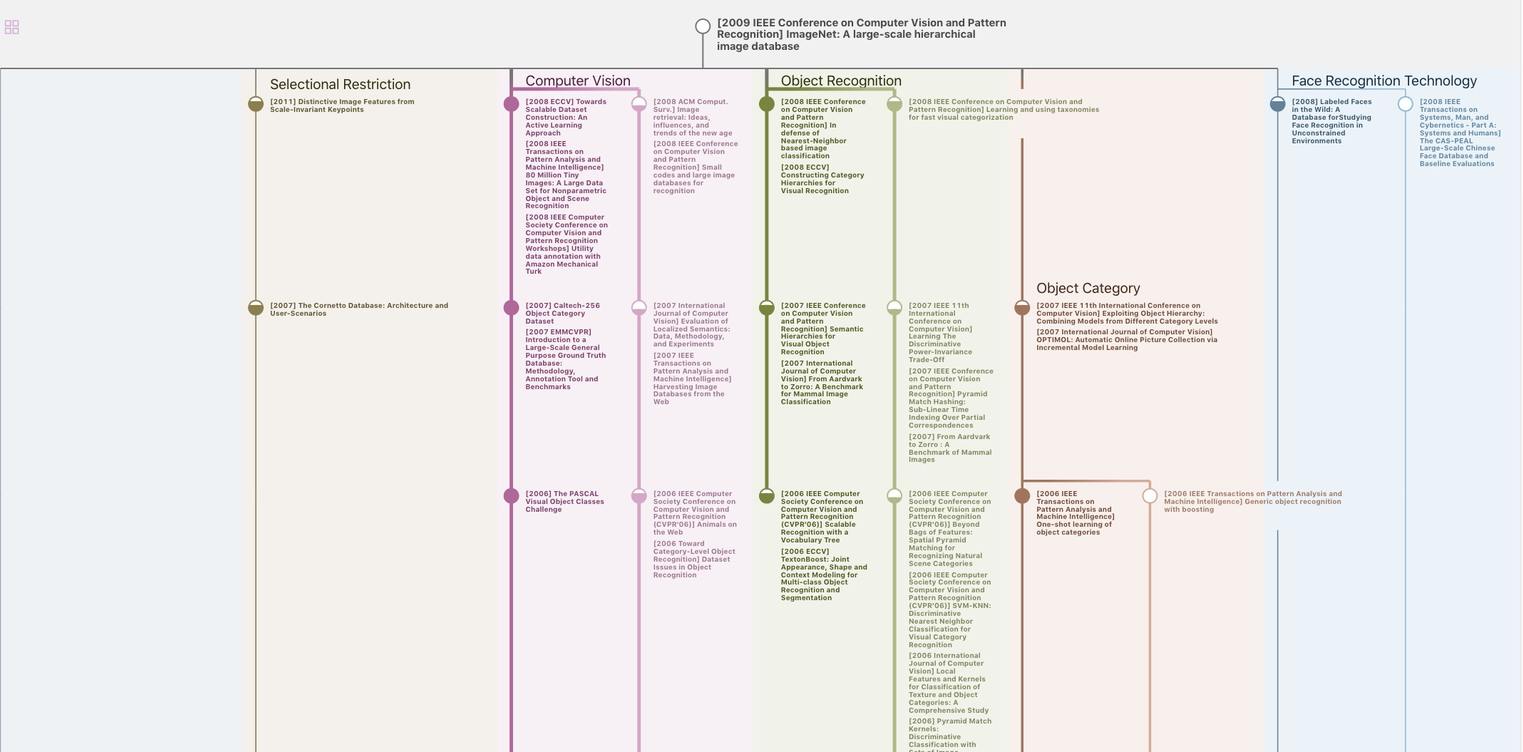
生成溯源树,研究论文发展脉络
Chat Paper
正在生成论文摘要