Cross-Modality Hierarchical Clustering and Refinement for Unsupervised Visible-Infrared Person Re-Identification
IEEE TRANSACTIONS ON CIRCUITS AND SYSTEMS FOR VIDEO TECHNOLOGY(2024)
摘要
Visible-infrared person re-identification (VI-ReID) is a challenging cross-modality image retrieval task. Compared to visible modality person re-identification that handles only the intra-modality discrepancy, VI-ReID suffers from an additional modality gap. Most existing VI-ReID methods achieve promising accuracy in a supervised setting, but the high annotation cost limits their scalability to real-world scenarios. Although a few unsupervised VI-ReID methods already exist, they typically rely on intra-modality initialization and cross-modality instance selection, despite the additional computational time required for intra-modality initialization. In this paper, we study the fully unsupervised VI-ReID problem and propose a novel cross-modality hierarchical clustering and refinement (CHCR) method by promoting modality-invariant feature learning and improving the reliability of pseudo-labels. Unlike conventional VI-ReID methods, CHCR does not rely on any manual identity annotation and intra-modality initialization. First, we design a simple and effective cross-modality clustering baseline that clusters between modalities. Then, to provide sufficient inter-modality positive sample pairs for modality-invariant feature learning, we propose a cross-modality hierarchical clustering algorithm to promote the clustering of inter-modality positive samples into the same cluster. In addition, we develop an inter-channel pseudo-label refinement algorithm to eliminate unreliable pseudo-labels by checking the clustering results of three channels in the visible modality. Extensive experiments demonstrate that CHCR outperforms state-of-the-art unsupervised methods and achieves performance competitive with many supervised methods.
更多查看译文
关键词
Cameras,Clustering algorithms,Training,Annotations,Representation learning,Intellectual property,Gray-scale,Person re-identification,cross-modality,unsupervised clustering,contrastive learning
AI 理解论文
溯源树
样例
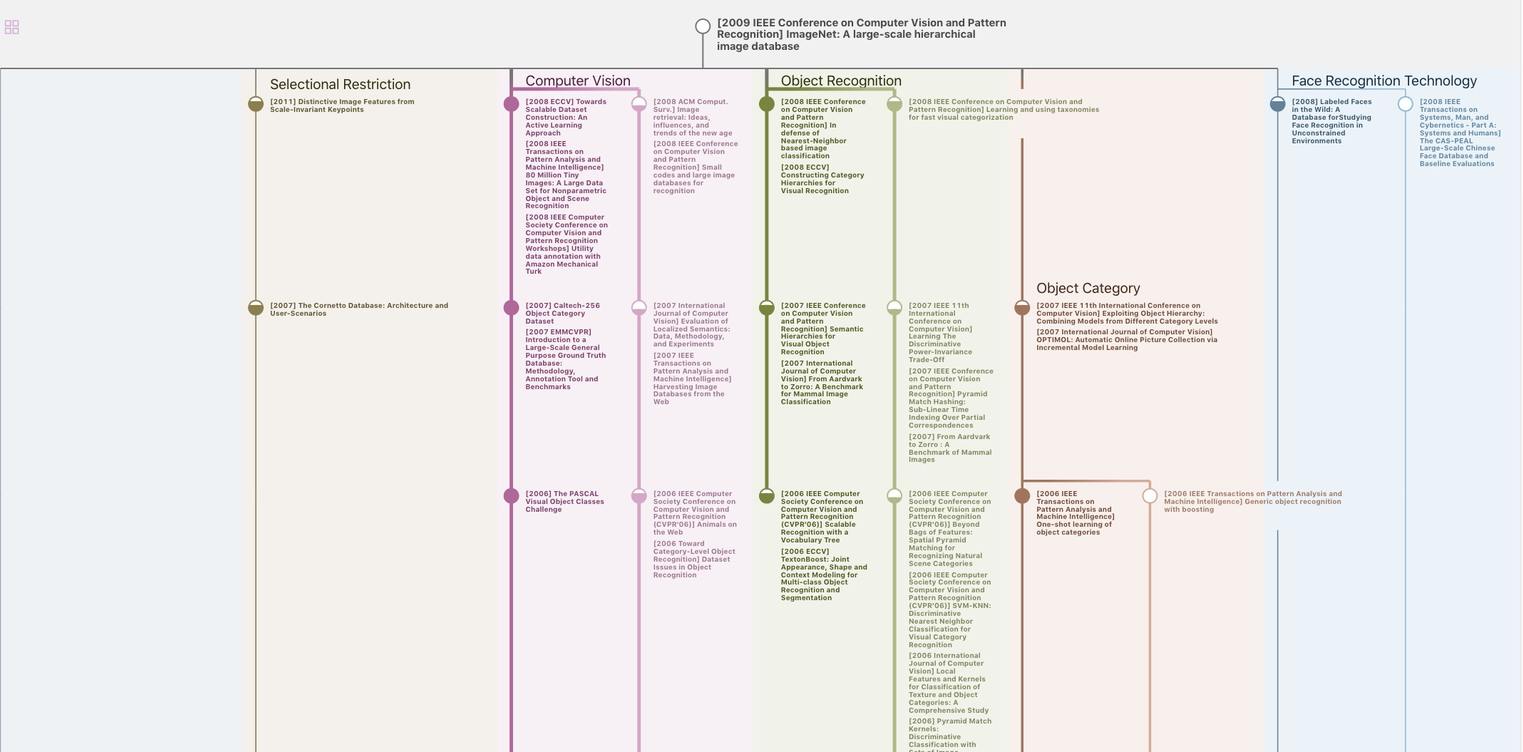
生成溯源树,研究论文发展脉络
Chat Paper
正在生成论文摘要