Evaluating Pyramid-Based Image Statistics Using Contrastive Learning
Journal of Vision(2023)
摘要
Texture models based on the steerable pyramid (Heeger & Bergen, 1995) have been long used both for texture synthesis (Portilla & Simoncelli, 1999), as well as for modeling human visual processing in areas V1/V2 (Freeman & Simoncelli, 2011), and have been adapted to model human peripheral vision (Balas et al., 2009). Yet, more work remains to determine which statistics are necessary and sufficient to fully represent textures computationally, as well as what statistics correspond to those used by the human visual system. To determine which statistics are most critical, we train a single-layer, fully-connected network to learn a representation that brings samples from the same texture together in latent space, and pushes apart samples from different textures. The network’s input is a vector of statistics from a recent peripheral vision model (Brown et al., 2021), and is trained with a contrastive learning loss. We find that this network successfully clusters both samples from the same texture, as well as families of similar textures. In analyzing the learned weight matrix, we identify the combinations of statistics useful for clustering like-textures. We perform this analysis for networks with varying numbers of output nodes, both above and below the number of input statistics. We then add a sparsity constraint that limits the representation to a single input statistic per output node, rather than a weighted combination of input statistics. We find that this network can still successfully cluster texture families, and again identify the most and least important statistics for this task. This work combines popular non-parametric statistics with learned representations, providing a simple platform to study texture representation, and giving further insight into models of mid-level vision by identifying the visual statistics most and least important for downstream visual processing.
更多查看译文
关键词
image statistics,learning,pyramid-based
AI 理解论文
溯源树
样例
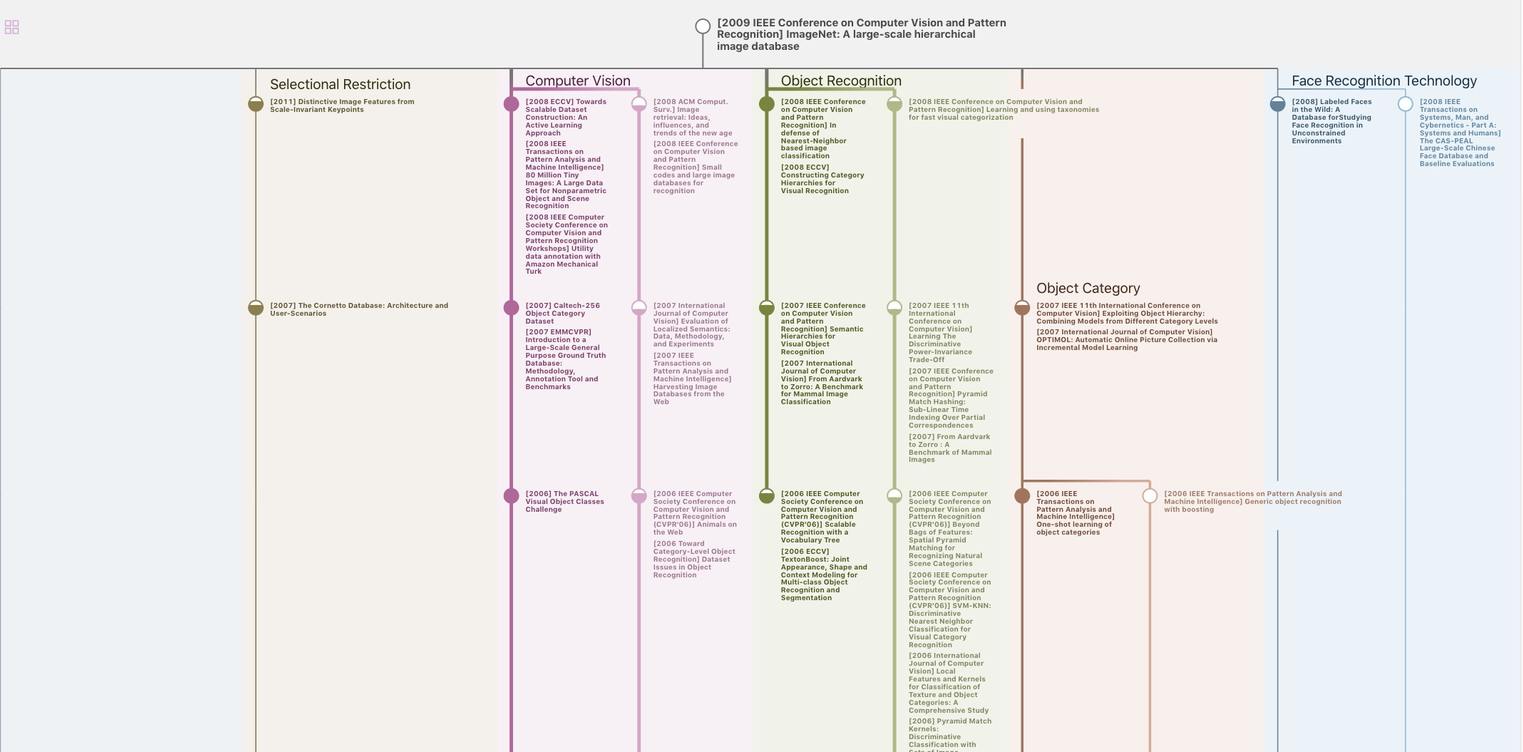
生成溯源树,研究论文发展脉络
Chat Paper
正在生成论文摘要