Toddler and preschooler attention to naturalistic scene features
Journal of Vision(2023)
摘要
Effective learning from visual exploration of our world depends on selective attention to informationally rich regions. In adults, attention is well-predicted by the distribution of semantic content, or local meaning, in a scene (Henderson & Hayes, 2017, 2018). Here, we address if meaning also predicts children’s attention in scene viewing. To investigate where 12- to 48-month-old children (N = 64, 30 girls) naturally look within scenes, we recorded children’s gaze using an SMI-Red eye tracker (at 120 Hz) toward digitized photographs of 24 real-world scenes for 6000 ms. The scenes were taken from Henderson and Hayes (2017), and adult ratings provided relative informativeness of scene patches about the meaning of scene regions, resulting in a meaning map for each scene. For example, a patch of blue sky would be judged less meaningful than a patch containing a recognizable part of a car tire. We compared toddler and preschoolers’ patterns of fixation with feature maps for meaning, physical saliency, and distance to the center of the screen. Children were more likely to fixate scene regions as meaning increased (ß = .90, z = 8.81, p < .001), saliency increased (ß = .52, z = 5.105, p < .001), and at more central regions of the scene (ß = .41, z = 5.105, p < .001). The effect of meaning was nearly double that of image saliency and center proximity. As the effect of meaning on attention increased, the effect of saliency decreased (ß = -0.14, z = -2.096, p = .036). Interestingly, we did not find that older children looked more to high meaning regions (p = .89). In conclusion, by 12 months, toddlers have refined their attention toward informative, adult-defined meaningful regions. As children explore novel objects in their visual world, this mechanism could lead to rapid accumulation of knowledge.
更多查看译文
关键词
preschooler attention,naturalistic scene features
AI 理解论文
溯源树
样例
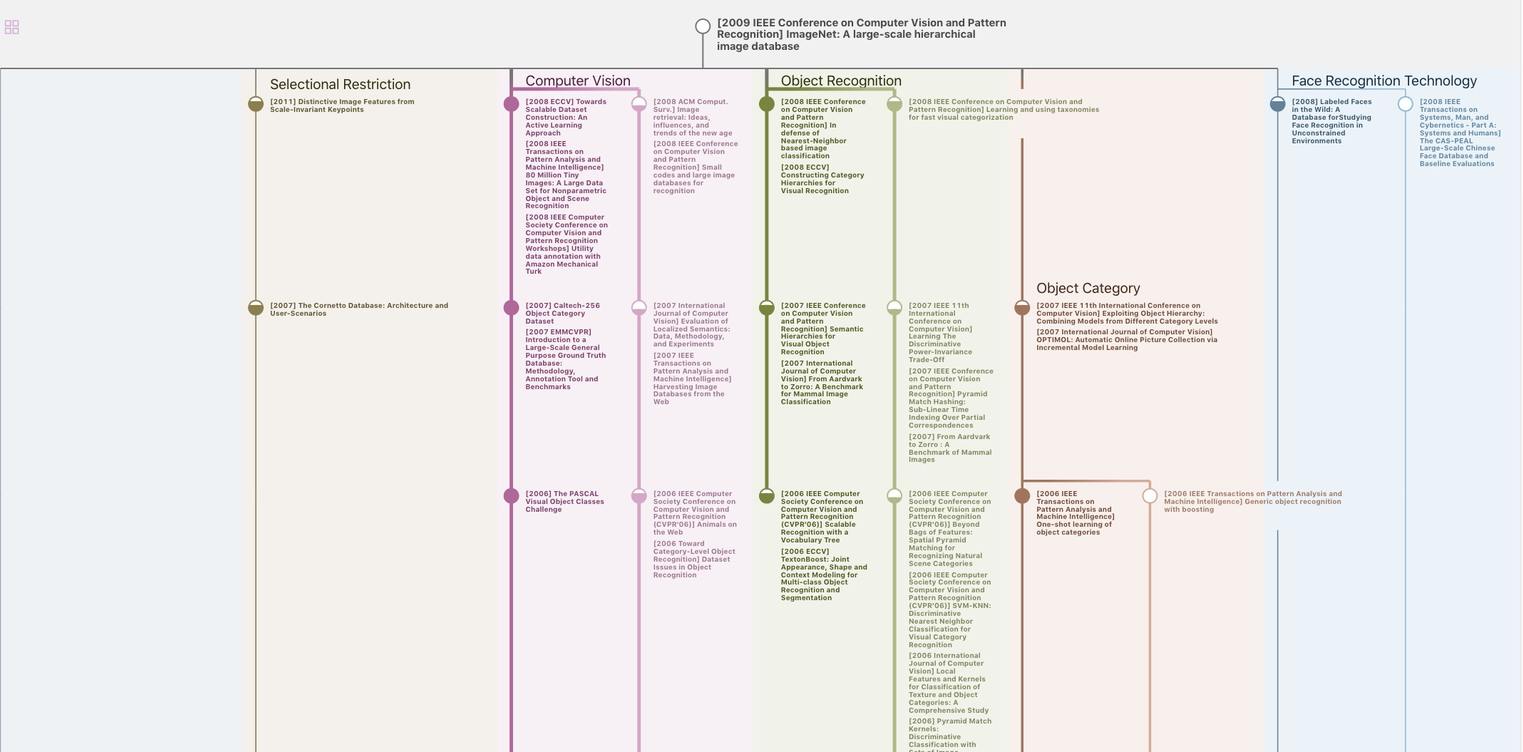
生成溯源树,研究论文发展脉络
Chat Paper
正在生成论文摘要