Efficient Filtering and Smoothing Algorithm For Train Key Components Based on Scattered Point Clouds
LASER & OPTOELECTRONICS PROGRESS(2023)
摘要
An efficient point cloud small-scale noise filtering and smoothing algorithm is proposed to address the issues of noise points and acquisition errors in the point cloud model of the key components of the train acquired by 3D laser scanning equipment. First, a K-D tree was used to construct the geometric relationship between points, set the center point, and query the neighborhood information using the K nearest neighbor algorithm. Then, it was assumed that there was a linear relationship between the input point cloud and the filtered output point cloud. Finally, the linear parameters were determined by solving the cost function's minimum value, and the smoothed point cloud model was obtained. To adjust the linear model's parameters dynamically, the weight was adaptively adjusted based on the ratio of the Euclidean distance variance of the point and its K nearest neighbor to the overall Euclidean distance variance during the construction of the cost function. The experimental results reveal that the proposed algorithm can quickly correct small-scale noise, smooth the boundary contour, improve the quality, and thereby lay the groundwork for future tasks such as point cloud recognition and reconstruction.
更多查看译文
关键词
image processing,point cloud,denoise,train key component,adaptive weighted guided filtering
AI 理解论文
溯源树
样例
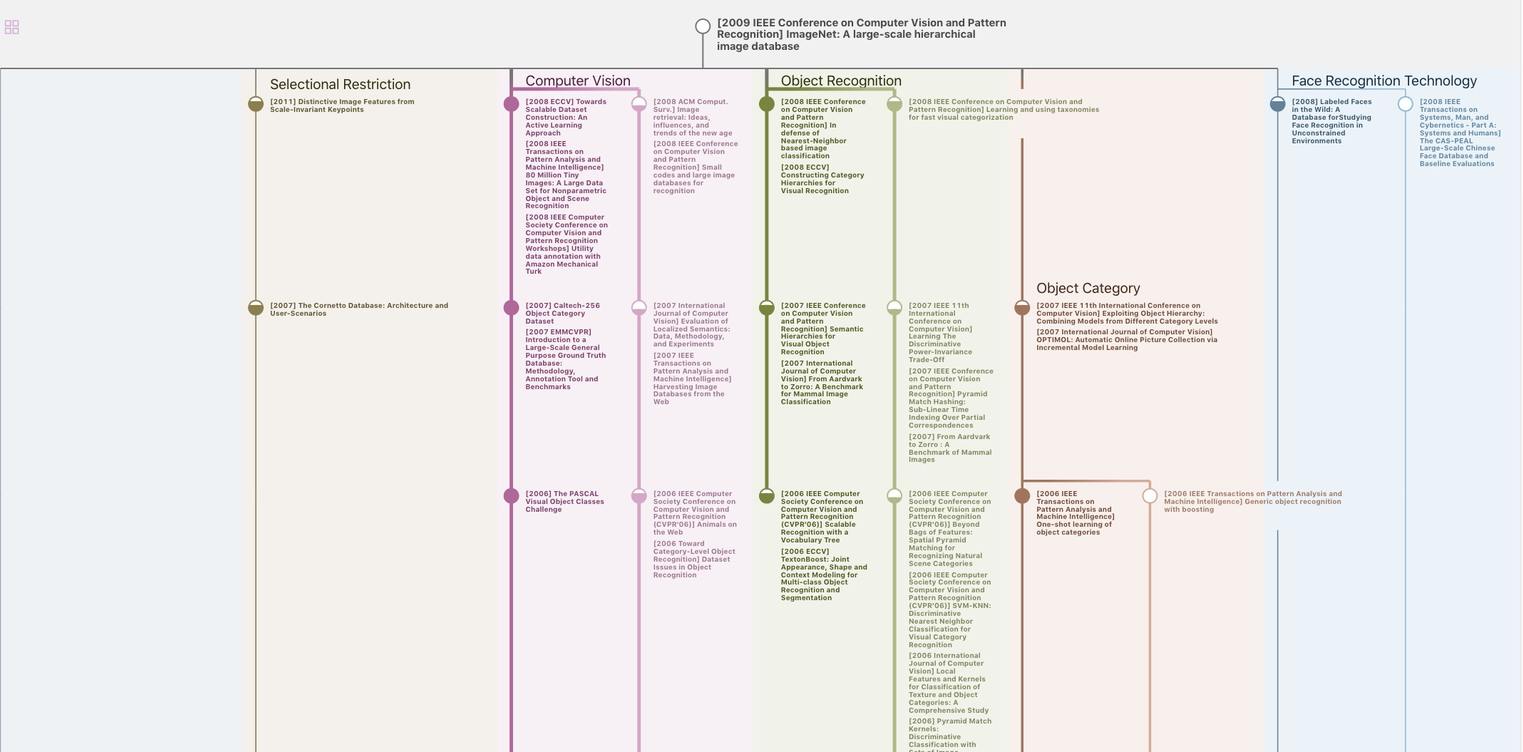
生成溯源树,研究论文发展脉络
Chat Paper
正在生成论文摘要