Deep estimation for Q* with minimax Bellman error minimization
INFORMATION SCIENCES(2023)
摘要
In this paper we consider the estimation of optimal state -action value function Q* with ReLU ResNet based on minimax Bellman error minimization. We construct the non -asymptotic error bounds for the minimax estimator and the estimated Q function induced by the estimated greedy policy. To bound the Bellman residual error, we guarantee the approximation errors based on deep approximation theory and the statistical ones by utilizing empirical processes taking into account the Markov decision process dependency. We provide a novel generalization bound with dependent data and an approximation bound in the H & ouml;lder class which are of independent interest. This bound depends on the sample size, the ambient dimension, the width and depth of the neural network, which can bring prior insights into tuning these hyper -parameters to achieve a desired convergence rate in practice. Furthermore, the bound circumvents the curse of dimensionality if the distribution of state -action pairs is assumed to be supported on a set of low intrinsic dimension.
更多查看译文
关键词
Reinforcement learning,(& lowast,)function,Deep neural networks,Minimax loss,Non-asymptotic error bound,Low intrinsic dimension
AI 理解论文
溯源树
样例
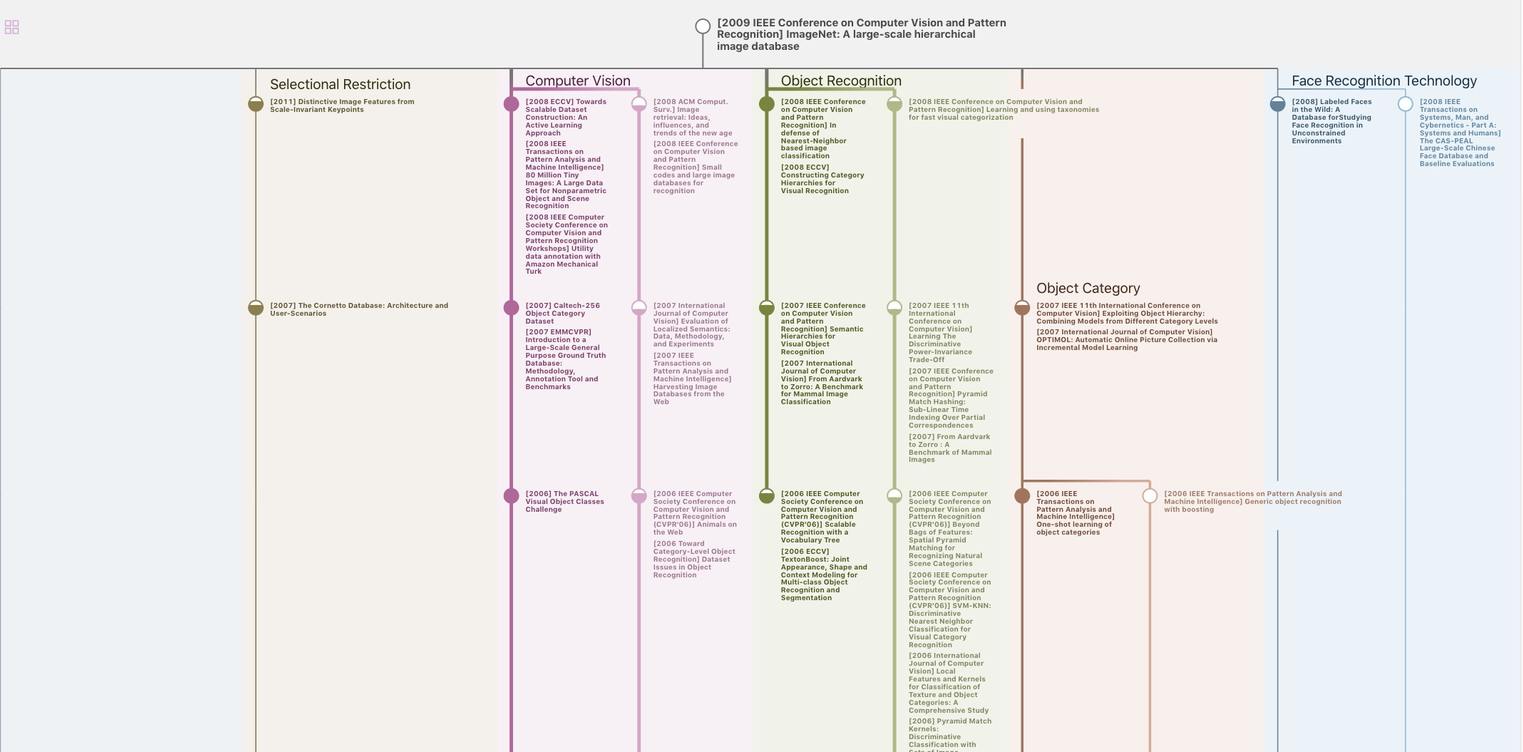
生成溯源树,研究论文发展脉络
Chat Paper
正在生成论文摘要