A machine learning model for fast approximation of free-surface Green's function and its application
Journal of Ocean Engineering and Science(2023)
摘要
In potential flow theory, the accurate and efficient calculation of free-surface Green's functions is essential for solving hydrodynamic issues. Given the impressive performance of machine learning methods in nonlinear function fitting, the present study utilizes an effective machine learning model called StripeGF for numerical approximation. In this model, equidistant horizontal datum lines are arranged in the computational domain away from the singularity, and Green's function and its derivatives on each line are fitted by a multi-layer perceptron (MLP) with a single input. Based on the first-order ordinary differential equation (ODE) that they satisfy, the fourth-order Runge-Kutta method is used to solve the Green's function and its derivatives between adjacent lines. In the domain nearing the singularity, a double-input MLP is applied. Use the Romberg quadrature to create a double-precision data set for training and validation, the numerical results demonstrate that StripeGF outperforms all 4 comparison methods in terms of efficiency and has accuracy of at least 4 digits in more than 99.9% of all zones. The boundary element program improved by StripeGF is verified in the hydrodynamic calculation of S175, showing good accuracy and reliability.
更多查看译文
关键词
green,fast approximation,machine learning model,free-surface
AI 理解论文
溯源树
样例
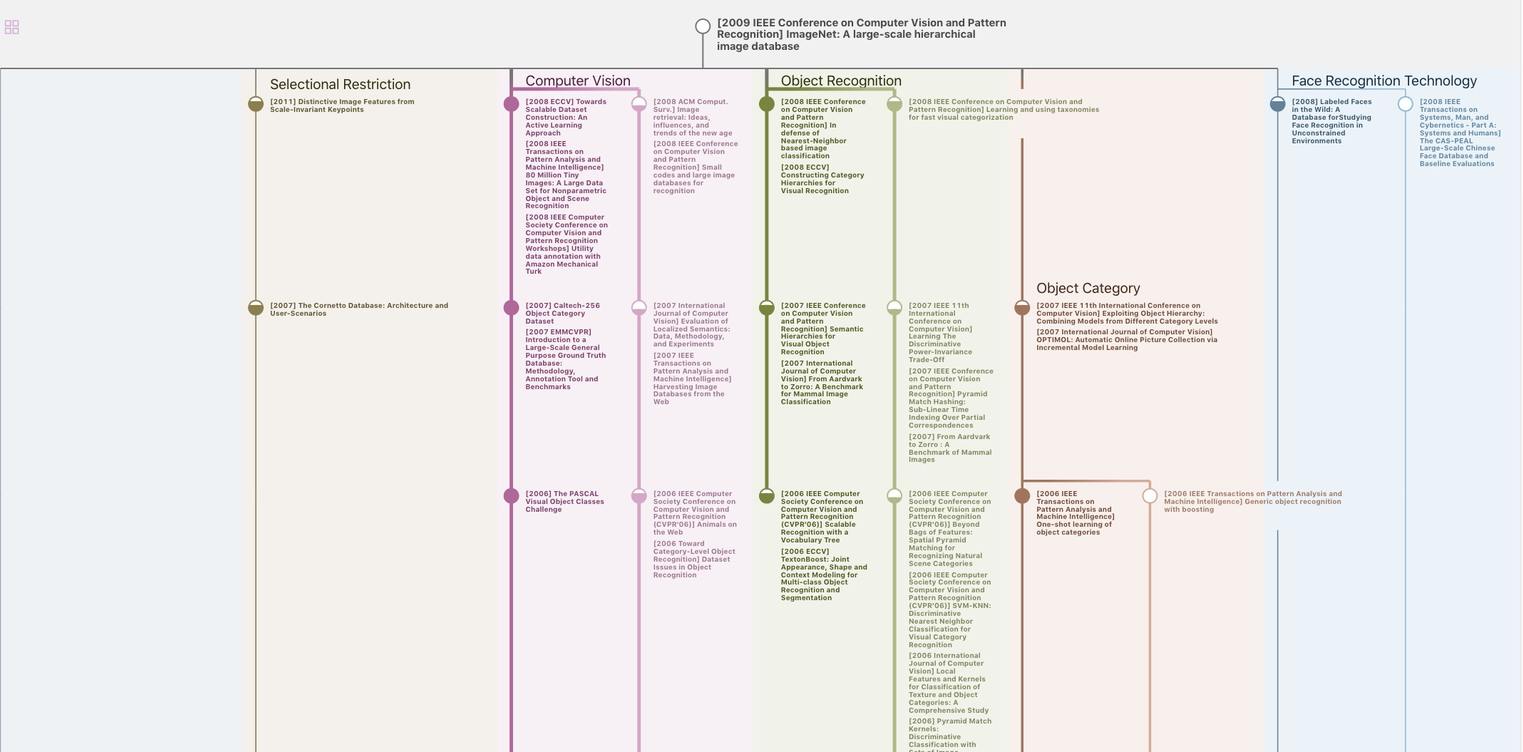
生成溯源树,研究论文发展脉络
Chat Paper
正在生成论文摘要