Multi‐trait genomic predictions using GBLUP and Bayesian mixture prior model in beef cattle
Animal Research and One Health(2023)
Abstract
Abstract Multiple trait genomic selection incorporating correlated traits can improve the predictive ability of low‐heritability traits. In this study, we evaluated genomic prediction accuracy using multi‐trait BayesCπ method (MT‐BayesCπ), which allows for a broader range of mixture priors for important traits in beef cattle. We compared the prediction performance of MT‐BayesCπ with single‐trait genomic best linear unbiased prediction (ST‐GBLUP), multi‐trait GBLUP (MT‐GBLUP), and single‐trait BayeCπ (ST‐BayesCπ) methods. We found that ribeye area (REA) and ribeye weight (REWT) showed high heritability, while slaughter weight (SWT) and carcass weight (CWT) displayed medium heritability, and slaughter rate (SR) and feedlot average daily gain (FDG) showed low heritability. Highly positive genetic correlations were observed between CWT and SWT (0.981) and SR and REWT (0.921). Notably, the MT‐BayesCπ method showed superior predictive abilities compared to other models. Using MT‐BayesCπ method, the accuracy increased from 0.272 to 0.694 for CWT compared to ST‐GBLUP and ST‐BayesCπ. MT‐GBLUP and ST‐BayesCπ showed similar prediction accuracies, while MT‐BayesCπ showed the least biased evaluations. Additionally, our results suggested that prediction accuracy of low‐heritability traits significantly increased when they were combined with traits with high genetic correlation in a multi‐trait prediction. Our study suggests that multi‐trait genomic predictions using GBLUP and Bayesian mixture prior models is feasible for genomic selection in beef cattle. Our findings indicate that MT‐BayesCπ outperforms other models (ST‐GBLUP, MT‐GBLUP and ST‐BayesCπ), especially for low‐heritability traits.
MoreTranslated text
Key words
Bayesian method,beef cattle,GBLUP,multi‐trait genomic predictions
AI Read Science
Must-Reading Tree
Example
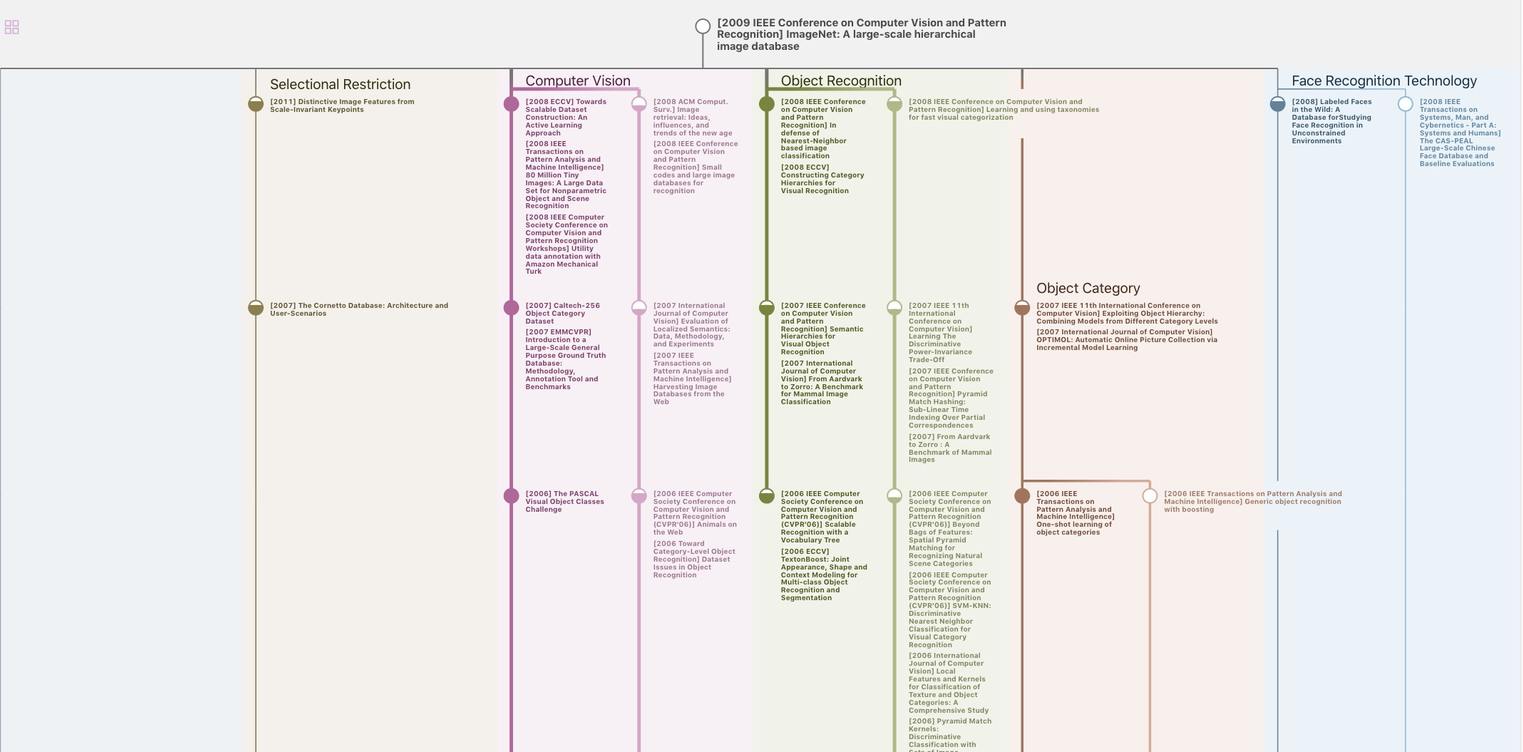
Generate MRT to find the research sequence of this paper
Chat Paper
Summary is being generated by the instructions you defined