Estimation of Extreme Risk Measures for Stochastic Volatility Models with Long Memory and Heavy Tails
Econometrics and Statistics(2023)
摘要
Financial data, such as returns on investments, typically exhibit some non-standard features: long memory or long range dependence (LRD) and heavy tails. Therefore, any mathematical model approximating the evolution of asset price should be able to generate these properties. This can be achieved through the use of a long memory stochastic volatility (LMSV) model. The focus is on estimation of Value-at-Risk (VaR) and Expected Shortfall (ES) for such models. While long memory has no effect on the Hill estimator of the tail index, in contrast it is shown that long memory affects the rates of convergence and asymptotic behaviour of the estimators of VaR and ES.
更多查看译文
关键词
Long Range Dependence,Heavy Tails,Hill Estimator,Stochastic Volatility,Value-at-Risk,Expected Shortfall
AI 理解论文
溯源树
样例
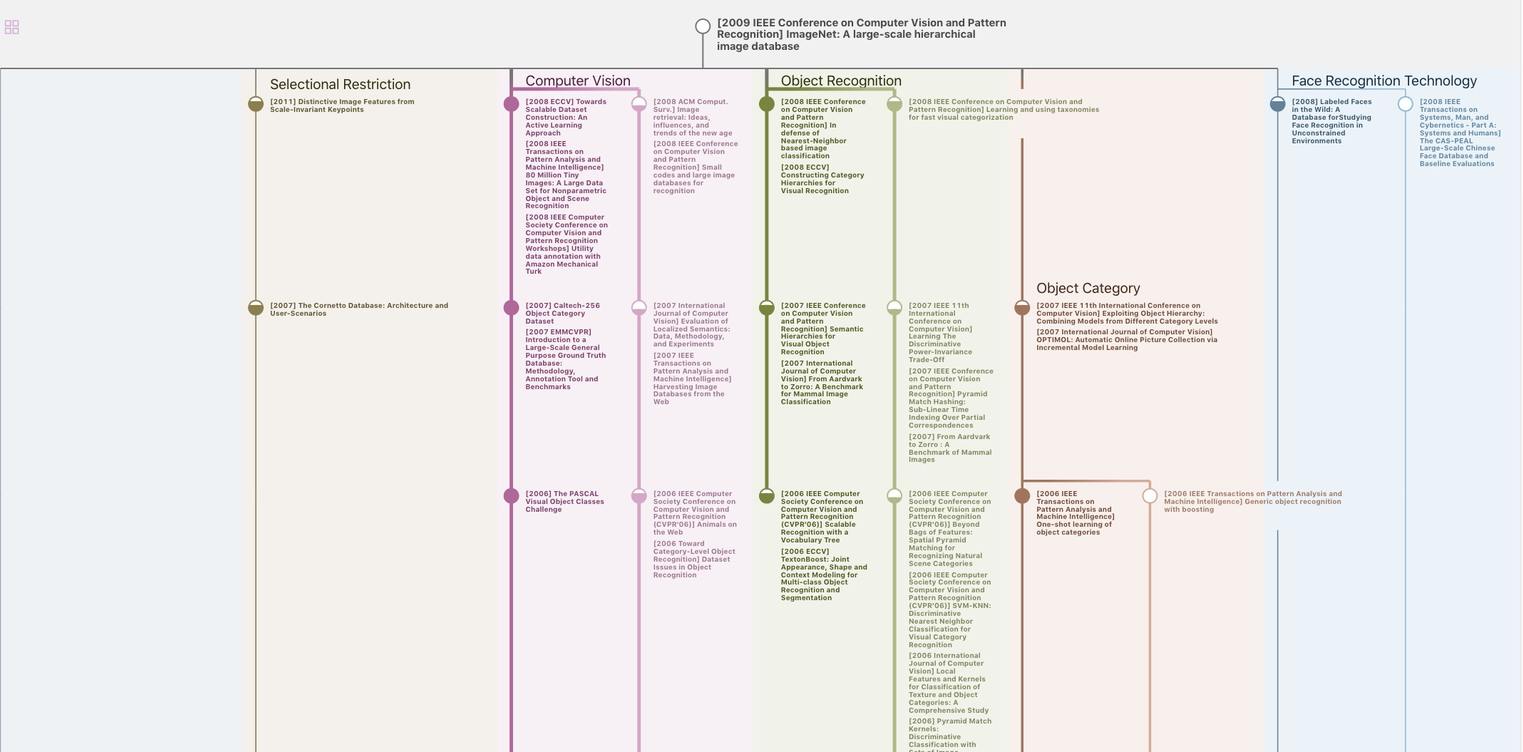
生成溯源树,研究论文发展脉络
Chat Paper
正在生成论文摘要