Distribution Unified and Probability Space Aligned Teacher-Student Learning for Imbalanced Visual Recognition
IEEE TRANSACTIONS ON CIRCUITS AND SYSTEMS FOR VIDEO TECHNOLOGY(2024)
摘要
Imbalanced label distribution is usually the case for real-world data, which poses a challenge for training unbiased recognition model. In this paper, we study two underlying mismatches, i.e., distribution mismatch and probability space mismatch, present in class-imbalanced learning. Firstly, we analyze the label distribution mismatch between imbalanced training data and balanced test data, and introduce a distribution unified framework to unify the two distributions through probability conversion. Secondly, we analyze that the utilization of cross-entropy loss under the proposed framework may lead to probability space mismatch, where the conversion of the predictive probability is implemented in softmax probability space while the comparison with one-hot label is implemented in true probability space. To alleviate this dilemma, we involve a teacher model and formulate a teacher-student learning strategy, which contains two novel techniques. The Teacher Guided Label Smoothing (TGLS) is first proposed to relax the one-hot label to smoother pseudo softmax probability, which is more aligned with the softmax probability space. Additionally, we propose Distribution Unified Knowledge Distillation (DU-KD) under the proposed framework to further reduce both the mismatches. Experiments on several benchmarks confirm the top-level performance of the proposed method.
更多查看译文
关键词
Training,Predictive models,Smoothing methods,Data models,Visualization,Training data,Sun,Class-imbalanced learning,distribution mismatch,probability space mismatch,teacher-student learning
AI 理解论文
溯源树
样例
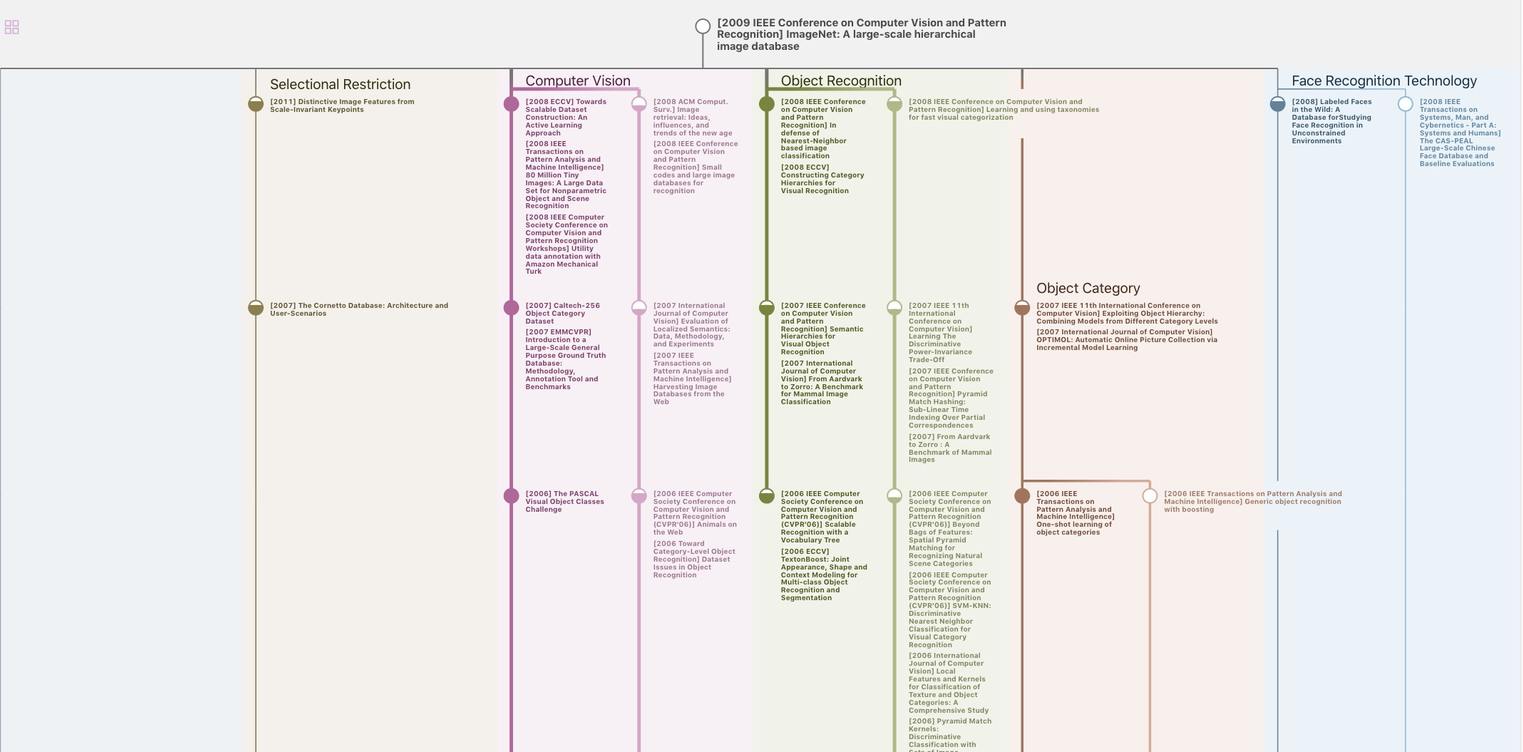
生成溯源树,研究论文发展脉络
Chat Paper
正在生成论文摘要