Deciphering Clusters With a Deterministic Measure of Clustering Tendency.
IEEE Trans. Knowl. Data Eng.(2024)
摘要
Clustering, a key aspect of exploratory data analysis, plays a crucial role in various fields such as information retrieval. Yet, the sheer volume and variety of available clustering algorithms hinder their application to specific tasks, especially given their propensity to enforce partitions, even when no clear clusters exist, often leading to fruitless efforts and erroneous conclusions. This issue highlights the importance of accurately assessing clustering tendencies prior to clustering. However, existing methods either rely on subjective visual assessment, which hinders automation of downstream tasks, or on correlations between subsets of target datasets and random distributions, limiting their practical use. Therefore, we introduce the Proximal Homogeneity Index (PHI) , a novel and deterministic statistic that reliably assesses the clustering tendencies of datasets by analyzing their internal structures via knowledge graphs. Leveraging PHI and the boundaries between clusters, we establish the Partitioning Sensitivity Index (PSI) , a new statistic designed for cluster quality assessment and optimal clustering identification. Comparative studies using twelve synthetic and real-world datasets demonstrate PHI and PSI's superiority over existing metrics for clustering tendency assessment and cluster validation. Furthermore, we demonstrate the scalability of PHI to large and high-dimensional datasets, and PSI's broad effectiveness across diverse cluster analysis tasks.
更多查看译文
关键词
clusters,clustering,deterministic measure
AI 理解论文
溯源树
样例
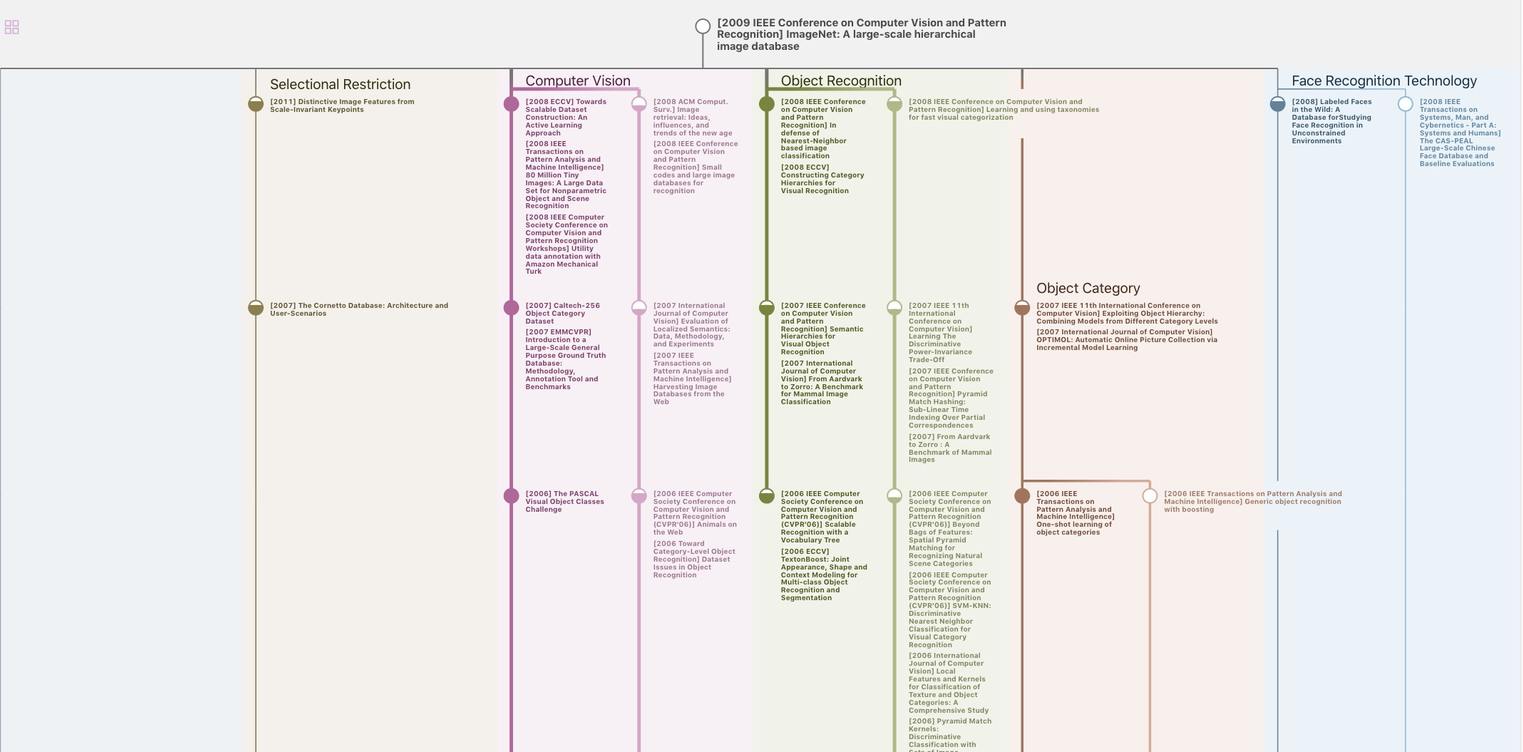
生成溯源树,研究论文发展脉络
Chat Paper
正在生成论文摘要