A Deep Learning Method Integrating Multi-source Data for ECMWF Forcasting Products Correction
IEEE Geoscience and Remote Sensing Letters(2023)
Abstract
Accurate numerical weather prediction is essential for public and commercial meteorological services, but due to the complexity of the meteorological system and the uncertainty of observation data, there may be certain errors in the forecast results.Therefore, this paper proposes a deep learning-based numerical forecast correction network (NFC-Net) that integrates multi-source heterogeneous data from FY-4A satellite, DEM and ERA5.NFC-Net leverages a spatial resolution alignment module and a spatiotemporal feature extraction module to extract and fuse features from diverse data sources, and then applies UNet to correct ECMWF forecast products. The model is evaluated using 2 meters Temperature (2m-T) and 10 meters Wind Speed (10m-WS) data, and compared against Anomaly Numerical-correction with Observations (ANO), Convlstm, and Fuse-CUnet, as well as ERA5 observations. Results demonstrate that NFC-Net outperforms other methods, reducing RMSE of 2m-T and 10 m-WS by 49.71% and 50.86%, respectively, compared to ECMWF forecast products. NFC-Net’s success highlights the importance of effectively integrating and processing heterogeneous data.
MoreTranslated text
Key words
ecmwf forcasting products correction,deep learning,multi-source
AI Read Science
Must-Reading Tree
Example
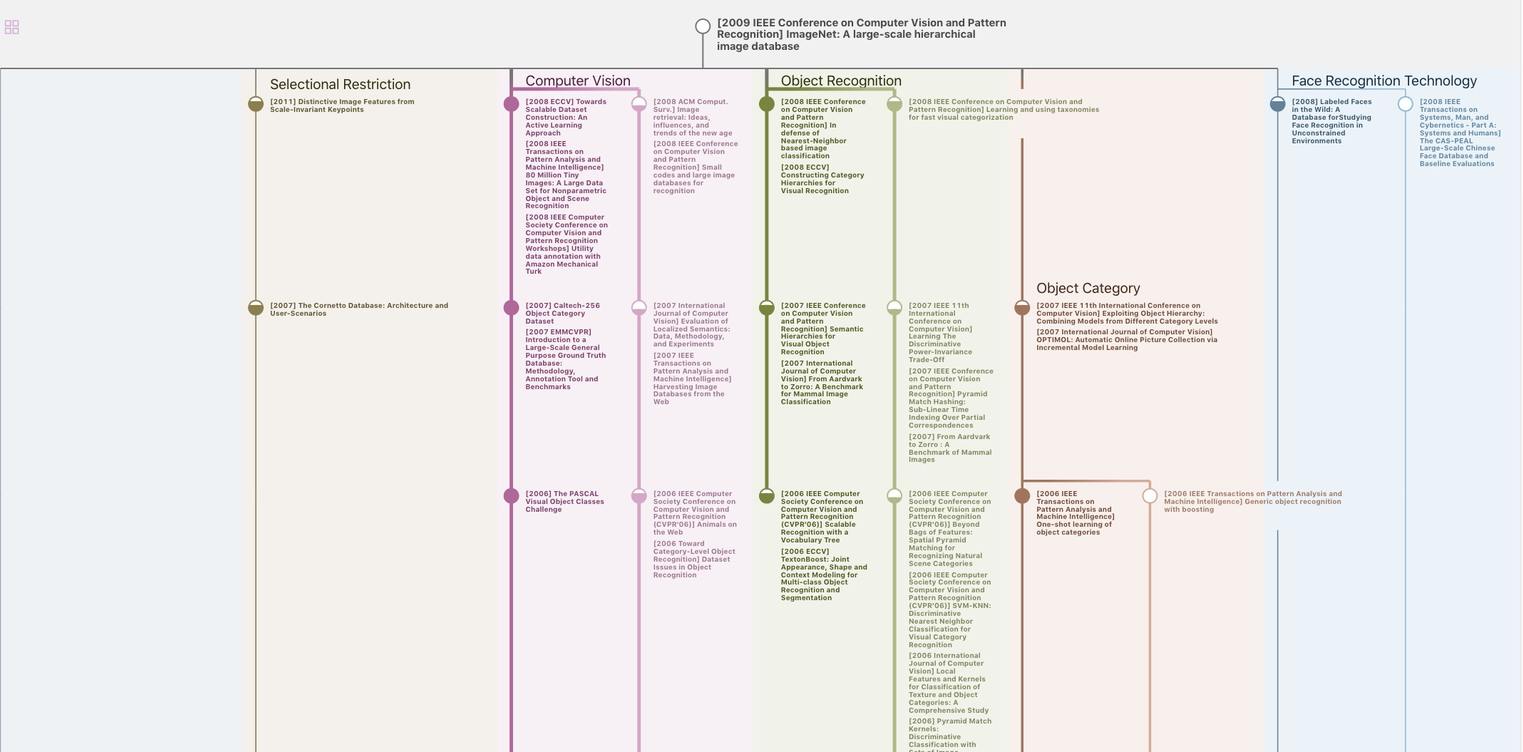
Generate MRT to find the research sequence of this paper
Chat Paper
Summary is being generated by the instructions you defined