Dynamics analysis and image encryption application of Hopfield neural network with a novel multistable and highly tunable memeristor
Research Square (Research Square)(2023)
摘要
Abstract Building neural network models and studying their dynamic behaviours is extremely important from both a theoretical and practical standpoint due to the rapid advancement of artificial intelligence. In addition to its engineering applications, this article concentrates primarily on the memristor model and chaotic dynamics of the asymmetric memristive neural network. First, we develop a novel-multistable, highly-tunable memristor model. Using this memristor model to build an asymmetric memristive neural network (AMNN), the chaotic dynamics of the proposed AMNN are investigated and analyzed using fundamental dynamics techniques such as equilibrium stability, bifurcation diagrams, and Lyapunov exponents. According to the findings of this study, the proposed AMNN possesses a number of complex dynamic properties, including scaling amplitude hyperchaos with coupling strength control, and coexisting unusual chaotic attractors with initial control and coupling strength control. Significantly, the neural networks demonstrate for the first time the phenomenon of infinitely persisting uncommon chaotic attractors. In the interim, a system for image encryption based on the proposed AMNN is constructed utilizing hyperchaotic attractors. By analyzing correlation, information entropy, and key sensitivity, the devised ciphering method reveals a number of benefits. The feasibility of the encryption system is validated through FPGA hardware experiments, and the proposed memristor and AMNN models have been translated into a Simulink model.
更多查看译文
关键词
Chaotic dynamics,Asymmetric memristive Hopfield neural network,Highly tunable memristor,Lyapunov exponents and bifurcation,Image encryption
AI 理解论文
溯源树
样例
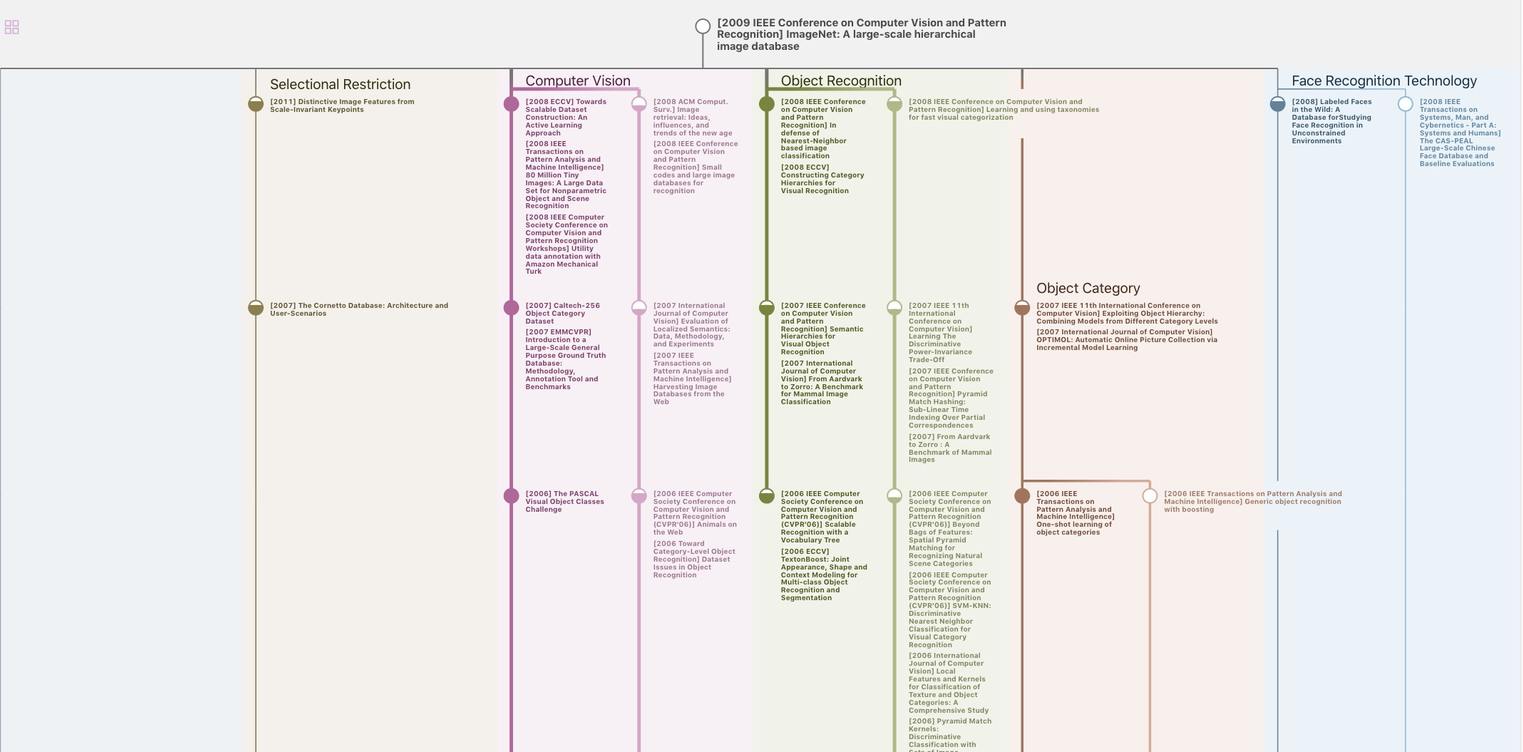
生成溯源树,研究论文发展脉络
Chat Paper
正在生成论文摘要