Joint clustering and missing value imputation for incomplete data via fuzzy modeling and alternate optimization
Research Square (Research Square)(2023)
摘要
Abstract Since missing information is an ordinary phenomenon in actual scenarios that increases the difficulty of data analysis, missing value imputation has attracted ever-growing attention in recent years, by exploiting data modeling. Particularly, missing information in engineering design and optimization is a challenging topic. In this work, an exquisite missing value imputation method based on Takagi-Sugeno (TS) fuzzy modeling is proposed, which first divides incomplete dataset by clustering into several fuzzy subsets and finally establishes global model with different regression models on each subset. Specifically, to improve the clustering performance of practical incomplete issues, we incorporate the measurement of common and uncommon observed feature subspace information of data instances into the fuzzy c-means (FCM) framework. Moreover, to better improve the model accuracy, feature selection is introduced to each fuzzy rule and update model parameters and imputations by co-training. The experiments reported both on UCI and tunnel boring machine (TBM) operation datasets demonstrate the proposed method can accurately accomplish missing value imputation of incomplete data.
更多查看译文
关键词
missing value imputation,incomplete data,fuzzy modeling,joint clustering
AI 理解论文
溯源树
样例
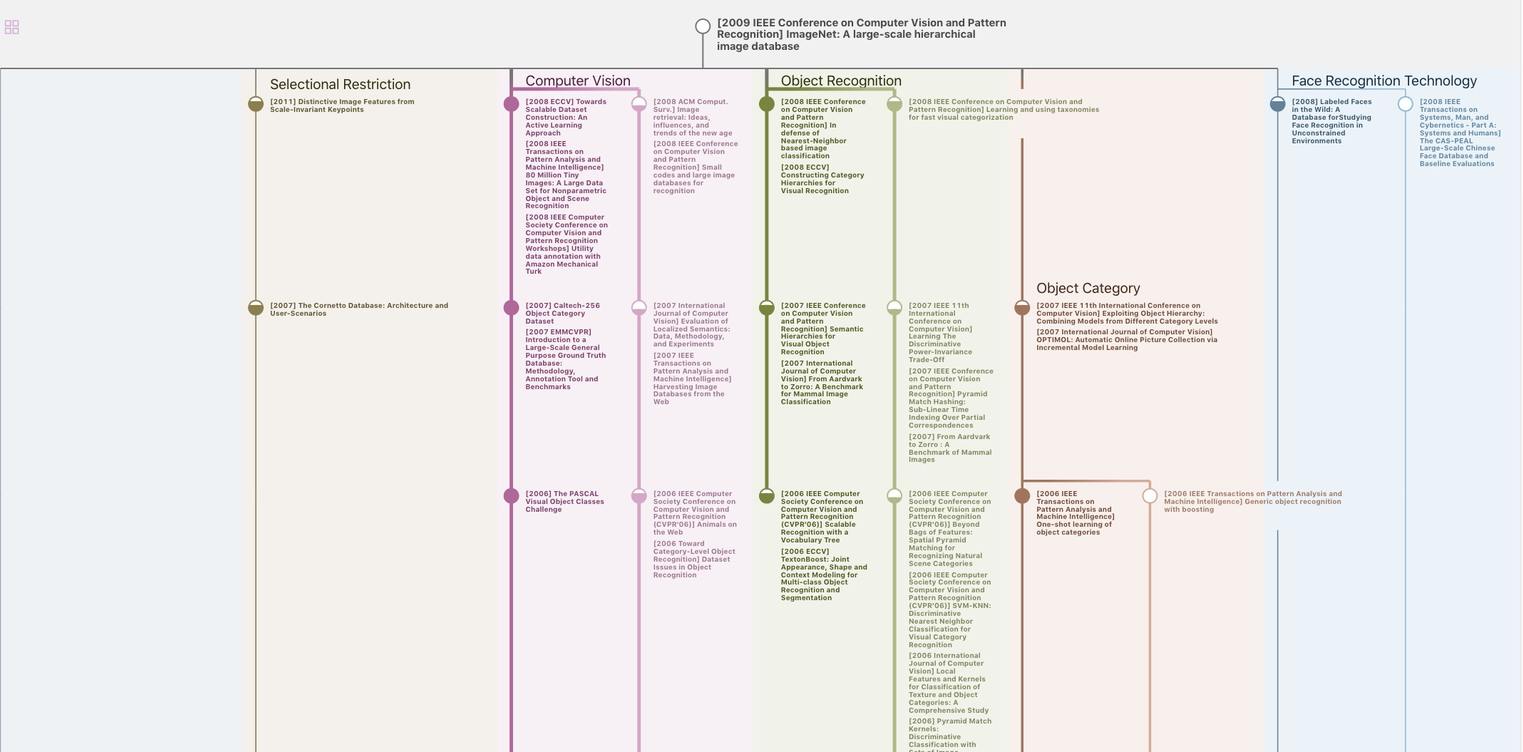
生成溯源树,研究论文发展脉络
Chat Paper
正在生成论文摘要