High-Level Deep Features and Convolutional Neural Network for Evaluating the Classification Performance of File Cluster Types
Lecture notes in networks and systems(2023)
摘要
Accurate file recovery of the corrupted or fragmented file is a very important task when missing the file system, since file recovery procedure involves methods, techniques, and tools that analyze and classify the contents of each data cluster including JPG file or non-JPG file. For classification problems, convolutional neural networks and high-deep learning have been applied to be very efficient and effective in file recovery. This study aims at finding a systematic comparison between a support vector machine (SVM) classifier method and an extreme learning machine (ELM) classifier method for classification issues. The classification methods automatically classify the files in a continuous series of data clusters based on three deep features which are rate of change (RoC), byte frequency distribution (BFD), and entropy. The methods are automatically assigning a class label of JEG file or non-JPG file for fragmented data clusters. The RABEI-2017 dataset was used for evaluating the performance of this study. The results demonstrate that the SVM method outperforms the ELM method with a high accuracy rate to classify fragmented JPG files. The accuracy of classification of the SVM method is 90.15% and of the ELM method is 96.21%.
更多查看译文
关键词
file cluster types,classification performance,high-level
AI 理解论文
溯源树
样例
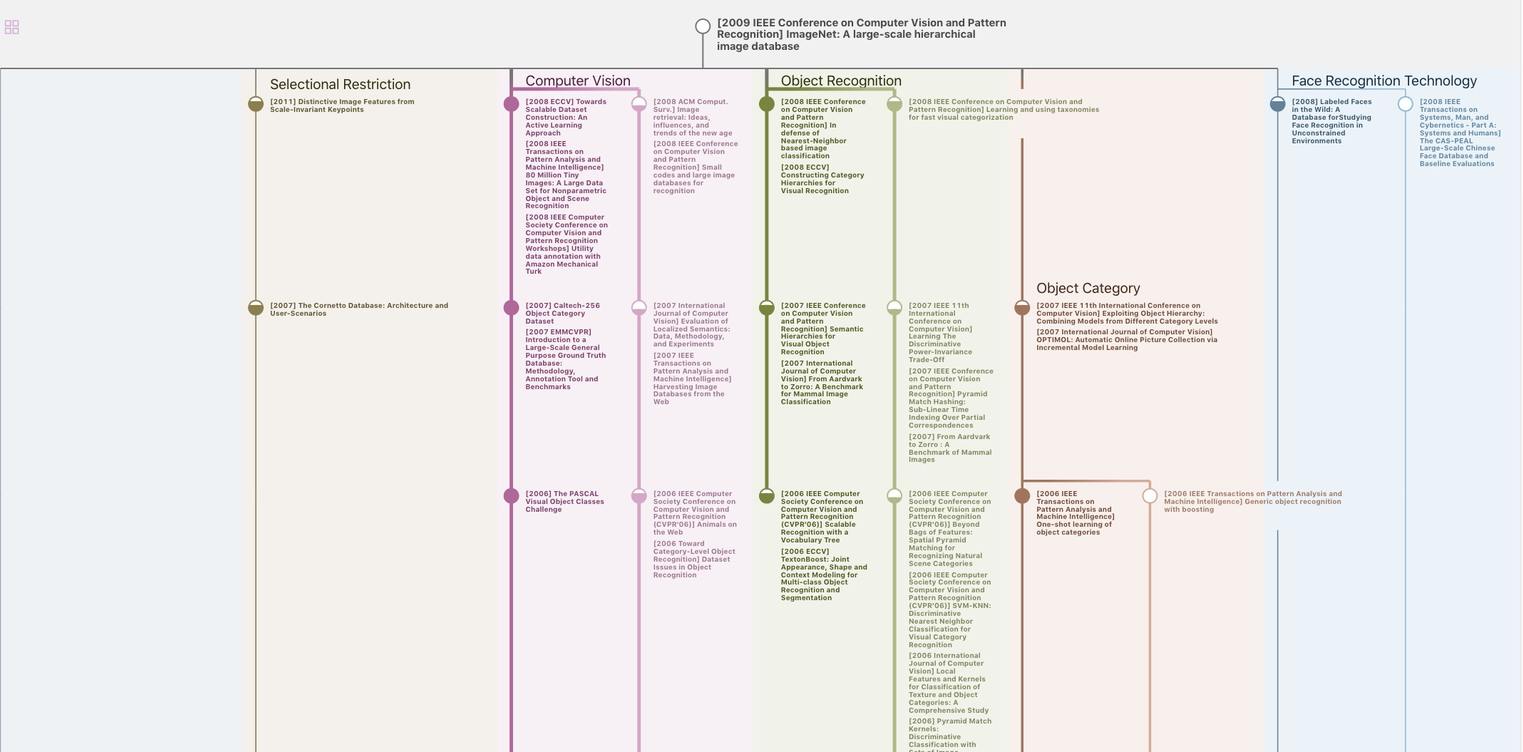
生成溯源树,研究论文发展脉络
Chat Paper
正在生成论文摘要