Statistical Method-Based Multi-Model Ensemble Forecasting for North American Hazardous Air Quality
Authorea (Authorea)(2023)
摘要
Wildfires can cause hazardous air quality conditions and affect human health. Accurate prediction of wildfire air quality is challenging due to uncertainties in fire emission, plume rise, etc. Ensemble forecasting techniques have been increasingly used to improve the predictability of wildfire aerosols. In this study, we aim to explore several ensemble creation methods to improve the ensemble forecast compared to the traditional ensemble mean. Our results show that the ensemble mean outperforms individual models in predicting fine particles (PM2.5) when compared to AirNow observations. To further improve the ensemble forecast, we employ various statistical methods, including multiple linear regression, ridge regression, weighted regression, and quantile regression to develop weighted ensembles. These weighted ensembles are capable of reducing model systematic error, e.g., fractional bias was 25% to 53% lower than the ensemble mean in the major fire regions. Furthermore, the weighted ensemble using ridge regression increased the hit rate by 17% and reduced the false alarm rate by 72% compared to the ensemble mean. This demonstrates that the weighting of the members comprising the ensemble is an effective method for reducing forecast uncertainty and improving the accuracy of air quality forecasting during wildfire events. The quantile and weighted regression methods improved the forecast of extreme air quality events. The PM2.5 exceedance hit rate is improved by 55% compared to the ensemble mean. Our findings provide insights into the development of advanced ensemble methods for wildfire air quality forecast offering support for decision-making to protect public health.
更多查看译文
关键词
ensemble,forecasting,method-based,multi-model
AI 理解论文
溯源树
样例
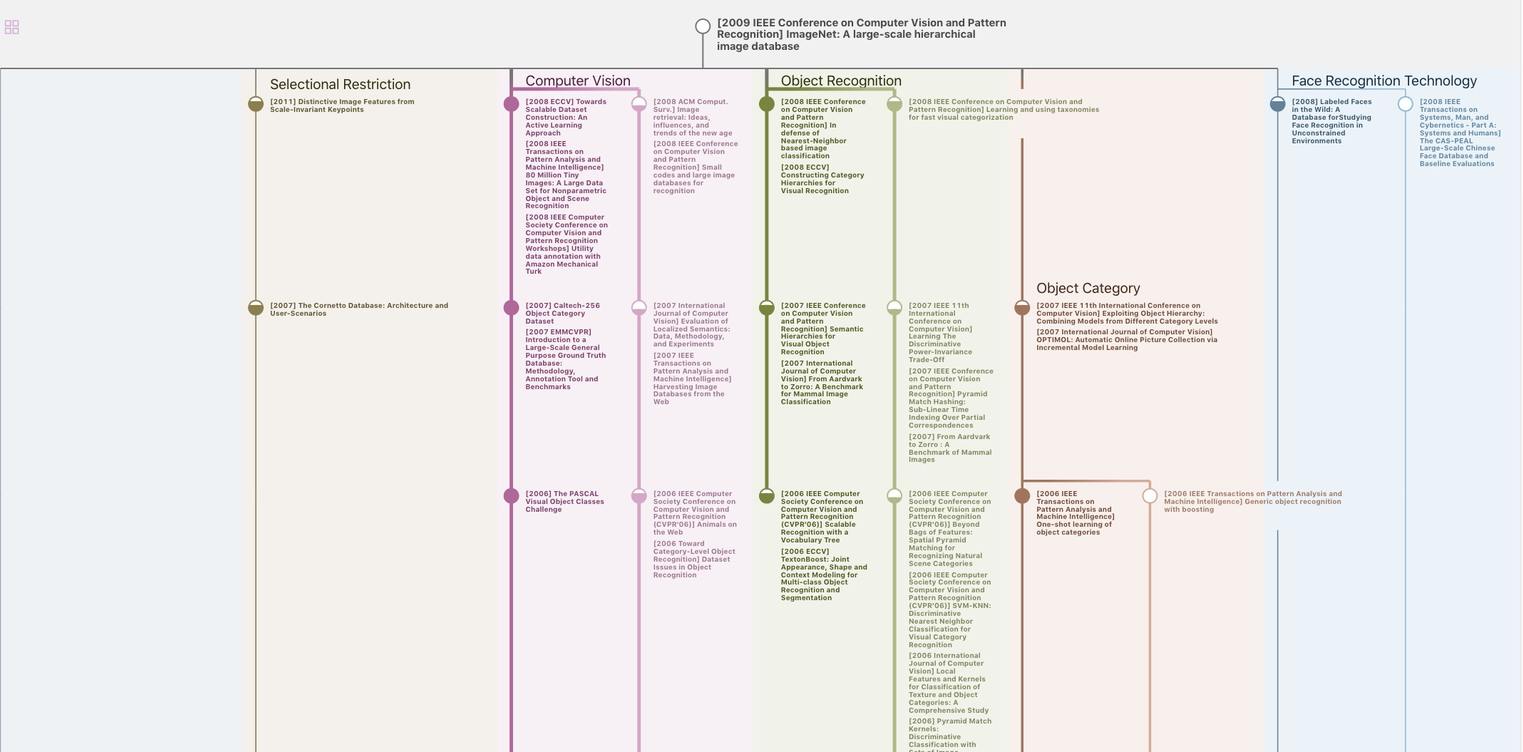
生成溯源树,研究论文发展脉络
Chat Paper
正在生成论文摘要