Dual-level Interaction for Domain Adaptive Semantic Segmentation
arXiv (Cornell University)(2023)
摘要
Self-training approach recently secures its position in domain adaptive semantic segmentation, where a model is trained with target domain pseudo-labels. Current advances have mitigated noisy pseudo-labels resulting from the domain gap. However, they still struggle with erroneous pseudo-labels near the boundaries of the semantic classifier. In this paper, we tackle this issue by proposing a dual-level interaction for domain adaptation (DIDA) in semantic segmentation. Explicitly, we encourage the different augmented views of the same pixel to have not only similar class prediction (semantic-level) but also akin similarity relationship with respect to other pixels (instance-level). As it's impossible to keep features of all pixel instances for a dataset, we, therefore, maintain a labeled instance bank with dynamic updating strategies to selectively store the informative features of instances. Further, DIDA performs cross-level interaction with scattering and gathering techniques to regenerate more reliable pseudo-labels. Our method outperforms the state-of-the-art by a notable margin, especially on confusing and long-tailed classes. Code is available at \href{https://github.com/RainJamesY/DIDA}
更多查看译文
关键词
segmentation,interaction,dual-level
AI 理解论文
溯源树
样例
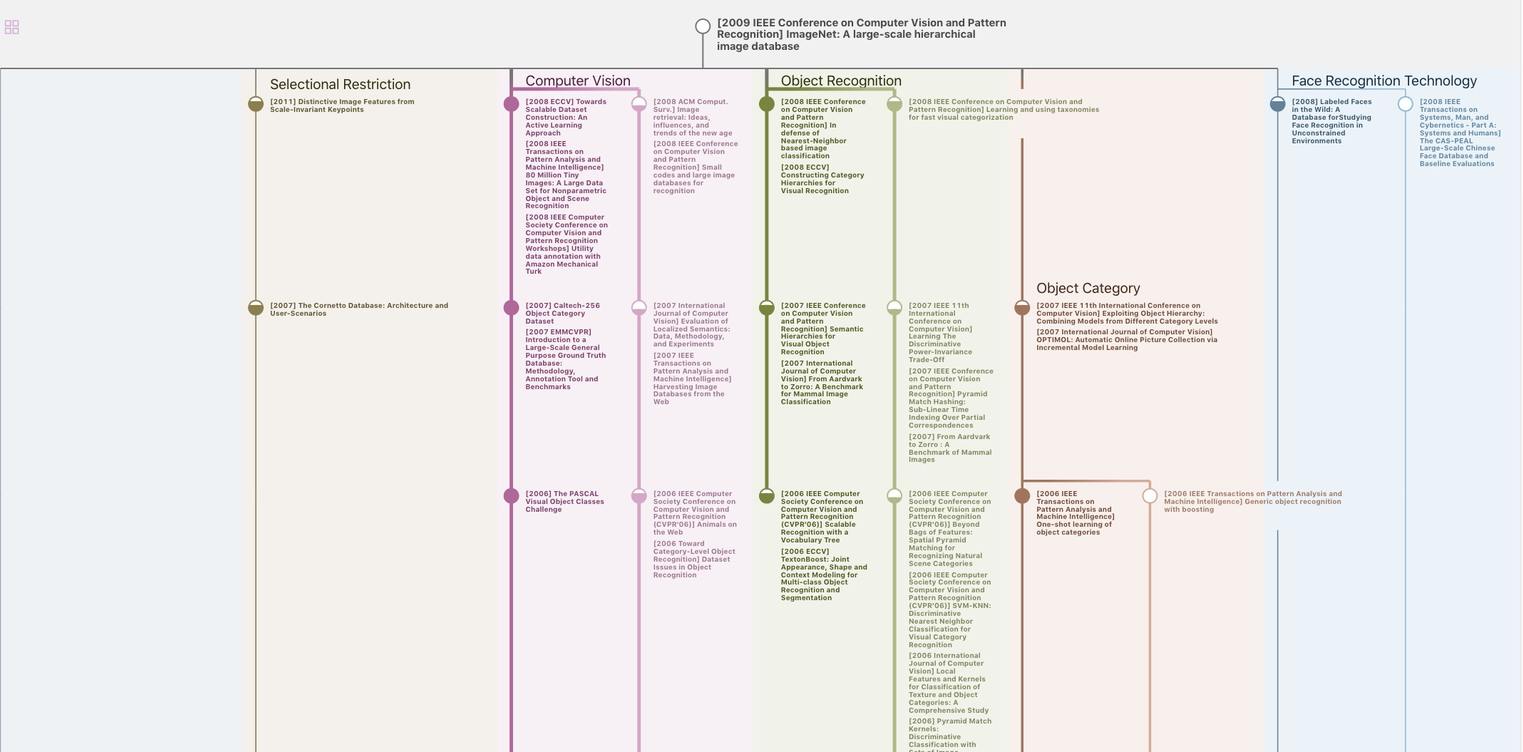
生成溯源树,研究论文发展脉络
Chat Paper
正在生成论文摘要