An interpretable deep learning framework for genome-informed precision oncology
bioRxiv (Cold Spring Harbor Laboratory)(2023)
摘要
Cancers result from aberrations in cellular signaling systems, typically resulting from driver somatic genome alterations (SGAs) in individual tumors. Precision oncology requires understanding the cellular state and selecting medications that induce vulnerability in cancer cells under such conditions. To this end, we developed a computational framework consisting of two components: 1) A representation-learning component, which learns a representation of the cellular signaling systems when perturbed by SGAs, using a biologically-motivated and interpretable deep learning model. 2) A drug-response-prediction component, which predicts the response to drugs by leveraging the information of the cellular state of the cancer cells derived by the first component. Our cell-state-oriented framework significantly enhances the accuracy of genome-informed prediction of drug responses in comparison to models that directly use SGAs as inputs. Importantly, our framework enables the prediction of response to chemotherapy agents based on SGAs, thus expanding genome-informed precision oncology beyond molecularly targeted drugs.
更多查看译文
关键词
precision oncology,interpretable deep learning framework,deep learning,genome-informed
AI 理解论文
溯源树
样例
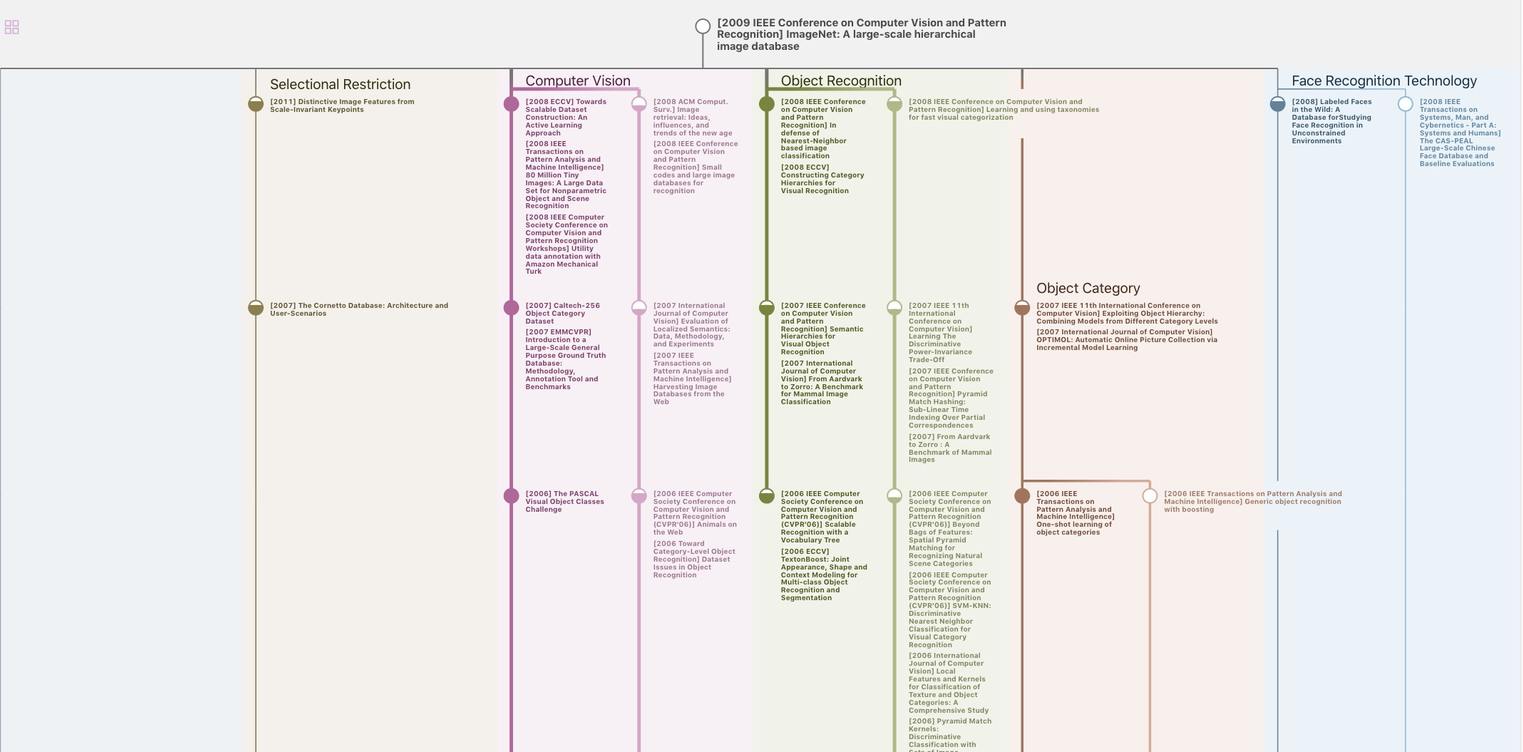
生成溯源树,研究论文发展脉络
Chat Paper
正在生成论文摘要