Direct Superpoints Matching for Robust Point Cloud Registration
arXiv (Cornell University)(2023)
摘要
Deep neural networks endow the downsampled superpoints with highly discriminative feature representations. Previous dominant point cloud registration approaches match these feature representations, \eg, using the Sinkhorn algorithm as the first step. A RANSAC-like method is then usually adopted as a post-processing refinement to filter the outliers. These approaches tend to be computationally intensive due to the iterative nature of RANSAC and require careful parameter tuning to adapt to various practical applications. In this paper, we emphasize the role of matching strategy in superpoint feature matching. We propose a straightforward and effective approach to directly match superpoints by leveraging a global softmax layer in an end-to-end fashion. These matched superpoints are instrumental in estimating the SE(3) transformation between the source and target point clouds. Notably, our approach employs softmax probabilities as weights for each correspondence, allowing us to reject the outliers and further weigh the rest inliers when fitting the transformation matrix, which does not need any post-processing refinement. Moreover, our approach enables joint optimization of different components, including feature representation learning, superpoints matching, and transformation estimation, leading to better registration performance. Experimental results on the standard benchmarks, including ModelNet, 3DMatch, and KITTI validate the effectiveness of our approach, where we obtain comparable or even better accuracy than state-of-the-art methods. Our code and model weights will be publicly available.
更多查看译文
关键词
robust superpoints cloud registration,direct superpoints matching
AI 理解论文
溯源树
样例
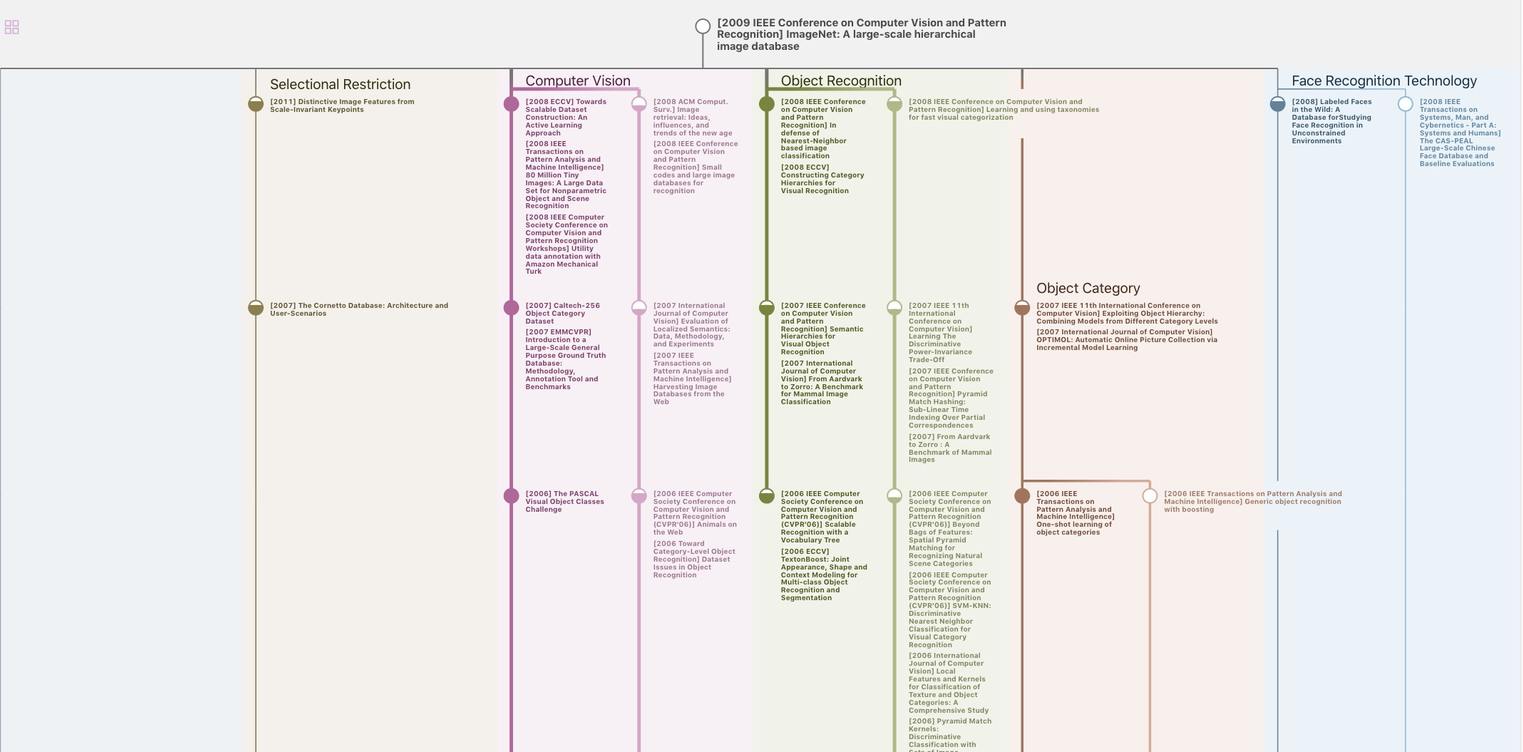
生成溯源树,研究论文发展脉络
Chat Paper
正在生成论文摘要