6-gene machine learning-found signature for the differentiation of pancreatic ductal carcinoma and chronic pancreatitis patients on peripheral blood mononuclear cells RNA-Seq data
Research Square (Research Square)(2023)
摘要
Abstract Background: Pancreatic ductal carcinoma (PDAC) has a poor prognosis given that it is usually found in a late stage. Finding a relevant and informative panel of biomarkers is crucial to diagnose the disease, and therefore, improving patient prognosis.In this work we explore the use of machine learning for finding a biologically relevant signature that can accurately differentiate between PDAC, chronic pancreatitis (CP), and control patients in peripheral blood mononuclear cells (PBMCs) RNA-Seq expression data. Results: Patients data were collected from two different sources: extracellular vesicle long RNA (exLR) and PBMCs RNA-Seq data. The minimum Redundancy Maximum Relevance (mRMR) algorithm was used to find a significant gene signature and a support vector machine (SVM) was trained to classify the classes. We firstly leverage an important dataset of exLR samples using feature selection methodologies to find a 15-gene signature that accurately classifies the classes with an F1-Score of ≈ 80% in both the exLR and the PBMCs datasets. Furthermore, we select six genes from the 15-gene list that have significant biological relevance for PDAC description, and train a machine learning classifier that obtains an F1-Score of 71.0% in a Leave-One-Out CV validation. Conclusion: We propose a relevant signature that can be used to accurately differentiate between the PDAC and CP in a non-invasive and cheap method, which can improve patient prognosis by providing an earlier diagnosis.
更多查看译文
关键词
pancreatic ductal carcinoma,chronic pancreatitis patients,learning-found,rna-seq
AI 理解论文
溯源树
样例
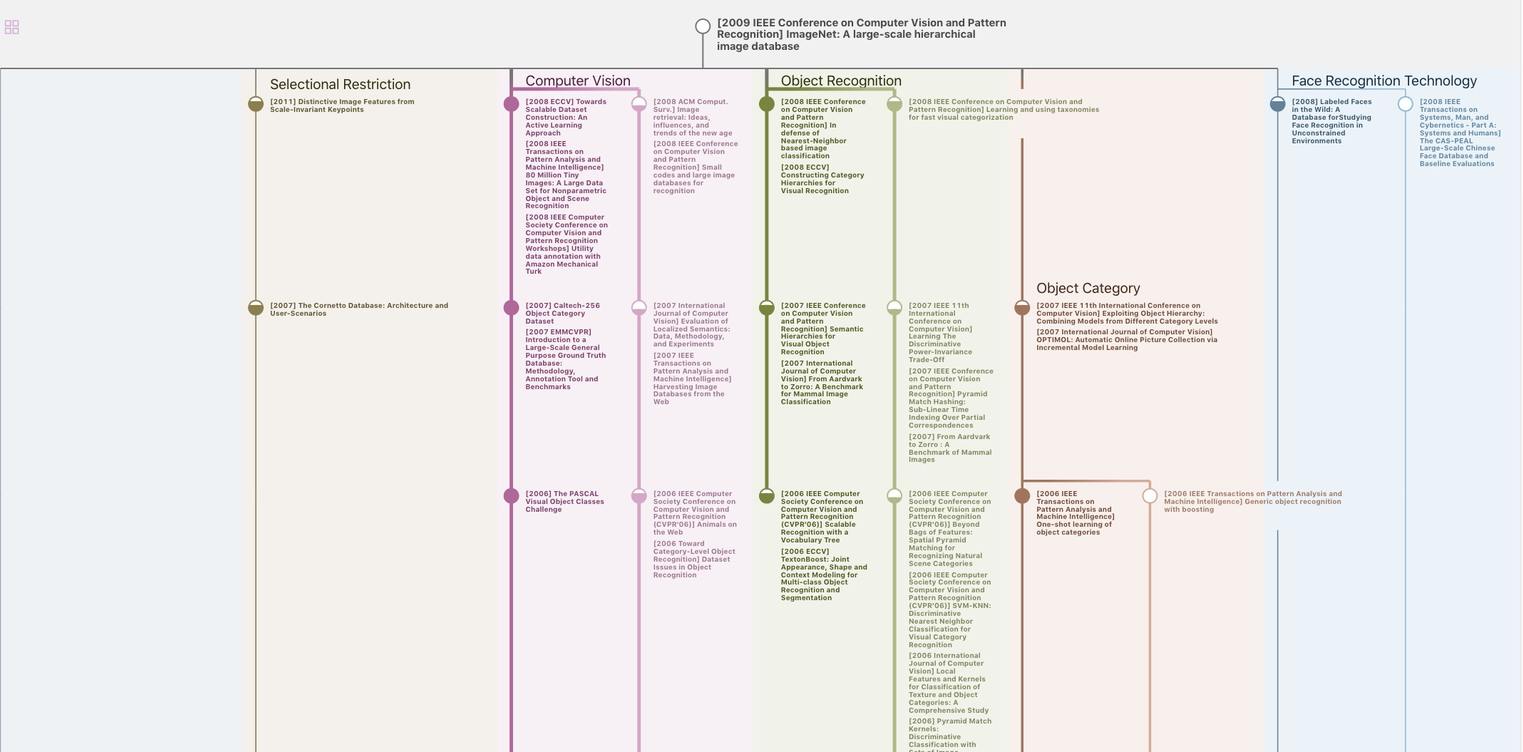
生成溯源树,研究论文发展脉络
Chat Paper
正在生成论文摘要