Robust Roadside Perception for Autonomous Driving: an Annotation-free Strategy with Synthesized Data
arXiv (Cornell University)(2023)
Abstract
Recently, with the rapid development in vehicle-to-infrastructure communication technologies, the infrastructure-based, roadside perception system for cooperative driving has become a rising field. This paper focuses on one of the most critical challenges - the data-insufficiency problem. The lacking of high-quality labeled roadside sensor data with high diversity leads to low robustness, and low transfer-ability of current roadside perception systems. In this paper, a novel approach is proposed to address this problem by creating synthesized training data using Augmented Reality and Generative Adversarial Network. This method creates synthesized dataset that is capable of training or fine-tuning a roadside perception detector which is robust to different weather and lighting conditions, or to adapt a new deployment location. We validate our approach at two intersections: Mcity intersection and State St/Ellsworth Rd roundabout. Our experiments show that (1) the detector can achieve good performance in all conditions when trained on synthesized data only, and (2) the performance of an existing detector trained with labeled data can be enhanced by synthesized data in harsh conditions.
MoreTranslated text
Key words
robust roadside perception,autonomous driving,synthesized data,annotation-free
AI Read Science
Must-Reading Tree
Example
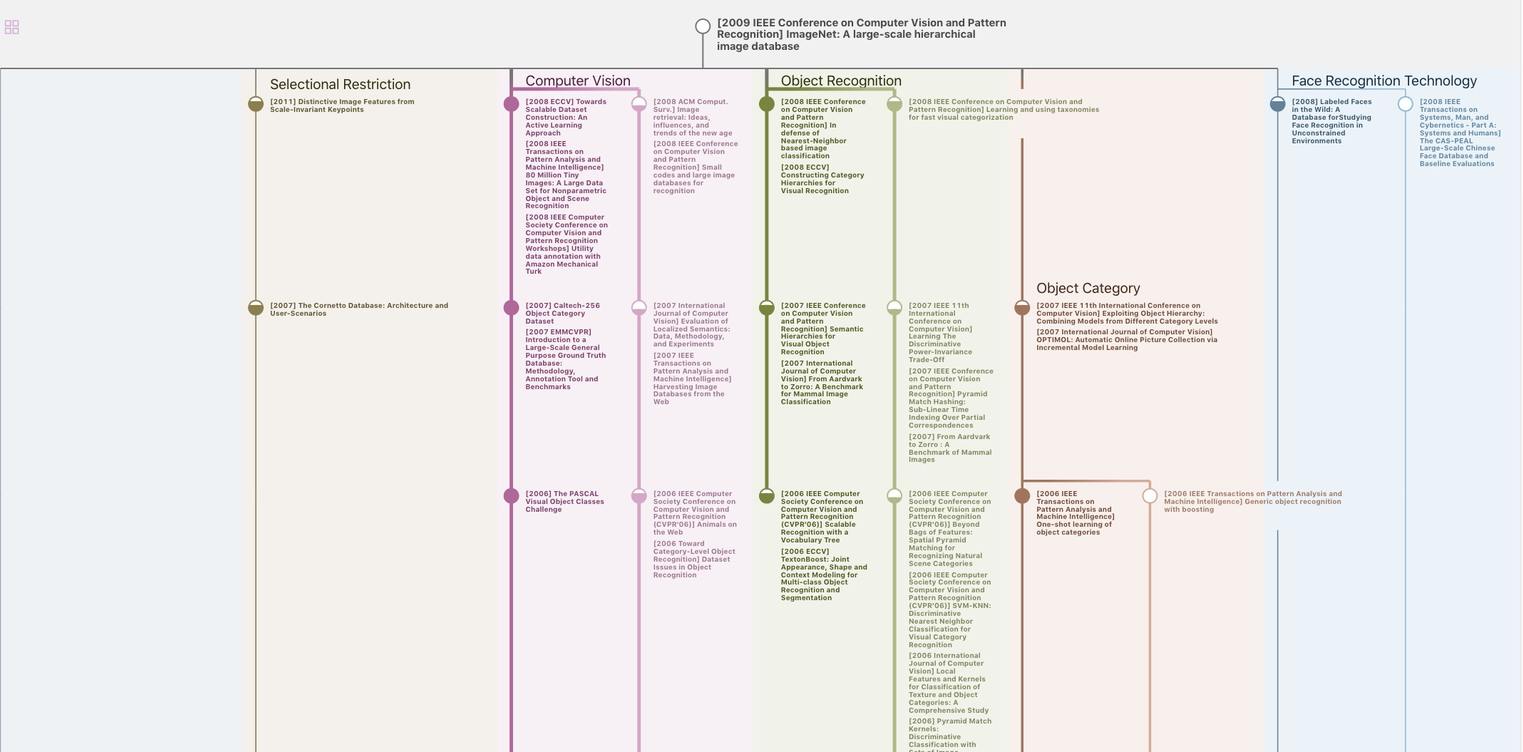
Generate MRT to find the research sequence of this paper
Chat Paper
Summary is being generated by the instructions you defined