Screening and identification of the potential diagnostic genes for Parkinson's Disease based on Machine Learning algorithm
Research Square (Research Square)(2023)
摘要
Background: Parkinson’s disease (PD) is one of the common neurodegenerative diseases, which have an increasingly morbidity rate, and the genetic markers of PD are receiving increasing attention currently. Method: From the Gene Expression Omnibus (GEO) database, we downloaded five array datasets which from the brain tissue of patients with PD and healthy people. We screened the differentially expressed genes (DEGs) between PD and healthy control samples while performed intersection analysis with Weighted Gene Coexpression Network Analysis (WGCNA) genes. Then three machine learning algorithms were employed the least absolute shrinkage and selection operator (LASSO) regression, supported vector machine recursive feature elimination (SVM-RFE) and the Random Forest (RF) analyze to selected the hub gene of PD. The receiver operating characteristic (ROC) curve was used to evaluate the diagnostic efficacy of hub gene. Subsequently, the reverse transcription quantitative polymerase chain reaction (RT-qPCR) experimentwas utilized to confirm the expression of hub gene based on the clinic samples. Finally, the correlations between the expression of hub gene and immune cell ratios were investigated using the CIBERSORT algorithm. Results: Based on the GEO database, 254 overlapping genes were screened from DEG and the blue modules of WGCNA. Functional enrichment analysis showed that these candidate disease genes were associated with PD and might participated in the progression of PD through the MAPK signaling pathway. Two hub genes ( EAF2 and HTR1F ) were subsequently selected through machine learning algorithms, and the ROC analysis showed that the area under curve (AUC) of both genes were all greater than 0.7 in the experimental datasets and validation datasets. Afterwards, the results of the RT-qPCR experiments demonstrated that the mRNA expression of EAF2 and HTR1F were lower in patients with PD, which consistent with the gene expression results in the GEO dataset. Finally, CIBERSORT analysis indicated the changes of the immune environment in PD patients, and revealed the types of immune cells associated with the expression of EAF2 and HTR1F . Conclusion: Two hub genes of PD, EAF2 and HTR1F , were identified by machine learning algorithms, which might provide a significant target for potential diagnosis and personalized treatment of PD in the future.
更多查看译文
关键词
parkinson,machine learning,potential diagnostic genes
AI 理解论文
溯源树
样例
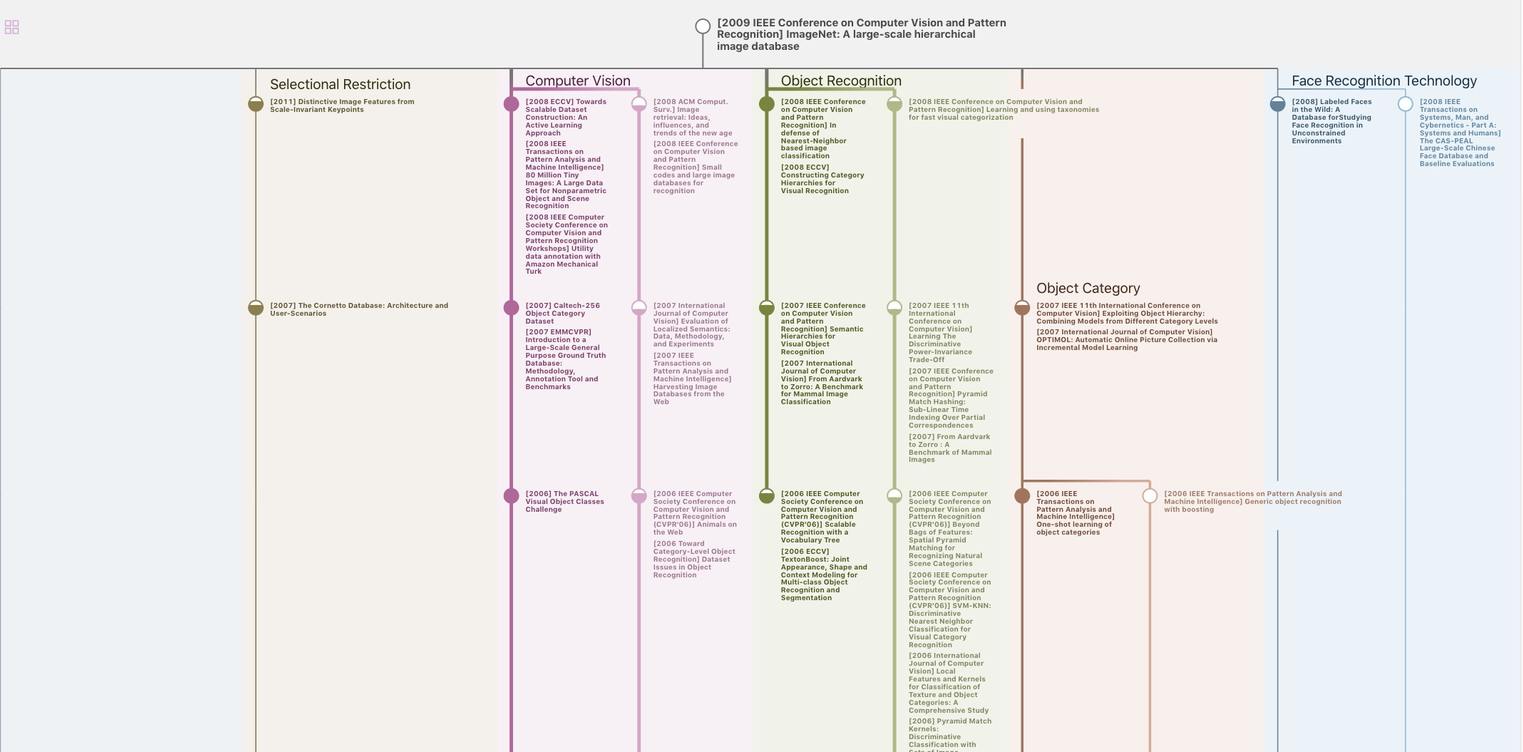
生成溯源树,研究论文发展脉络
Chat Paper
正在生成论文摘要