Shape-based segmentation of retinal layers and fluids in OCT image data.
Medical Imaging: Computer-Aided Diagnosis(2023)
摘要
Optical coherence tomography (OCT) offers non-invasive imaging of the retina and has been well established in the field of ophthalmology for several decades. Based on its high-resolution cross-sectional images, OCT supports diagnosis of various eye diseases. For clinical examination and treatment planning, automated segmentation of individual retinal layers and pathologies is helpful. Retinal layers follow a strict topology that is not addressed by most state-of-the-art methods. While graph-based methods can be used to correct for topology errors, their application is costly and complex, especially in the presence of pathologies. In this work, we propose a segmentation method that uses shape information of retinal layers for improved topology preservation while providing a simple applicability. For this purpose, we use a multi-task framework based on the U-Net architecture that integrates regression of the shape information of the retinal layers in addition to pixel-wise classification. On the one hand, shape-based regression of retinal layers provides spatial regularization and enables the generation of plausible segmentations. On the other hand, the simultaneous classification results in sharper delineations of pathological structures. Furthermore, a task consistency enables semi-supervised training, allowing the use of unlabeled image data for training. A comprehensive evaluation of our segmentation method is performed using OCT image data from patients with diabetic macular edema. Both an ablation study and comparison with other methods have shown the advantages of our proposed segmentation framework. Learning multiple tasks via separate paths leads to higher generalization and prevents overfitting of network parameters. Simultaneously, feature representations preferred by both tasks are learned. Results also show improved preservation of retinal topology while maintaining sharp pathology delineation [1].
更多查看译文
AI 理解论文
溯源树
样例
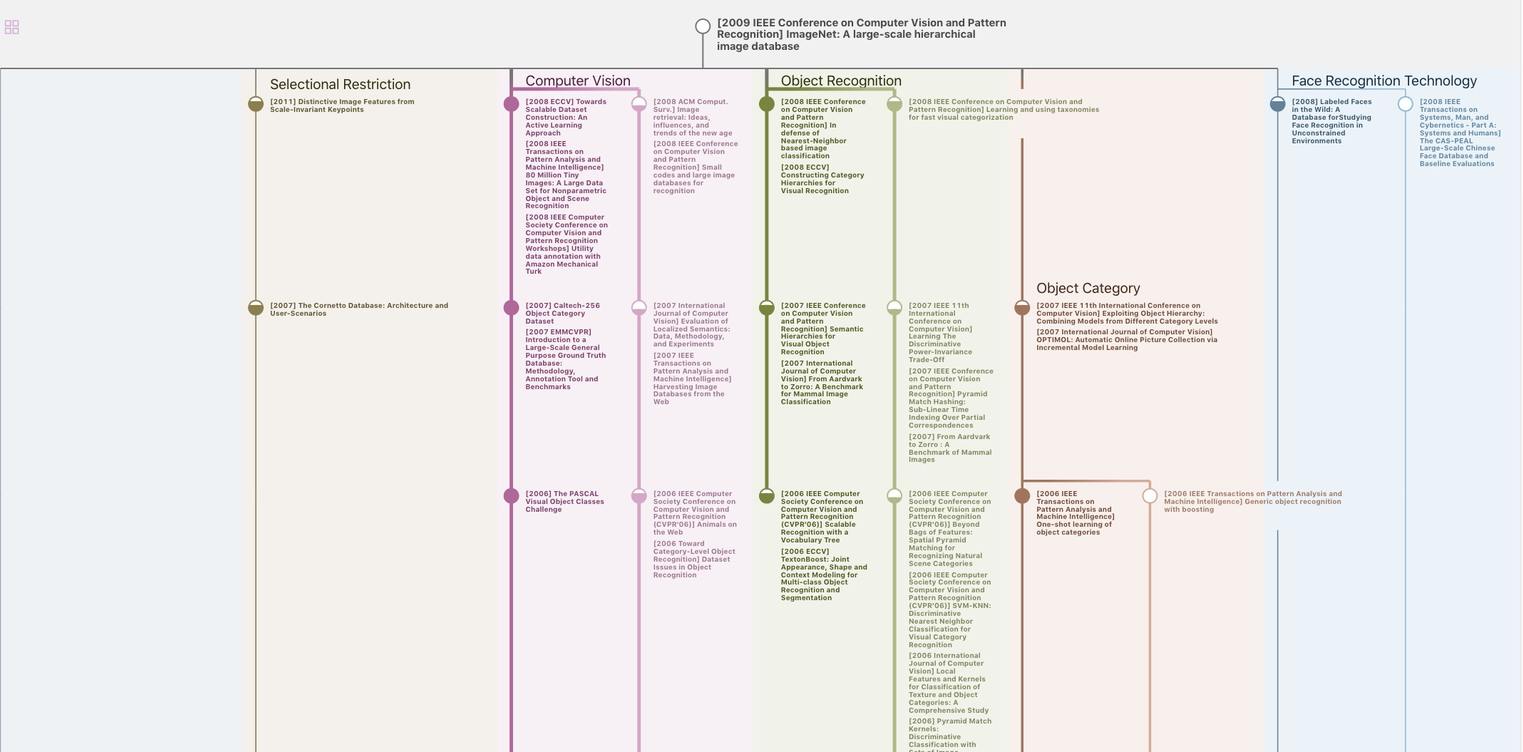
生成溯源树,研究论文发展脉络
Chat Paper
正在生成论文摘要