Prediction of stock prices with automated reinforced learning algorithms
Authorea (Authorea)(2023)
Abstract
The prediction of stock price developments represents one of the greatest challenges in the analysis of time series data and has been investigated for decades using a wide variety of methods. Until now, only a few approaches have been able to establish themselves. The reasons for this vary. One of the challenges in stock price forecasting is that the desired model changes over time due to market dynamics. The objective of this paper is to predict stock prices using automated reinforcement learning algorithms. The models are automated by repeatedly retraining them using data from the recent past. Our approach is designed to capture the market dynamics. DQN models and their modified variants are used as the reinforcing learning algorithms. They have shown remarkable results in recent studies in stock price forecasting. In this paper we were able to show that for different DAX stocks, measured in daily intervals, the test accuracy can improve from 50.00 % to about 60 %. It was shown that for certain step sizes n and window sizes an improvement of the results can be achieved by a dynamic implementation. However, this only holds for stock price data that is measured at daily and weekly intervals.
MoreTranslated text
Key words
stock prices,prediction,learning algorithms
AI Read Science
Must-Reading Tree
Example
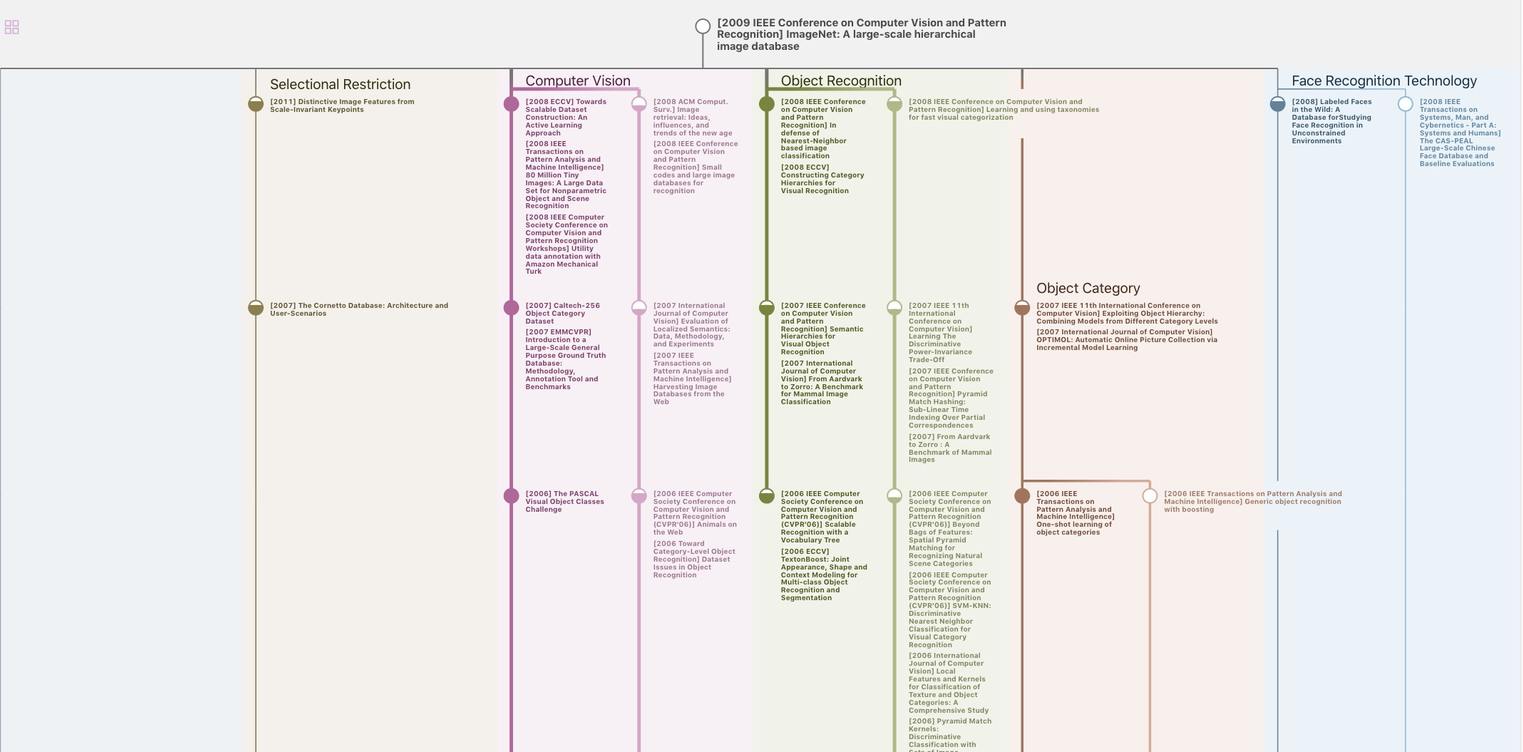
Generate MRT to find the research sequence of this paper
Chat Paper
Summary is being generated by the instructions you defined