One-step TMLE for targeting cause-specific absolute risks and survival curves
Biometrika(2023)
摘要
Summary This paper considers the one-step targeted maximum likelihood estimation methodology for multi-dimensional causal parameters in general survival and competing risk settings where event times take place on the positive real line and are subject to right censoring. We focus on effects of baseline treatment decisions possibly confounded by pretreatment covariates, but remark that our work generalizes to settings with time-varying treatment regimes and time-dependent confounding. We point out two overall contributions of our work. First, our methods can be used to obtain simultaneous inference for treatment effects on multiple absolute risks in competing risk settings. Second, our methods can be used to achieve inference for the full survival curve, or a full absolute risk curve, across time. The one-step targeted maximum likelihood procedure is based on a one-dimensional universal least favourable submodel for each cause-specific hazard that we implement in recursive steps along a corresponding nonuniversal multivariate least favourable submodel. Our empirical study demonstrates the practical use of the methods.
更多查看译文
关键词
survival curves,risks,one-step,cause-specific
AI 理解论文
溯源树
样例
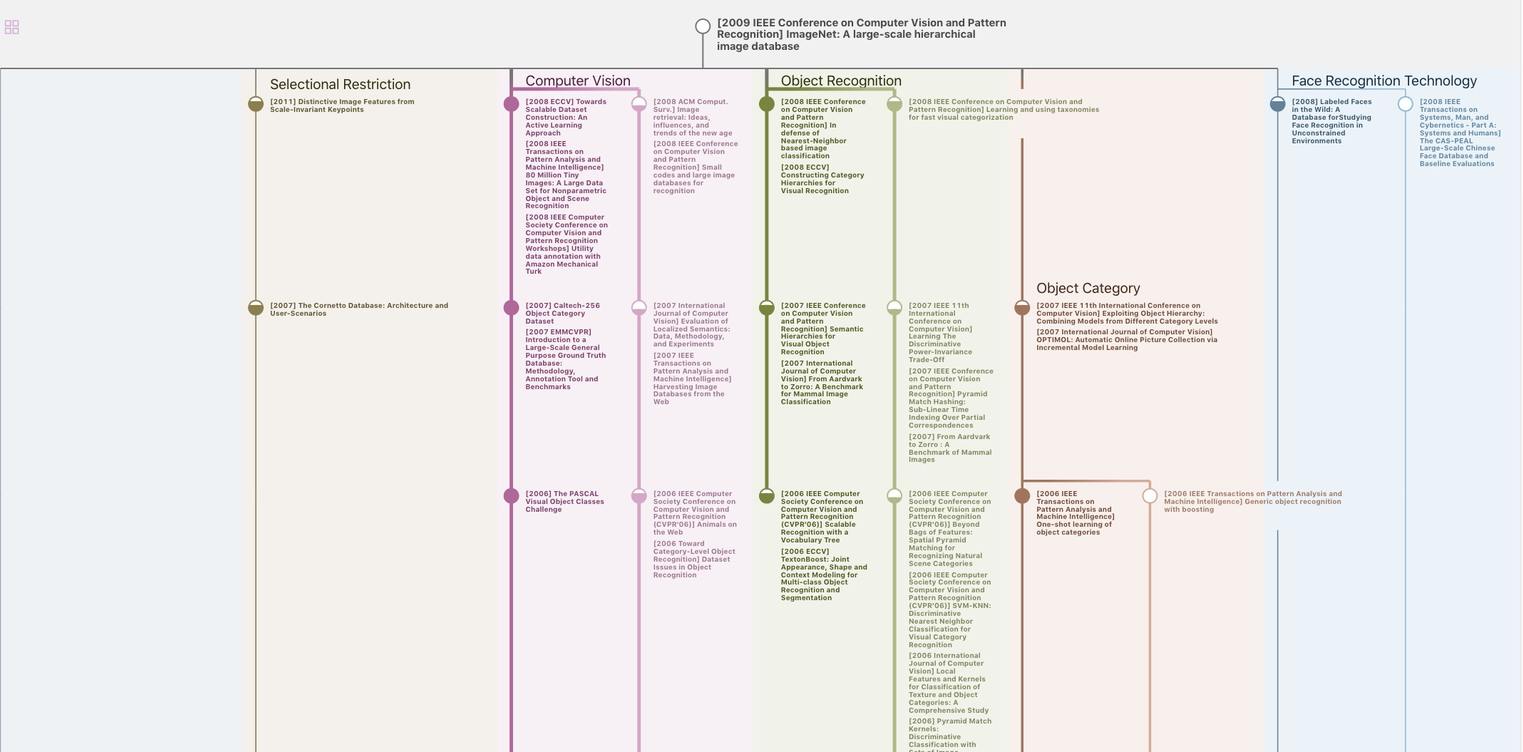
生成溯源树,研究论文发展脉络
Chat Paper
正在生成论文摘要