Robustness Analysis of Deep Reinforcement Learning for Online Portfolio Selection
Oklahoma International Publishing eBooks(2023)
摘要
Online Portfolio Selection (OLPS) requires a careful mix of assets to minimize risk and maximize rewards over a trading episode. The stochastic, non-stationary aspect of the market makes decision-making very complex. Heuristic methods relying on historical returns were traditionally used to select assets that found a balance of risk and reward. However, improvements in modeling time series from Neural Networks led to new solutions. Deep Reinforcement Learning (DRL) has become a popular approach to solve this problem, but its methods rarely reach a consensus among publications. In other fields, solutions using non-Markovian state representations are frequent. Crafting rewards to improve agent learning is common but has effects on the resulting behaviors. The resulting processes are rarely compared to other recent State-of-the-Art solutions but to heuristic algorithms. The proliferation of approaches motivated us to benchmark them using traditional financial metrics, and evaluate their robustness over time and across market conditions. We aim to evaluate the contributions to measured performance from each method in market representation, policy learning and value estimation.
更多查看译文
AI 理解论文
溯源树
样例
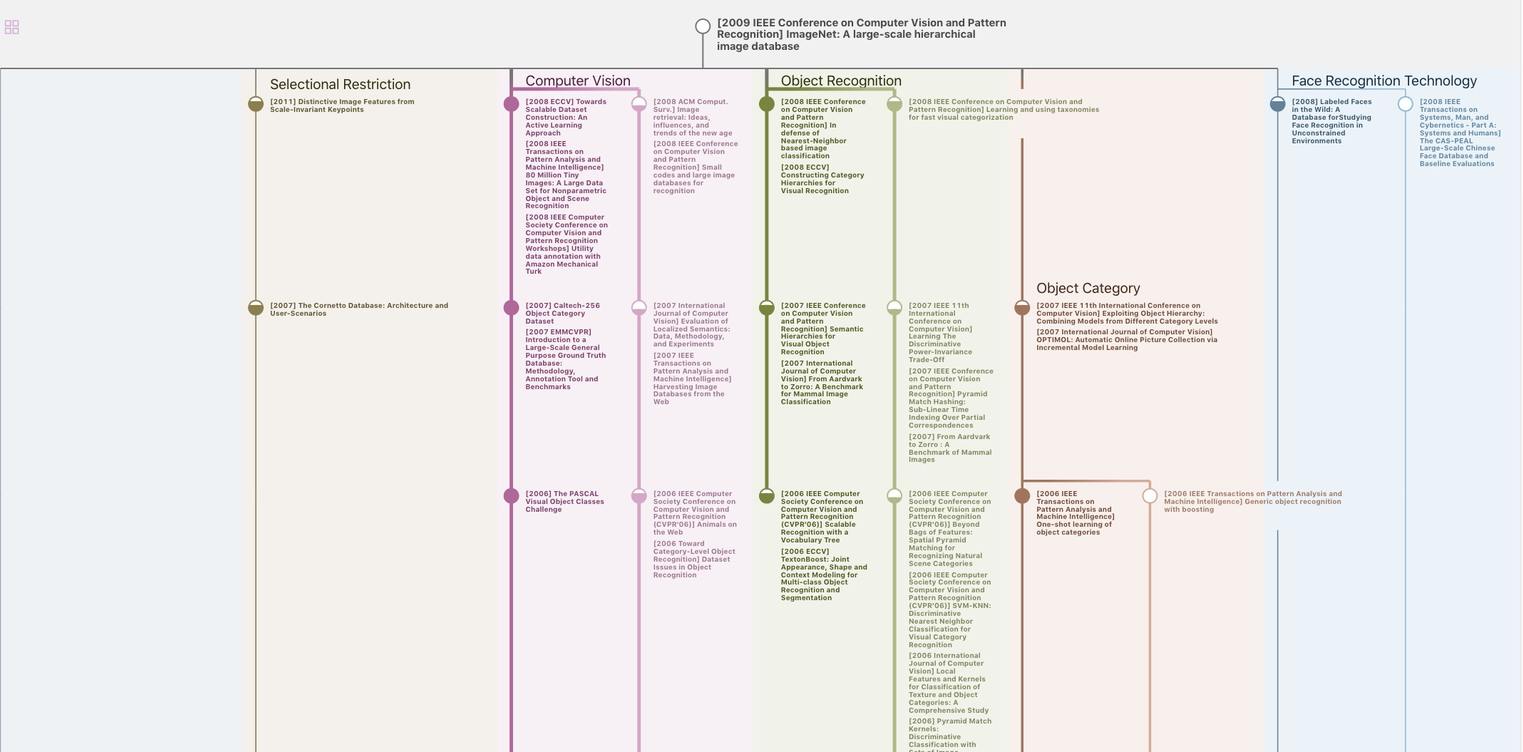
生成溯源树,研究论文发展脉络
Chat Paper
正在生成论文摘要