Insights into deep learning framework for molecular property prediction based on different tokenization algorithms
CHEMICAL ENGINEERING SCIENCE(2024)
摘要
With the rapid development of deep learning, research on quantitative structure-property relationships based on deep learning has received widespread attention. The deep learning architecture combining Bidirectional Encoder Representation from Transformers (BERT) and Feedforward Neural Networks (FNN) is proposed to compare the performance of different tokenization algorithms. And t-distributed stochastic neighbor embedding reveals valuable information about the mechanism of structure-property relationships. Additionally, a deep learning framework, BERT-Convolutional Neural Network (CNN)-FNN, is developed based on the optimal tokenization algorithm to accurately predict the sigma-profile and VCOSMO. The molecular structures are vectorized with the BERT model capturing local and global features of the entire molecule. And the CNN model enhances the latent representation associated with molecular properties, while the FNN model establishes the correlation. The deep learning frameworks predict sigma-profile and VCOSMO properties with R2 greater than 0.9703, making it a promising intelligent tool for guiding solvent design and screening.
更多查看译文
关键词
Deep learning,Molecular latent representation,QSPR,Tokenization algorithm,COSMO-SAC
AI 理解论文
溯源树
样例
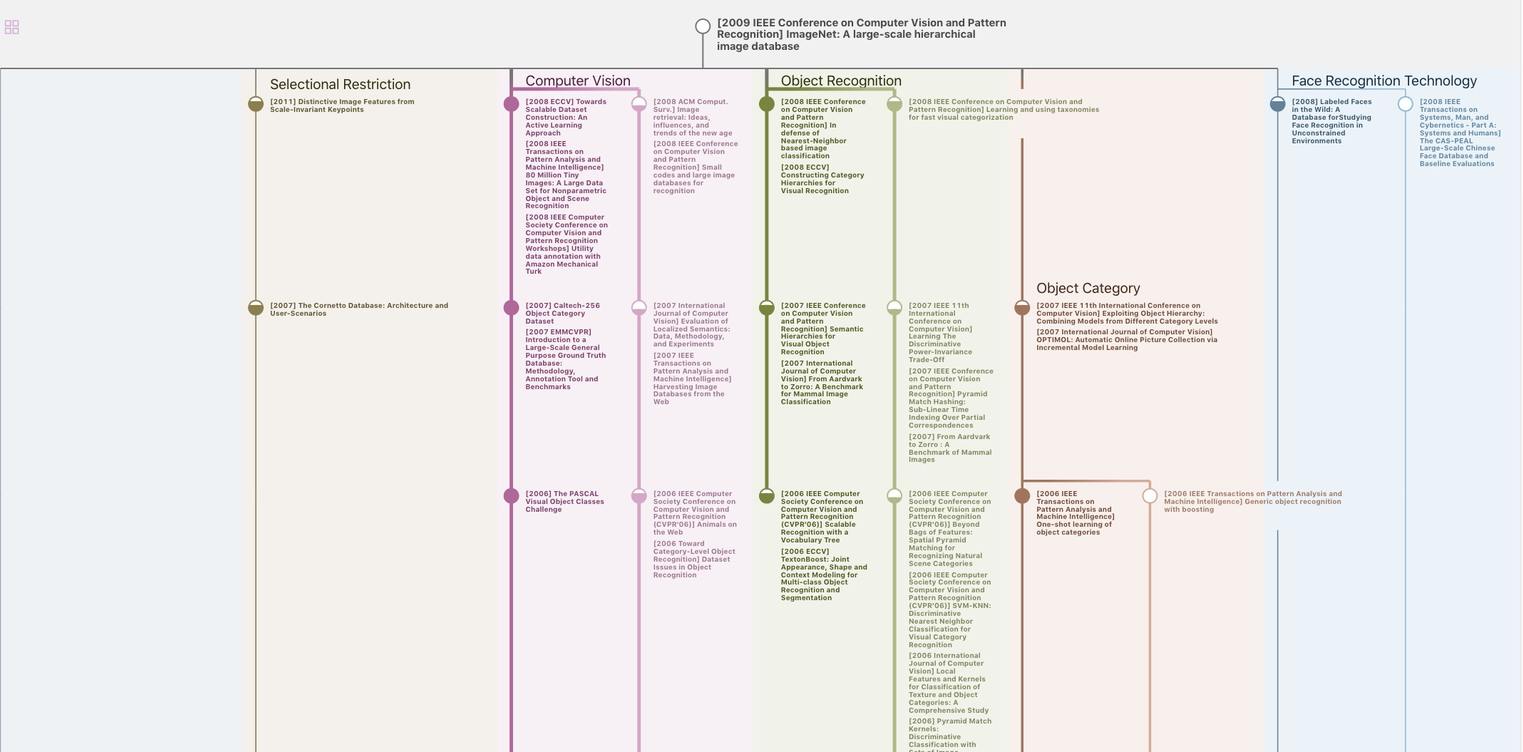
生成溯源树,研究论文发展脉络
Chat Paper
正在生成论文摘要