Semi-supervised, Neural Network based approaches to face mask and anomaly detection in surveillance networks
JOURNAL OF NETWORK AND COMPUTER APPLICATIONS(2024)
摘要
In the post-pandemic world, surveillance cameras play a key aspect when it comes to detecting various kinds of security risks. These can range from burglars entering a premises to an individual wearing or not wearing a mask where convention dictates one way versus the other. We are proposing a system that would allow the autonomous detection of these security risks with minimal human intervention. We propose using Multi-task Cascaded Convolutional Neural Networks as the face detector, followed by a Gabor image feature extractor, and the Kernel-based Online Anomaly Detection algorithm for detecting and identifying potential risks in real-time. We have tested our proposed framework on five different datasets comprising two datasets from public online repositories, one publicly available dataset from the Georgia Institute of Technology, and two from real-life settings. We compared our results to the Viola Jones legacy face detector, and with two other anomaly detection algorithms based on Principal Component Analysis (PCA) and the Autoencoder scheme. Our proposed framework has yielded high detection rates with low false alarm rates, in addition to being adaptive, portable, and requiring minimal infrastructure.
更多查看译文
关键词
Semi-supervised learning,Neural networks,Kernel-based online anomaly detection,Principal component analysis,Autoencoder,Surveillance networks
AI 理解论文
溯源树
样例
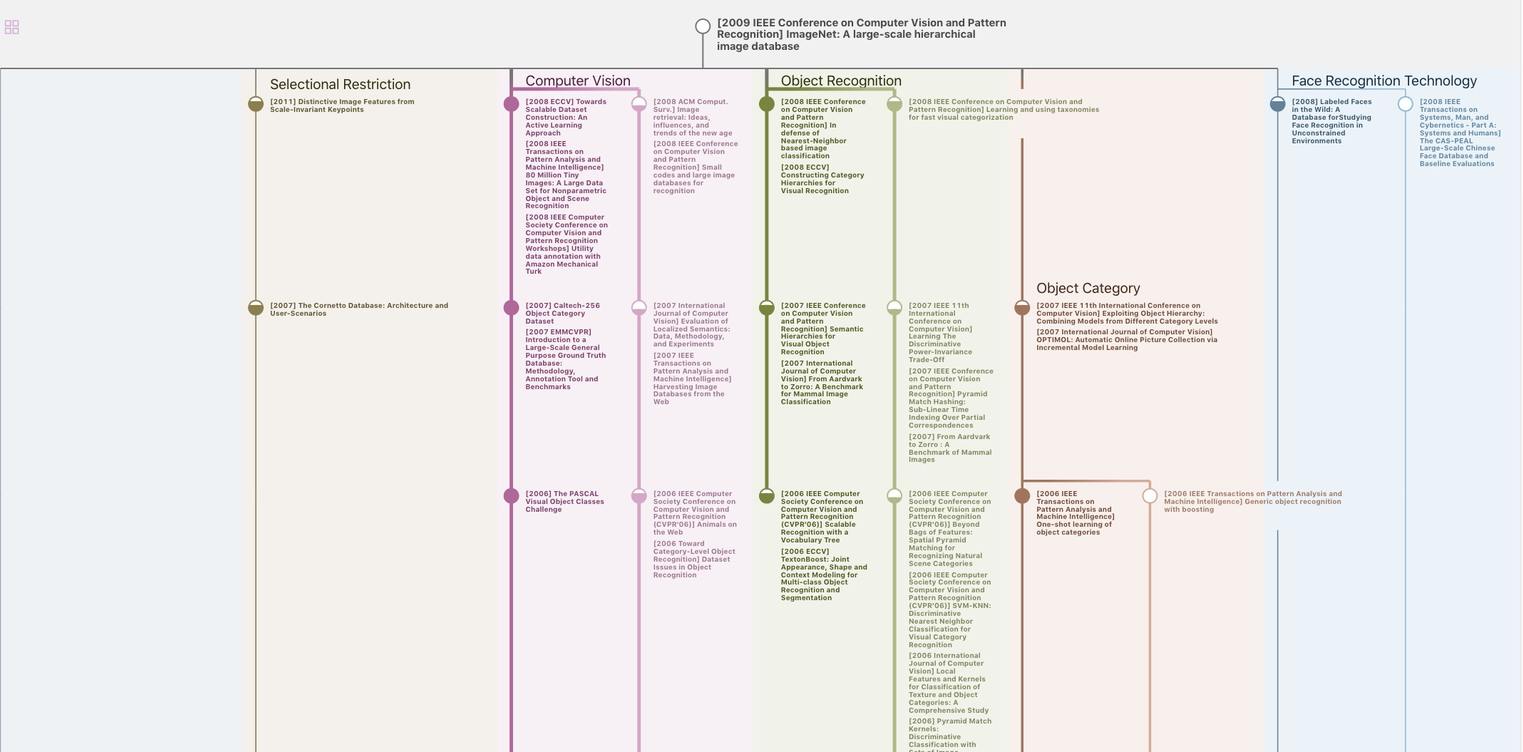
生成溯源树,研究论文发展脉络
Chat Paper
正在生成论文摘要