SIBoLS: Robust and Energy-efficient Learning for Spike-based Machine Intelligence in Information Bottleneck Framework
IEEE Transactions on Cognitive and Developmental Systems(2023)
摘要
Spike-based machine intelligence has recently attracted increasing research attention, and has been considered as a promising approach towards artificial general intelligence (AGI). It has been applied in energy-efficient neuromorphic computing systems. One of the most critical questions for spike-based learning is how to leverage powerful and promising information-theoretic learning theories to derive learning algorithms for improving the robustness and energy efficiency of spiking neural networks (SNNs). In this study, we first present an efficient and effective information bottleneck (IB) framework for training SNN, named Spike-based Information Bottleneck with Learnable State (SIBoLS). We thoroughly explore the design space concerning IB framework by using the membrane potential state for the hidden information representation with learnable hidden variable. Comprehensive robustness test is conducted, in which two types of neuromorphic background noise and five types of input noise are considered. It shows SIBoLS can improve the learning robustness for both static image and event-based dataset of neuromorphic processor. Furthermore, SIBoLS induces less spiking rate, resulting in lower power consumption compared to other techniques. SIBoLS has advantages in terms of robustness and energy efficiency for spike-based machine learning applications, which can give insights for the development of AGI.
更多查看译文
关键词
spiking neural network (SNN),artificial general intelligence,information bottleneck,robust learning,information theoretic learning (ITL)
AI 理解论文
溯源树
样例
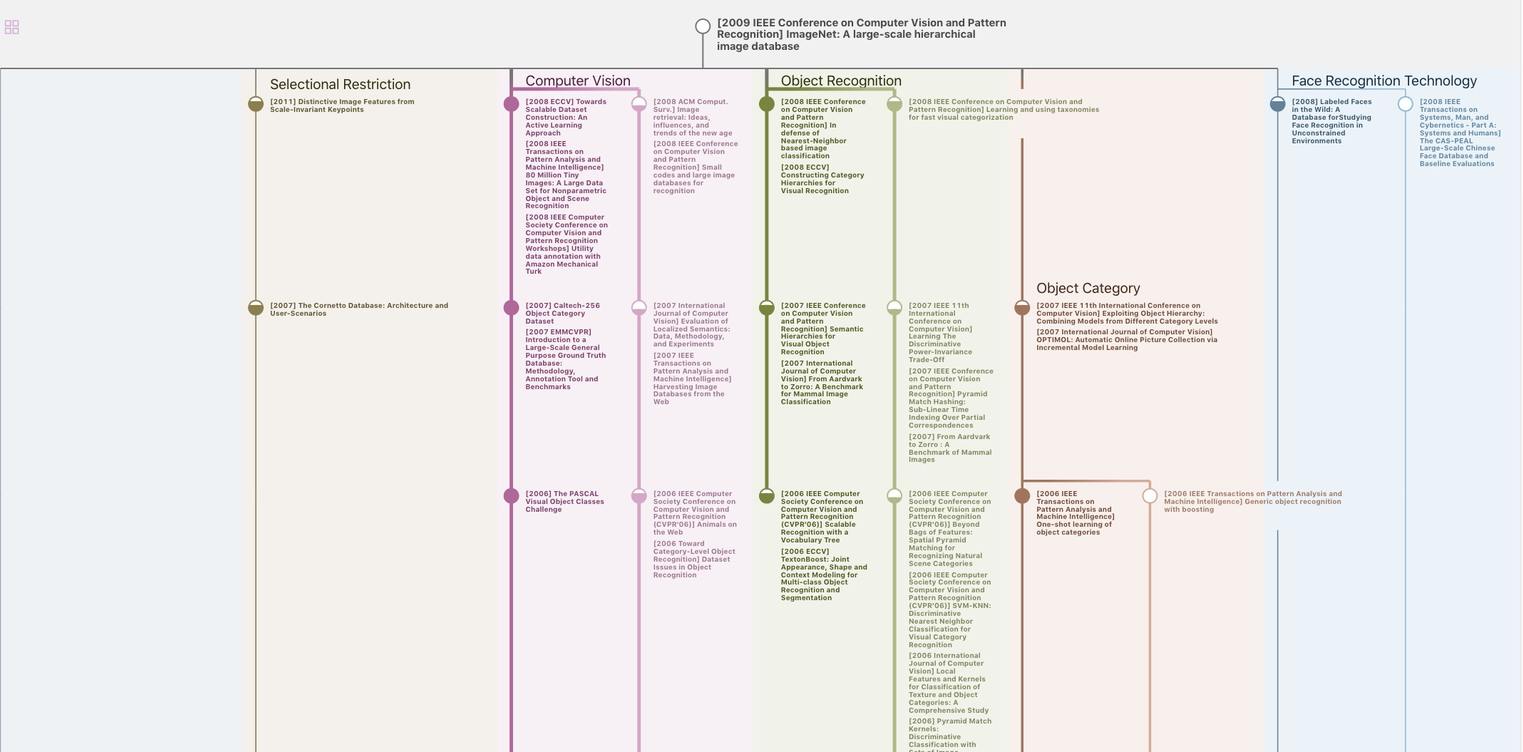
生成溯源树,研究论文发展脉络
Chat Paper
正在生成论文摘要