Bootstrap-based Layer-wise Refining for Causal Structure Learning
IEEE transactions on artificial intelligence(2023)
摘要
Learning causal structures from observational data is critical for causal discovery and many machine learning tasks. Traditional constraint-based methods first adopt conditional independence (CI) tests to learn a global skeleton layer by layer and then orient the undirected edges to obtain a causal structure. However, the reliability of these statistical tests largely depends on the quality of data samples. In real-life scenarios, the presence of data noise or limited samples often makes many CI tests unreliable at each layer in the skeleton learning phase, leading to an inaccurate skeleton. As the number of layers increases, the inaccurate skeleton will continue to impair the skeleton construction of subsequent layers. Furthermore, an unreliable skeleton hampers the skeleton orientation procedure, resulting in an unsatisfactory causal structure. In this paper, we propose a Bootstrap-based Layer-wise Refining (BLR) algorithm for causal structure learning, which includes two new procedures to solve the above problems. First, BLR utilizes a novel layer-wise skeleton refining procedure to construct the global skeleton layer by layer based on the bootstrap sampling. Second, BLR employs a collective skeleton orientation procedure that incorporates scoring techniques to collectively orient the global skeleton. The experimental results show that BLR outperforms the state-ofthe-art methods on the benchmark Bayesian Network datasets.
更多查看译文
关键词
Causal structure learning,Directed acyclic graph,Layer-wise refining,Bootstrap sampling
AI 理解论文
溯源树
样例
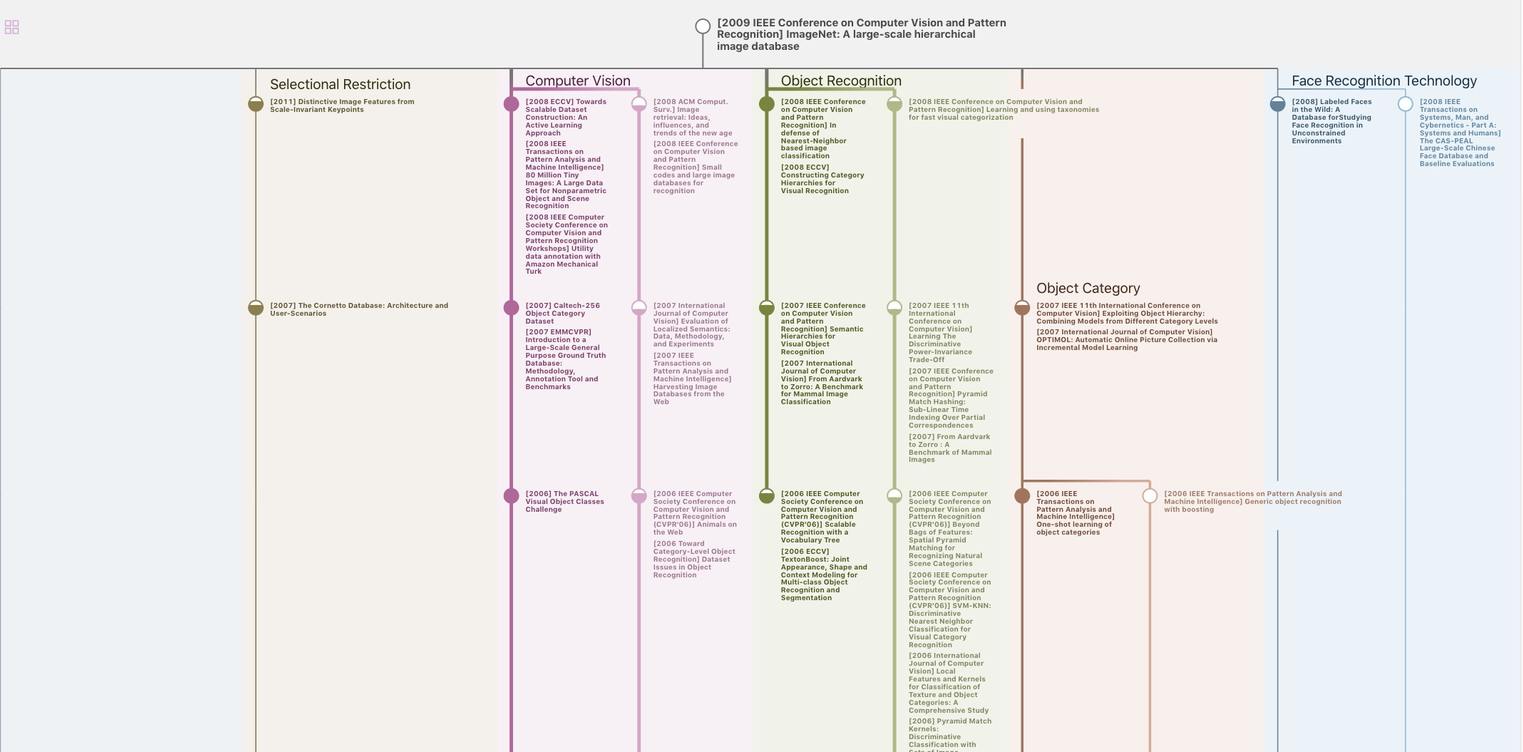
生成溯源树,研究论文发展脉络
Chat Paper
正在生成论文摘要