Super resolution reconstruction of background oriented schlieren based on the convolution neural network with the gradient loss function
Research Square (Research Square)(2023)
Abstract
Abstract In order to refine the displacement field of the background oriented schlieren method, a super-resolution method based on deep learning has been newly proposed in the study, and were compared with the bicubic interpolation. The gradient loss functions were firstly introduced into the hybrid downsampled skip-connection/multi-scale (DSC/MS) model to improve the reconstruction effect. The reconstruction effects of the new loss functions were compared with that of the traditional MSE loss function. The results showed that the Laplace operator with average pooling had better performance than the origin loss function in all the indexes including PSNR, MSE, MSE of the gradient and the max MSE. In these four indexes, MSE of the gradient and the max MSE performed especially better than the others, where the MSE of the gradient reduced from 3.90E-05 to 3.30E-05 and the max MSE reduced from 0.392 to 0.360.
MoreTranslated text
Key words
super resolution reconstruction,schlieren,neural network,gradient loss function
AI Read Science
Must-Reading Tree
Example
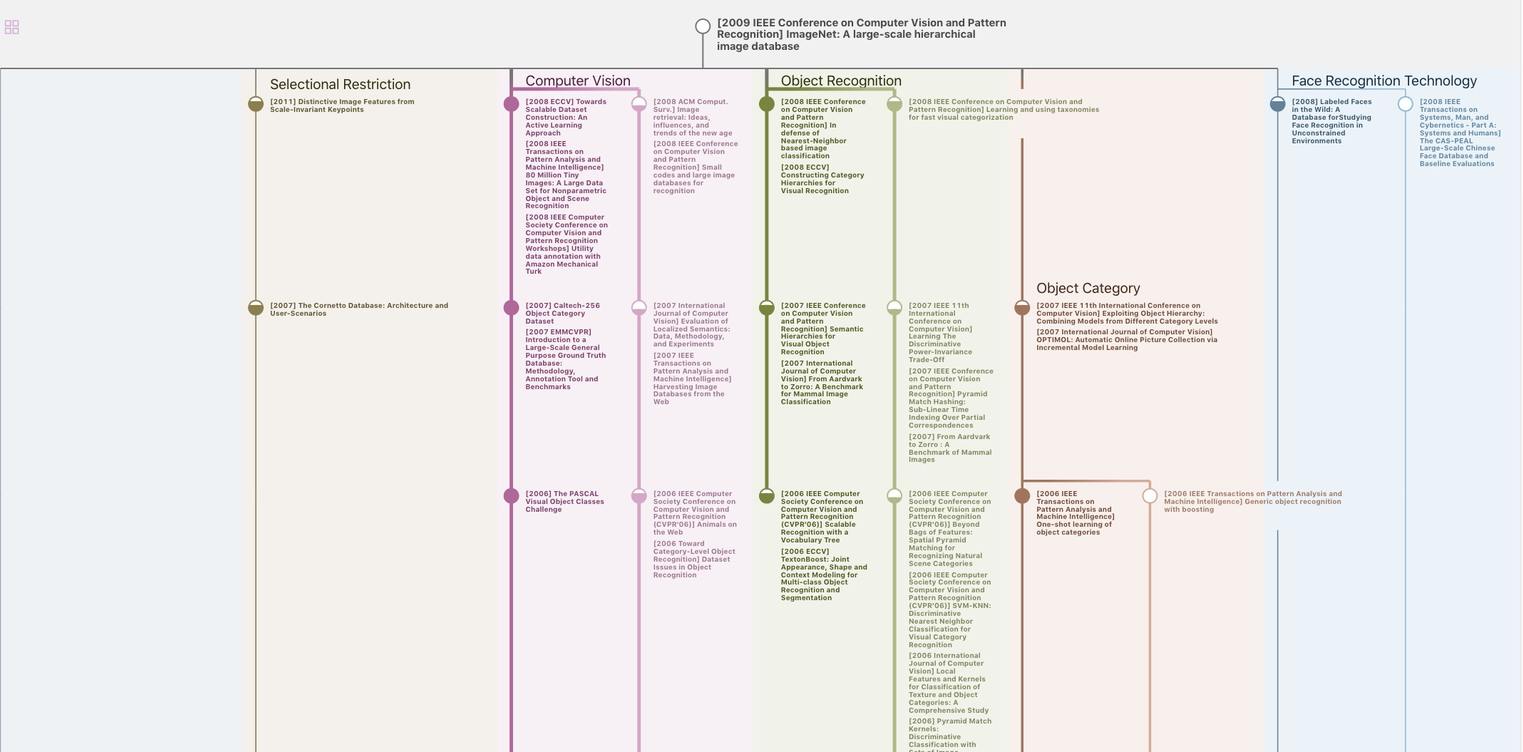
Generate MRT to find the research sequence of this paper
Chat Paper
Summary is being generated by the instructions you defined