A Comparative Study on Random Forests and Deep CNN-Based Learning Techniques with and without Random Forests as Classifier for COVID-19 Identification from Radiography X-Ray Images
Social Science Research Network(2023)
摘要
To reduce the outbreak of COVID-19 early identification and diagnosis of COVID-19 patients is the main concern for researchers and clinicians. And it is already discovered that medical imaging can be a less costly process for this purpose than PCR and antigen tests. As the COVID-19 is pneumonia disease it mostly affects the lung of the patients. That’s why the chest X-Ray images and breast CT scan images are mostly used for the purpose of COVID-19 identification, in which the chest X-Ray images are lower-cost ones. Medical imaging on these types of image datasets has shown promising results using machine learning, deep learning-based techniques. This comparative study includes both machine learning and deep learning-based CNN model for the COVID-19 identifications. We use Random Forests as a machine learning classifier and compare it with CNN-based models with and without the Random Forests classifier at the top CNN models. The CNN models include VGG16, ResNet50, and a lightweight designed Stacked CNN. The study shows that the ResNet50 model has the best performance in terms of all performance metrices for both with and without the Random Forests classifier, where the accuracy is 93%. Also stacked CNN provides 89% accuracy without Random Forets as a classifier with low computational complexity. VGG16 provides a comparable better performance of 90% accuracy with the Random Forest classifier, where the Random Forests classifier has the lowest performance, the 79% of accuracy.
更多查看译文
关键词
random forests,cnn-based,x-ray
AI 理解论文
溯源树
样例
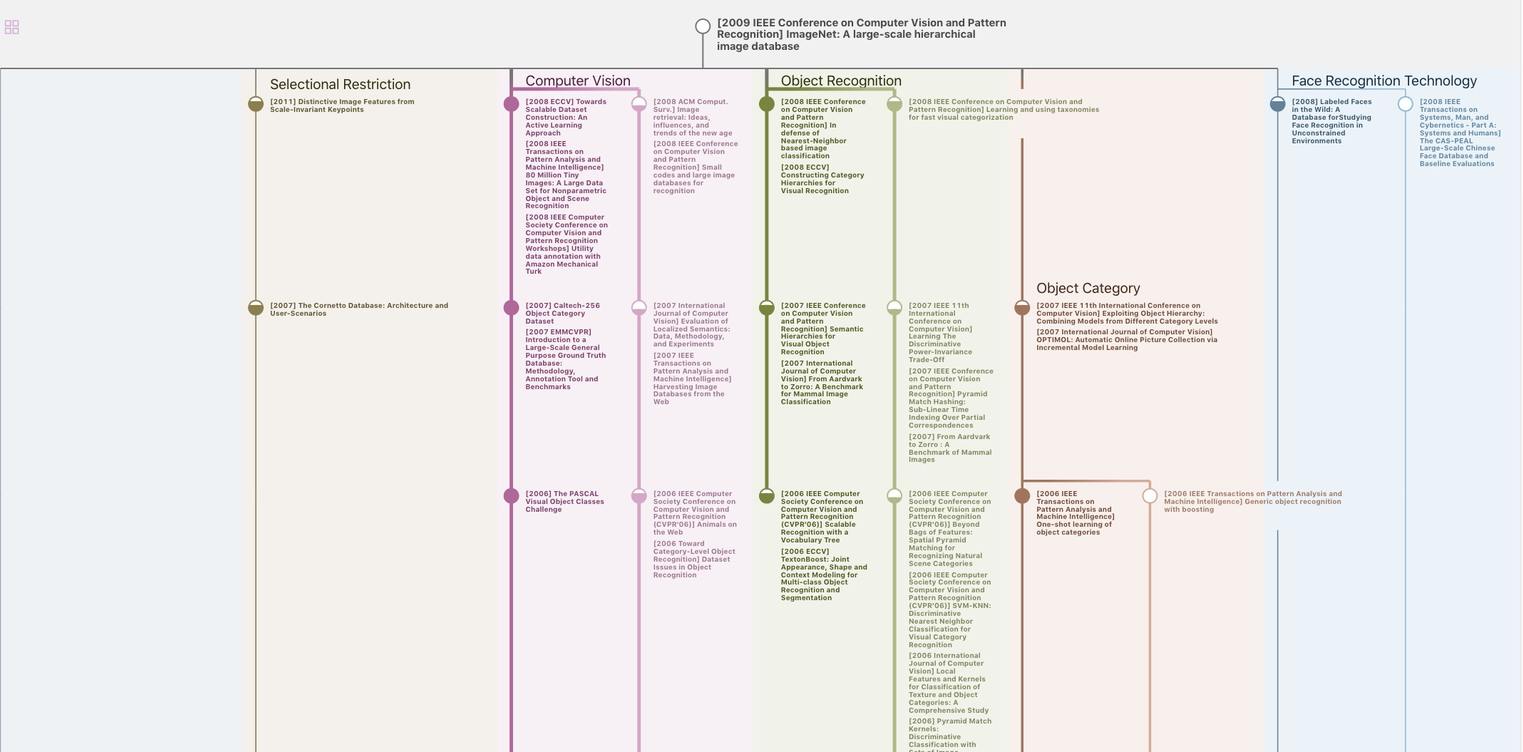
生成溯源树,研究论文发展脉络
Chat Paper
正在生成论文摘要