Combining two user-friendly machine learning tools increases species detection from acoustic recordings
CANADIAN JOURNAL OF ZOOLOGY(2024)
摘要
Passive acoustic monitoring usually generates large datasets that require machine learning algorithms to scan sound files, although the complexity of developing machine learning algorithms can be a barrier. We assessed the ability and speed of two user-friendly machine learning tools, Kaleidoscope Pro and BirdNET, for detecting the American toad (Anaxyrus americanus (Holbrook, 1836)) in sound recordings. We developed a two-step approach, combining both tools to maximize species detection while minimizing the time needed for output verification. When considered separately, Kaleidoscope Pro successfully detected the American toad in 85.9% of recordings in the validation dataset, while BirdNET detected the species in 58.4% of recordings. Combining the two tools in the two-step approach increased the detection rate to 93.3%. We applied the two-step approach to a large acoustic dataset (n = 6194 recordings). We started by scanning the dataset using Kaleidoscope Pro (species detected in 417 recordings), then we used BirdNET on the remaining recordings without confirmed presence. The two-step approach reduced the scanning time, the time needed for output verification, and added 37 additional species detections in 45 min. Our findings highlight that combining machine learning tools can improve species detectability while minimizing time and effort.
更多查看译文
关键词
American toad,BirdNET,convolutional neural network,Kaleidoscope Pro,Anaxyrus americanus (Holbrook,1836),passive acoustic monitoring
AI 理解论文
溯源树
样例
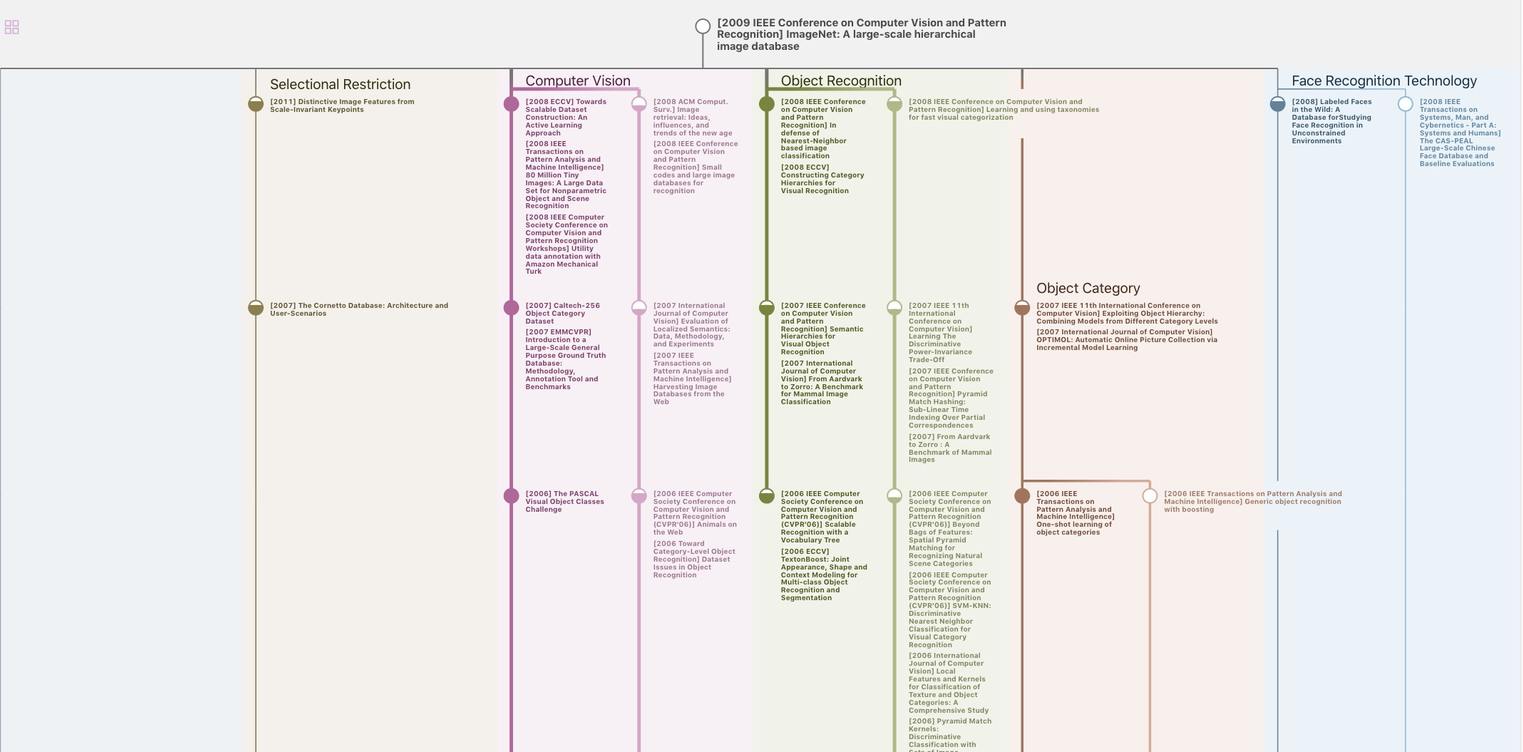
生成溯源树,研究论文发展脉络
Chat Paper
正在生成论文摘要