Can machine learning improve predictive performance of long-term outcomes for adults with breathlessness?
Epidemiology(2023)
摘要
Background: With advancements in technology, machine learning has shown merit in improving predictive performance for binary outcomes compared to logistic regression (LR). Aim: To compare the predictive performance of LR and machine learning algorithms (MLA) for outcomes of unplanned admission and all-cause mortality in patients coded with breathlessness in primary care records. Methods: Adults with a relevant diagnosis code (index date) subsequent to a breathlessness code recorded between 2007 and 2017 using UK CPRD were included. Three MLA (random forest [RF], gradient boosting machines [GBM], and elastic net) were compared to LR to predict unplanned admission and death within 2-years of diagnosis code. Data was split 70:30 (train:test) and predictive accuracy was compared by area under the curve (AUC [95% CI]). Results: 66909 adults were identified (45% male; mean [SD] age 53 [16] y). Compared to LR for unplanned admission (0.66 [0.65-0.66]), MLA improved prediction: elastic net (0.92 [0.91-0.92]), GBM (0.67 [0.67-0.68]); but, RF worsened prediction (0.62 [0.62-0.63]). In contrast, LR outperformed all MLA when predicting all-cause mortality (0.89 [0.88-0.90]) correctly predicting 446/568 cases (sensitivity 83%). Conclusion: Our results suggest ML methods may not always improve predictive performance of models for health research and LR can be sufficient.
更多查看译文
关键词
breathlessness,predictive performance,machine learning,long-term
AI 理解论文
溯源树
样例
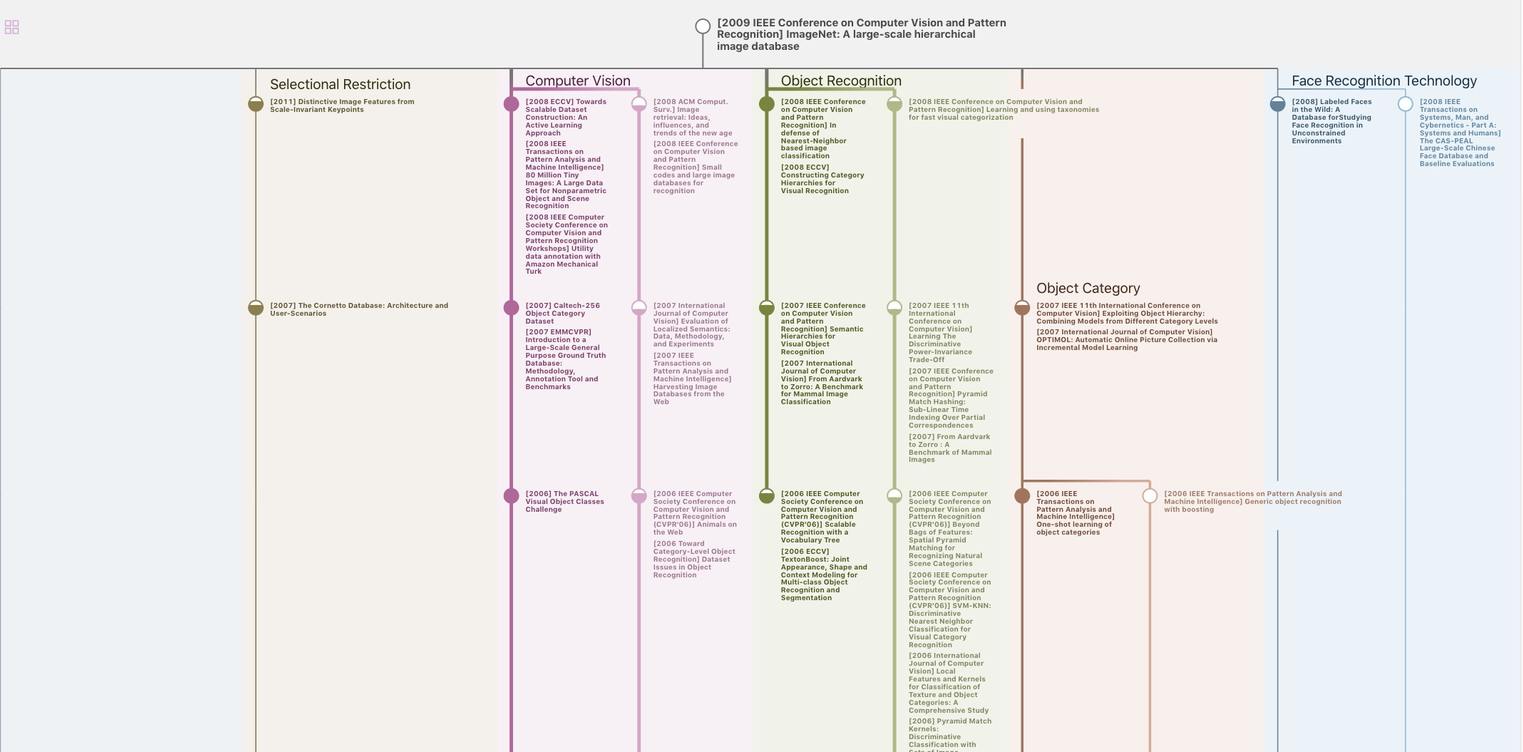
生成溯源树,研究论文发展脉络
Chat Paper
正在生成论文摘要