Mutual information-based feature selection for inverse mapping parameter updating of dynamical systems
Research Square (Research Square)(2023)
摘要
Abstract A digital twin should be and remain an accurate model representation of a physical system throughout its operational life. To this end, we aim to update (physically interpretable) parameters of such a model in an online fashion. Hereto, we employ the Inverse Mapping Parameter Updating (IMPU) method which uses an Artificial Neural Network (ANN) to map features, extracted from measurement data, to parameter estimates. This is achieved by training the ANN offline on simulated data, i.e., pairs of known parameter value sets and sets of features extracted from corresponding simulations. Since a plethora of features (and feature types) can be extracted from simulated time domain data, Feature Selection (FS) strategies are investigated. These strategies employ the mutual information between features and parameters to select an informative subset of features. Hereby, accuracy of the parameters estimated by the ANN is increased and, at the same time, ANN training and inference computation times are decreased.Additionally, Bayesian search-based hyperparameter tuning is employed to enhance performance of the ANNs and optimize the ANN structure for various FS strategies.Finally, the IMPU method is applied to a high-tech industrial use case of a semi-conductor machine, for which measurements are performed in closed-loop on the controlled physical system. This system is modeled as a nonlinear multibody model in the Simscape multibody environment. It is shown that the model updated using the IMPU method simulates the measured system more accurately than a reference model of which the parameter values have been determined manually.
更多查看译文
关键词
inverse mapping parameter updating,feature selection,information-based
AI 理解论文
溯源树
样例
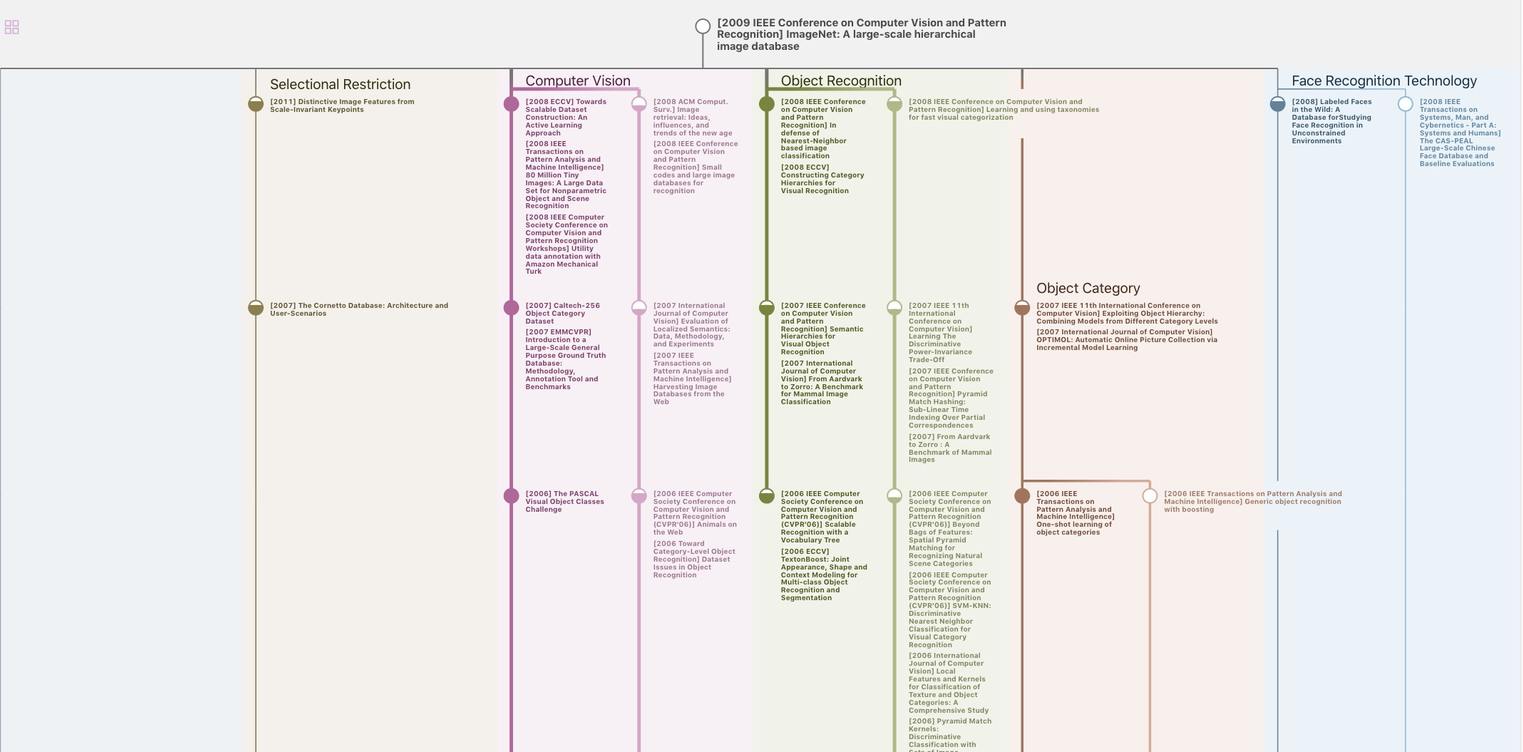
生成溯源树,研究论文发展脉络
Chat Paper
正在生成论文摘要